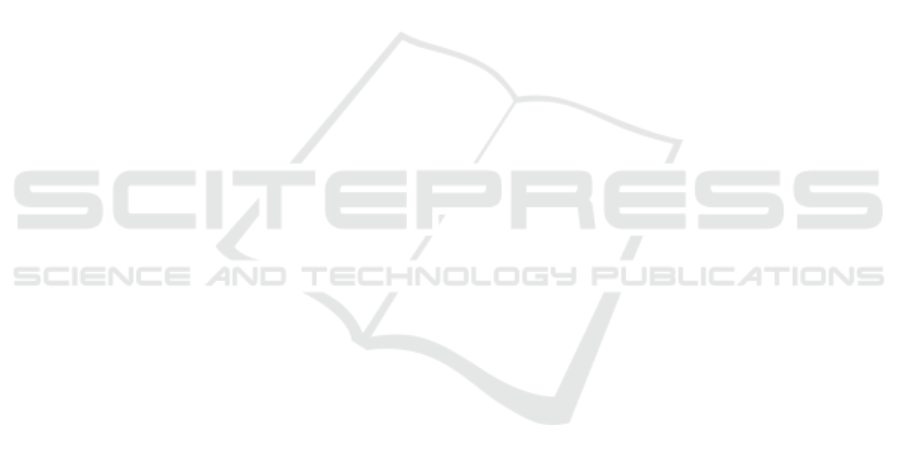
Mitsubishi i–MiEV minicar. Transportation Research
Part D: Transport and Environment, 58(2018):225–
238.
Cauwer, C. D., Verbeke, W., Coosemans, T., Faid, S., and
Mierlo, J. V. (2017). A data-driven method for energy
consumption prediction and energy-efficient routing
of electric vehicles in real-world conditions. Energies,
10(5):608.
Cordts, M., Omran, M., Ramos, S., Rehfeld, T., Enzweiler,
M., Benenson, R., Franke, U., Roth, S., and Schiele,
B. (2016). The Cityscapes Dataset for Semantic Urban
Scene Understanding. Proc. of the IEEE Conference
on Computer Vision and Pattern Recognition (CVPR).
Enthaler, A. and Gauterin, F. (2016). Method for reduc-
ing uncertainties of predictive range estimation algo-
rithms in electric vehicles. 2015 IEEE 82nd Vehicular
Technology Conference, VTC Fall 2015 - Proceedings.
Ericsson, E. (2001). Independent driving pattern factors and
their influence on fuel-use and exhaust emission fac-
tors. Transportation Research Part D: Transport and
Environment, 6(5):325–345.
Fetene, G. M., Kaplan, S., Mabit, S. L., Jensen, A. F., and
Prato, C. G. (2017). Harnessing big data for estimat-
ing the energy consumption and driving range of elec-
tric vehicles. Transportation Research Part D: Trans-
port and Environment, 54:1–11.
Fukushima, A., Yano, T., Imahara, S., Aisu, H.,
Shimokawa, Y., and Shibata, Y. (2018). Prediction of
energy consumption for new electric vehicle models
by machine learning. IET Intelligent Transport Sys-
tems, 12(9):1174–1180.
Gebhardt, K., Schau, V., and Rossak, W. R. (2015). Ap-
plying stochastic methods for range prediction in e-
mobility. In 2015 15th International Conference on
Innovations for Community Services (I4CS). IEEE.
Genikomsakis, K. N. and Mitrentsis, G. (2017). A computa-
tionally efficient simulation model for estimating en-
ergy consumption of electric vehicles in the context of
route planning applications. Transportation Research
Part D: Transport and Environment, 50:98–118.
Grubwinkler, S., Hirschvogel, M., and Lienkamp, M.
(2014). Driver- and situation-specific impact factors
for the energy prediction of EVs based on crowd-
sourced speed profiles. IEEE Intelligent Vehicles Sym-
posium, Proceedings, (Iv):1069–1076.
Helmer, T., Wang, L., Kompass, K., and Kates, R. (2015).
Safety Performance Assessment of Assisted and Au-
tomated Driving by Virtual Experiments: Stochastic
Microscopic Traffic Simulation as Knowledge Syn-
thesis. In Intelligent Transportation Systems (ITSC),
2015 IEEE 18th International Conference on, volume
2015-Octob, pages 2019–2023. IEEE.
Horrein, L., Bouscayrol, A., Lhomme, W., and D
´
epature,
C. (2017). Impact of heating system on the range of
an electric vehicle. IEEE Transactions on Vehicular
Technology, 66(6):4668–4677.
Hu, K., Wu, J., and Schwanen, T. (2017). Differences
in energy consumption in electric vehicles: An ex-
ploratory real-world study in Beijing. Journal of Ad-
vanced Transportation, 2017:1–17.
Langner, J., Bach, J., Otten, S., Sax, E., Esselborn, C., Holz,
M., and Eckert, M. (2017). Framework for using real
driving data in automotive feature development and
validation.
Lewis, D. D. (1992). Representation and Learning in In-
formation Retrieval. PhD thesis, Amherst, MA, USA.
UMI Order No. GAX92-19460.
Mazzega, J., K
¨
oster, F., Lemmer, K., and Form, T. (2016).
Testing of Highly Automated Driving Functions. ATZ
worldwide, 118(10):44–48.
Mitchell, H. B. (2012). Data Fusion: Concepts and Ideas.
Springer Berlin Heidelberg, Berlin, Heidelberg, 2nd
ed. 2012 edition.
Ondr
´
u
ˇ
ska, P. and Posner, I. (2014). The route not taken:
Driver-centric estimation of electric vehicle range.
In Proceedings of the Twenty-Fourth International
Conferenc on International Conference on Automated
Planning and Scheduling, ICAPS’14, pages 413–420.
AAAI Press.
Qi, X., Wu, G., Boriboonsomsin, K., and Barth, M. J.
(2018). Data-driven decomposition analysis and esti-
mation of link-level electric vehicle energy consump-
tion under real-world traffic conditions. Transporta-
tion Research Part D: Transport and Environment,
64:36–52.
Rolim, C. C., Gonc¸alves, G. N., Farias, T. L., and Ro-
drigues,
´
O. (2012). Impacts of Electric Vehicle Adop-
tion on Driver Behavior and Environmental Perfor-
mance. Procedia - Social and Behavioral Sciences,
54:706–715.
Sautermeister, S., Ott, F., Vaillant, M., and Gauterin, F.
(2017). Reducing Range Estimation Uncertainty with
a Hybrid Powertrain Model and Online Parameter Es-
timation. 2017 IEEE 20th International Conference
on Intelligent Transportation Systems (ITSC), pages
910–915.
Sax, E. (2008). Automatisiertes Testen Eingebetteter
Systeme in der Automobilindustrie. Hanser, Carl,
M
¨
unchen.
Tannahill, V. R., Muttaqi, K. M., and Sutanto, D. (2016).
Driver alerting system using range estimation of elec-
tric vehicles in real time under dynamically varying
environmental conditions. IET Electrical Systems in
Transportation, 6(2):107–116.
Thibault, L., Nunzio, G. D., and Sciarretta, A. (2018). A
unified approach for electric vehicles range maximiza-
tion via eco-routing, eco-driving, and energy con-
sumption prediction. IEEE Transactions on Intelligent
Vehicles, pages 1–1.
Tseng, C.-M. and Chau, C.-K. (2017). Personalized pre-
diction of vehicle energy consumption based on par-
ticipatory sensing. IEEE Transactions on Intelligent
Transportation Systems, 18(11):3103–3113.
Wager, G., Whale, J., and Braunl, T. (2016). Driving elec-
tric vehicles at highway speeds: The effect of higher
driving speeds on energy consumption and driving
range for electric vehicles in australia. Renewable and
Sustainable Energy Reviews, 63:158–165.
Wang, J., Besselink, I., and Nijmeijer, H. (2015). Electric
vehicle energy consumption modelling and prediction
VEHITS 2019 - 5th International Conference on Vehicle Technology and Intelligent Transport Systems
442