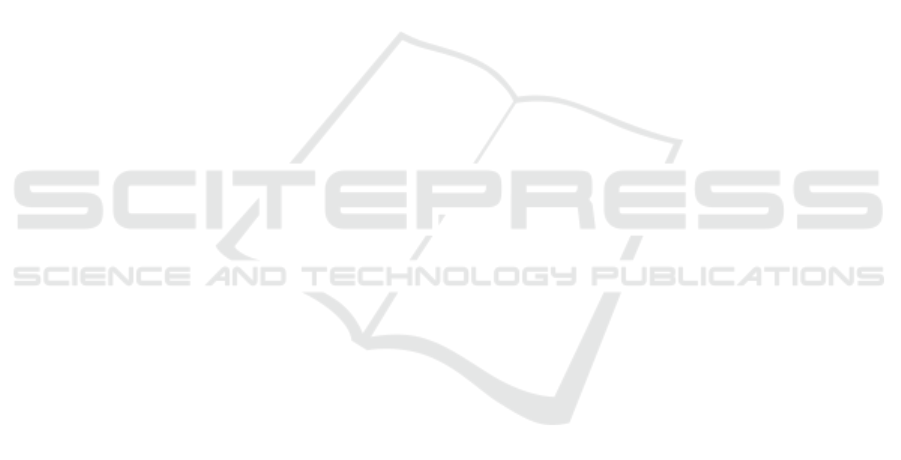
variable-width CL-band (cf. figure 1). It is bounded
by the upper limit of the PI of the day-ahead predic-
tion of uncond. loads used as day-ahead schedule.
REFERENCES
Arora, S. and Taylor, J. W. (2016). Forecasting electricity
smart meter data using conditional kernel density es-
timation. Omega, 59:47–59.
Atzeni, I. (2014). Distributed demand-side optimization in
the smart grid. PhD thesis.
Bagemihl, J., Boesner, F., Riesinger, J., K
¨
unzli, M., Wilke,
G., Binder, G., Wache, H., Laager, D., Breit, J.,
Wurzinger, M., Zapata, J., Ulli-Beer, S., Layec, V.,
Stadler, T., and Stabauer, F. (2018). A market-based
smart grid approach to increasing power grid capacity
without physical grid expansion. Computer Science -
Research and Development, 33(1-2):177–183.
Doostizadeh, M. and Ghasemi, H. (2012). A day-ahead
electricity pricing model based on smart metering and
demand-side management. Energy, 46:221–230.
Ghofrani, M., Hassanzadeh, M., Etezadi-Amoli, M., and
Fadali, M. S. (2011). Smart meter based short-term
load forecasting for residential customers. In 2011
North American Power Symposium, pages 1–5.
Hunziker, C., Schulz, N., and Wache, H. (2018). Shap-
ing aggregated load profiles based on optimized local
scheduling of home appliances. Computer Science -
Research and Development, 33:61–70.
Kong, W., Dong, Z. Y., Jia, Y., Hill, D. J., Xu, Y., and
Zhang, Y. (2017). Short-term residential load fore-
casting based on lstm recurrent neural network. IEEE
Transactions on Smart Grid.
Marino, D. L., Amarasinghe, K., and Manic, M. (2016).
Building energy load forecasting using deep neural
networks. In Industrial Electronics Society, IECON
2016-42nd Annual Conference of the IEEE, pages
7046–7051. IEEE.
Mirowski, P., Chen, S., Ho, T. K., and Yu, C.-N. (2014). De-
mand forecasting in smart grids. Bell Labs technical
journal, 18(4):135–158.
Mocanu, E., Nguyen, P. H., Gibescu, M., and Kling, W. L.
(2016). Deep learning for estimating building energy
consumption. Sustainable Energy, Grids and Net-
works, 6:91–99.
Mohsenian-Rad, A.-H. and Leon-Garcia, A. (2010). Op-
timal residential load control with price prediction
in real-time electricity pricing environments. IEEE
Transactions on Smart Grid, 1:120–133.
Ryu, S., Noh, J., and Kim, H. (2016). Deep neural network
based demand side short term load forecasting. Ener-
gies, 10(1):3.
Shaikh, P. H., Bin Mohd Nor, N., Nallagownden, P., Elam-
vazuthi, I., and Ibrahim, T. (2014). A review on op-
timized control systems for building energy and com-
fort management of smart sustainable buildings. Re-
newable and Sustainable Energy Reviews, 34:409–
429.
Shariatzadeh, F., Mandal, P., and Srivastava, A. K. (2015).
Demand response for sustainable energy systems: A
review, application and implementation strategy. Re-
newable and Sustainable Energy Reviews, 45:343–
350.
Shi, H., Xu, M., and Li, R. (2018). Deep learning for
household load forecasting—a novel pooling deep
rnn. IEEE Transactions on Smart Grid, 9(5):5271–
5280.
Taylor, J. W. (2010). Triple seasonal methods for short-term
electricity demand forecasting. European Journal of
Operational Research, 204(1):139–152.
Taylor, J. W., De Menezes, L. M., and McSharry, P. E.
(2006). A comparison of univariate methods for fore-
casting electricity demand up to a day ahead. Interna-
tional Journal of Forecasting, 22(1):1–16.
Taylor, J. W., McSharry, P. E., et al. (2007). Short-term
load forecasting methods: An evaluation based on eu-
ropean data. IEEE Transactions on Power Systems,
22(4):2213–2219.
Thirugnanam, K., Kerk, S. K., Yuen, C., Liu, N., and Zhang,
M. (2018). Energy management for renewable micro-
grid in reducing diesel generators usage with multiple
types of battery. IEEE TRANSACTIONS ON INDUS-
TRIAL ELECTRONICS, 65:6772–6786.
Zhao, Z., Lee, W., Shin, Y., and Song, K. (2013). An opti-
mal power scheduling method for demand response in
home energy management system. IEEE Transactions
on Smart Grid, 4:1391–1400.
Zheng, J., Xu, C., Zhang, Z., and Li, X. (2017). Electric
load forecasting in smart grids using long-short-term-
memory based recurrent neural network. In Informa-
tion Sciences and Systems (CISS), 2017 51st Annual
Conference on, pages 1–6. IEEE.
Zufferey, T., Ulbig, A., Koch, S., and Hug, G. (2016). Fore-
casting of smart meter time series based on neural net-
works. In International Workshop on Data Analyt-
ics for Renewable Energy Integration, pages 10–21.
Springer.
SMARTGREENS 2019 - 8th International Conference on Smart Cities and Green ICT Systems
130