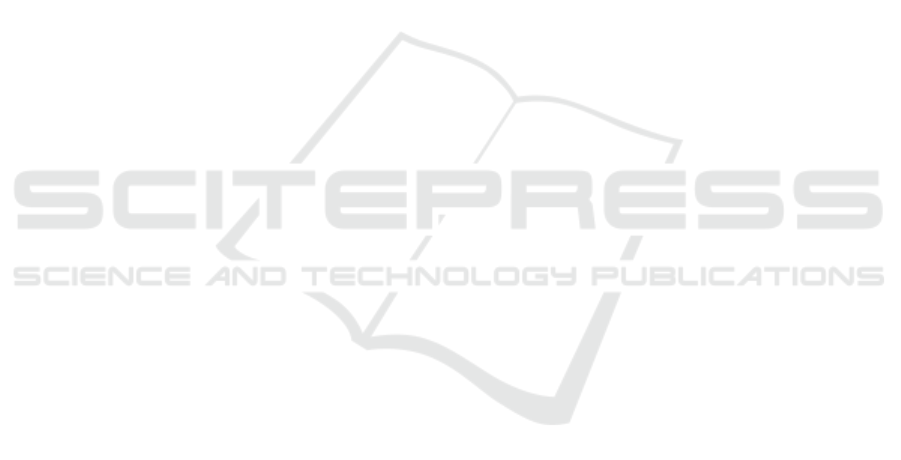
application to network anomaly detection by
hierarchical self-organising maps, Knowl. Based Syst.
71, 322–338.
Koc L., Mazzuchi T. A., Sarkani S., 2012. A network
intrusion detection system based on a hidden naive
bayes multiclass classifier, Expert Syst. Appl. 39 (18),
13492–13500.
Louvieris P., Clewley N., Liu X., 2013. Effects-based
feature identification for network intrusion detection,
Neurocomputing 121 (18), 265–273.
Mukherjee S., Sharma N., 2012. Intrusion detection using
naive bayes classifier with feature reduction, Procedia
Technol. 4 (11), 119–128.
Liao Y., Vemuri V.R., 2002. Use of k-nearest neighbor
classifier for intrusion detection, Comput. Security 21
(5), 439–448.
Li Y., Xia J., Zhang S., Yan J., Ai X., Dai K., 2012. An
efficient intrusion detection system based on support
vector machines and gradually feature removal method,
Expert Syst. Appl. 39 (1), 424–430.
Fu S., Liu J. G., and Pannu H., 2012. A Hybrid anomaly
detection framework in cloud computing using one-
class and two-class support vector machines, In 8 th
International Conference, Advanced Data Mining and
Applications, pp. 726-738.
Manzoor Akashdeep I. and Kumar N., 2017. A feature
reduced intrusion detection system using ANN
classifier, Expert Systems with Applications, 88, 249-
257.
Farnaaz N. and Jabbar M. A., 2016. Random Forest
Modeling for Network Intrusion Detection System,
Procedia Computer Science 89, 213-217.
Sangkatsanee P., Wattanapongsakorn N., and
Charnsripinyo C., 2011. Practical real time intrusion
detection using machine learning approach. Journal of
Computer Communication, 34, 2227–2235.
Liu Q., Sui S., and Xiao J., 2014. A mutual information
based hybrid feature selection method using feature
clustering. In IEEE 38th annual international
conference on computers, software and application, pp.
27–32.
Al-Jarrah O. Y., Siddiqui A., Elsalamouny M., Yoo P. D.,
Muhaidat S., and Kim K., 2014. Machine learning
based feature selection techniques for large scale
intrusion detection. In 2014 IEEE 34th International
Conference on Distributed Computing Systems
Workshops, pp. 177–181.
Kuang F., Xu W., Zhang S., 2014. A novel hybrid kpca and
svm with ga model for intrusion detection, Appl. Soft.
Comput. 18 (4), 178–184.
Lin W.-C., Ke S.-W., Tsai C.-F., 2015. Cann: an intrusion
detection system based on combining cluster centers
and nearest neighbors, Knowl. Based Syst. 78 (1), 13–
21.
Horng S. J., Su M. Y., Chen Y.H., Kao T. W., Chen R.J.,
Lai J. L., Perkasa C.D., 2011. A novel intrusion
detection system based on hierarchical clustering and
support vector machines, Expert Syst. Appl. 38 (1),
306–313.
Wang H. W., Gu J., and Wang S. S., 2017. An effective
intrusion detection framework based on SVM with
feature augmentation, Knowledge-Based System, 136,
130-139.
Zhou Z. H., 2016. Machine Learning, Tsinghua University
Press. China, 1
nd
edition.
Tavallaee M., Bagheri E., Lu W., Ghorbani A. A., 2009. A
detailed analysis of the kdd cup 99 data set, In
Proceedings of the Second IEEE Symposium on
Computational Intelligence for Security and Defense
Applications.
Bhattacharya S., Selvakumar S., 2015. LAWRA: a layered
wrapper feature selection approach for network attack
detection, Security Commun Netw 8 (18), 3459–3468.
De La Hoz E., De La Hoz E., Ortiz A., Ortega J., Prieto B.,
2015. PCA filtering and probabilistic som for network
intrusion detection, Neurocomputing 164, 71–81.
Kim G., Lee S., Kim S., 2014. A novel hybrid intrusion
detection method integrating anomaly detection with
misuse detection, Expert Syst. Appl. 41 (4), 1690–
1700.
ICEIS 2019 - 21st International Conference on Enterprise Information Systems
228