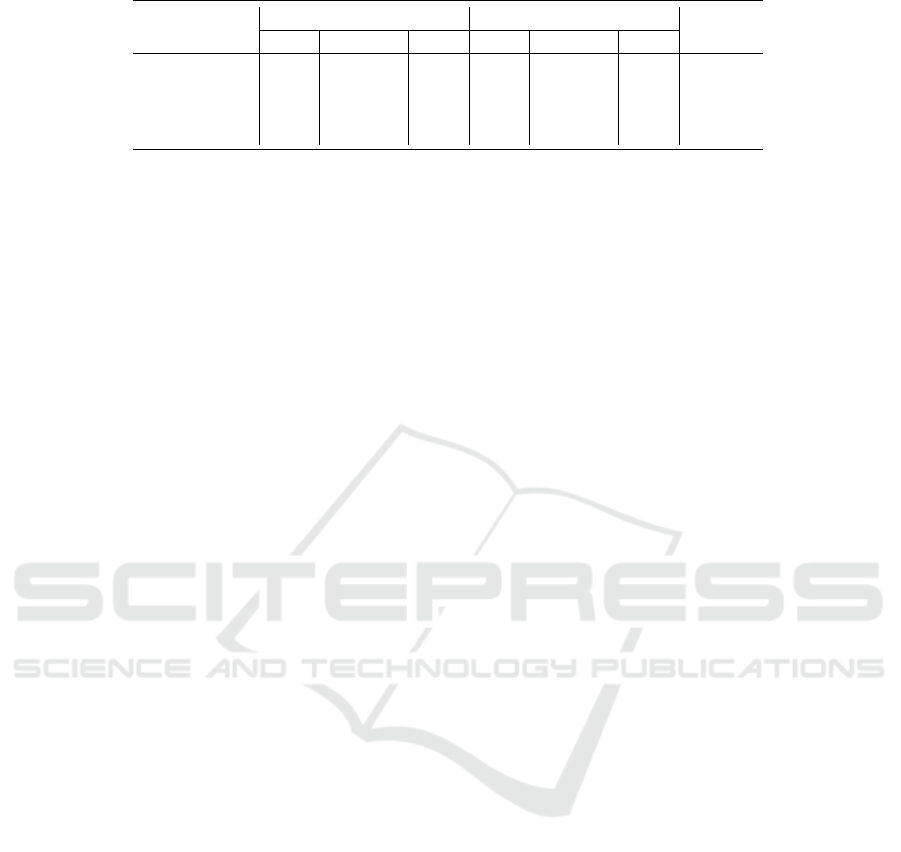
Table 6: Result of Quality Assessment based on Consistency and Conciseness Dimension.
Dataset
Consistency Conciseness
Quality
low medium high low medium high
Peel 58% 41% 0% 0% 11% 88% 41%
DBLP (L3S) 3% 96% 0% 0% 2% 97% 49%
DBpedia 0% 37% 62% 0% 3% 96% 59%
EIONET 0% 34% 65% 24% 75% 0% 60%
Table 5, the quality is high when greater than 50% and
the dataset has high quality since it meets this rule.
6 CONCLUSION
In this work, we proposed the use of fuzzy logic to
model the domain of data quality. We described a
fuzzy ontology to represent data quality. Additionally,
we described and analyzed an approach for evaluating
Linked Data quality based on fuzzy logic. In the pro-
posed approach, quality measurement is inferred by
fuzzy inference systems based on user-defined fuzzy
rules. Results of experiments on real datasets were
reported and discussed.
We emphasize that the proposed approach present
real benefits in the process of providing linked data
mashups with required level of quality. As future
work, we shall investigate the use of fuzzy logic in
computing mashup data quality.
ACKNOWLEDGEMENTS
This work was partly funded by CNPq under grants
303332/2013-1 and 442338/2014-7, by FAPERJ un-
der grant E-26/201.337/2014, and by FCT - Fundac¸
˜
ao
para a Ci
ˆ
encia e a Tecnologia, under the grant SFRH/-
BPD/76024/2011.
REFERENCES
Bizer, C. and Cyganiak, R. (2009). Quality-driven informa-
tion filtering using the WIQA policy framework. Web
Semant., 7(1):1–10.
Debattista, J., Auer, S., and Lange, C. (2016a). Luzzu—a
methodology and framework for linked data quality
assessment. Journal of Data and Information Quality
(JDIQ), 8(1):4.
Debattista, J., Dekkers, M., Gu
´
eret, C., Lee, D., Mihinduku-
lasooriya, N., and Zaveri, A. (2016b). Data on the web
best practices: Data quality vocabulary.
Debattista, J., Lange, C., and Auer, S. (2014a). daq, an on-
tology for dataset quality information. In Proc. Work-
shop on Linked Data on the Web co-located with the
23rd Int. World Wide Web Conference (WWW 2014).
Debattista, J., Lange, C., Auer, S., and Cortis, D. Evaluat-
ing the quality of the lod cloud: An empirical investi-
gation.
Debattista, J., Londo
˜
no, S., Lange, C., and Auer, S. (2014b).
LUZZU - A framework for linked data quality assess-
ment. CoRR, abs/1412.3750.
F
¨
urber, C. and Hepp, M. (2011). Towards a vocabulary
for data quality management in semantic web archi-
tectures. In Proc. 1st Int. Workshop on Linked Web
Data Management, LWDM ’11, NY, USA. ACM.
Gu
´
eret, C., Groth, P., Stadler, C., and Lehmann, J.
(2012). Assessing linked data mappings using net-
work measures. In Proc. 9th International Confer-
ence on The Semantic Web: Research and Applica-
tions, ESWC’12, pages 87–102. Berlin, Heidelberg.
Ivezi
´
c, D., Tanasijevi
´
c, M., and Ignjatovi
´
c, D. (2008).
Fuzzy approach to dependability performance evalu-
ation. Quality and Reliability Engineering Interna-
tional, 24(7):779–792.
Knap, T., Michelfeit, J., Daniel, J., Jerman, P., Rych-
novsk
`
y, D., Soukup, T., and Ne
ˇ
cask
`
y, M. (2012). Od-
cleanstore: a framework for managing and providing
integrated linked data on the web. In Int. Conf. Web
Information Systems Engineering, pages 815–816.
Mendes, P. N., M
¨
uhleisen, H., and Bizer, C. (2012). Sieve:
Linked data quality assessment and fusion. In Proc.
2012 Joint EDBT/ICDT Workshops, EDBT-ICDT ’12,
pages 116–123, NY, USA. ACM.
Ross, T. (2009). Fuzzy logic with engineering applications.
John Wiley & Sons, Inc.
Schultz, A., Matteini, A., Isele, R., Bizer, C., and Becker, C.
(2011). LDIF - linked data integration framework. In
Proc. Second Int. Conf. on Consuming Linked Data,
COLD’11, Aachen, Germany. CEUR-WS.org.
Wang, R. Y. (2005). Information Quality (Advances in Man-
agement Information Systems). M. E. Sharpe, Inc.,
Armonk, NY, USA.
Wang, R. Y. and Strong, D. M. (1996). Beyond accuracy:
What data quality means to data consumers. J. Man-
age. Inf. Syst., 12(4):5–33.
Yaguinuma, C., Santos, M. T. P., Camargo, H. A., Nico-
letti, M. C., and Nogueira, T. M. (2012). Fuzz-onto:
A meta-ontology for representing fuzzy elements and
supporting fuzzy classification rules. In Proc. 12th Int.
Conf. on Intelligent Systems Design and Applications.
Zadeh, L. (1965). Fuzzy sets. Information and Control,
8(3):338 – 353.
Zaveri, A., Rula, A., Maurino, A., Pietrobon, R., Lehmann,
J., and Auer, S. (2016). Quality assessment for linked
data: A survey. Semantic Web, 7(1):63–93.
ICEIS 2019 - 21st International Conference on Enterprise Information Systems
406