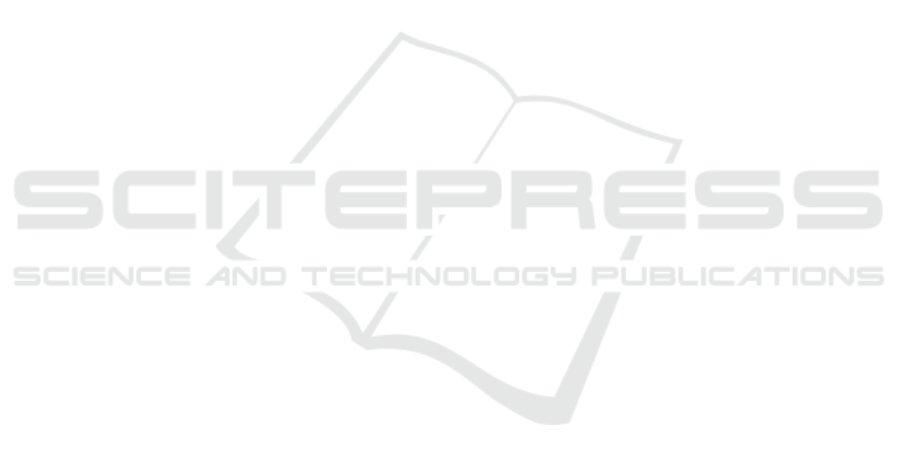
for Environment and Sustainability, 2015. The reference
scenario in the LUISA platform: updated configuration
2014. Publications Office, Luxembourg.
Bayerisches Staatsministerium für Umwelt und Gesundheit,
Bayerisches Staatsministerium für Wirtschaft,
Infrastruktur, Verkehr und Technologie, Oberste
Baubehörde im Bayerischen Staatsministerium des
Innern, 2011. Leitfaden Energienutzungsplan. URL
http://www.stmi.bayern.de/imperia/md/content/stmi/bau
en/rechtundtechnikundbauplanung/_staedtebau/veroeffe
ntlichungen/oeko/leitfaden_enp.pdf (accessed 4.18.12).
Cajot, S., Peter, M., Bahu, J.-M., Guignet, F., Koch, A.,
Maréchal, F., 2017. Obstacles in energy planning at the
urban scale. Sustain. Cities Soc. 30, 223–236.
https://doi.org/10.1016/j.scs.2017.02.003
DeCarolis, J., Daly, H., Dodds, P., Keppo, I., Li, F.,
McDowall, W., Pye, S., Strachan, N., Trutnevyte, E.,
Usher, W., Winning, M., Yeh, S., Zeyringer, M., 2017.
Formalizing best practice for energy system optimization
modelling. Appl. Energy 194, 184–198.
https://doi.org/10.1016/j.apenergy.2017.03.001
Deutsche Bundesnetzagentur, 2018. Kraftwerksliste - Stand
19.11.2018 URL
https://www.bundesnetzagentur.de/DE/Sachgebiete/Ele
ktrizitaetundGas/Unternehmen_Institutionen/Versorgun
gssicherheit/Erzeugungskapazitaeten/Kraftwerksliste/kr
aftwerksliste-node.html (accessed 1.2.19).
Deutsche Gesellschaft für Sonnenenergie e.V. (DGS), 2016.
EnergyMap - Bundesrepublik Deutschland. URL
http://www.energymap.info/energieregionen/DE/105.ht
ml (accessed 1.2.19).
Dong, J., Liu, C., Lin, Z., 2014. Charging infrastructure
planning for promoting battery electric vehicles: An
activity-based approach using multiday travel data.
Transp. Res. Part C Emerg. Technol. 38, 44–55.
https://doi.org/10.1016/j.trc.2013.11.001
EAFO, 2018. Home | EAFO. URL https://www.eafo.eu/
(accessed 1.2.19).
Energie-Atlas Bayern – Mischpult „Energiemix Bayern vor
Ort“, Informationen zu den Rahmendaten:
Stromverbrauch, 2018. 4.
Foley, A., Tyther, B., Calnan, P., Ó Gallachóir, B., 2013.
Impacts of Electric Vehicle charging under electricity
market operations. Appl. Energy, Sustainable
Development of Energy, Water and Environment
Systems 101, 93–102.
https://doi.org/10.1016/j.apenergy.2012.06.052
Fraunhofer, 2019. Energy Charts. URL https://www.energy-
charts.de/ (accessed 1.2.19).
Garcia-Valle, R., Lopes, J.A.P., 2012. Electric Vehicle
Integration into Modern Power Networks. Springer
Science & Business Media.
Genikomsakis, K.N., Bocquier, B., Lopez, S., Ioakimidis,
C.S., 2016. Utilizing plug-in electric vehicles for peak
shaving and valley filling in non-residential buildings
with solar photovoltaic systems, in: 2016 5th
International Conference on Smart Cities and Green ICT
Systems (SMARTGREENS). Presented at the 2016 5th
International Conference on Smart Cities and Green ICT
Systems (SMARTGREENS), pp. 1–10.
Hans-Ertel-Zentrum für Wetterforschung, 2018. COSMO
Regional Reanalysis - COSMO-REA6 URL
http://reanalysis.meteo.uni-bonn.de/?COSMO-REA6
(accessed 1.24.18).
Janečka, K., Souček, P., 2017. A Country Profile of the
Czech Republic Based on an LADM for the
Development of a 3D Cadastre. ISPRS Int. J. Geo-Inf. 6,
143. https://doi.org/10.3390/ijgi6050143
Mu, Y., Wu, J., Jenkins, N., Jia, H., Wang, C., 2014. A
Spatial–Temporal model for grid impact analysis of plug-
in electric vehicles. Appl. Energy 114, 456–465.
https://doi.org/10.1016/j.apenergy.2013.10.006
Nationale Plattform Elektromobilität, 2015. Charging
Infrastructure for Electric Vehicles in Germany:
Working Group 3 – Charging Infrastructure and Power
Grid Integration.
Pagany, R., Ramirez Camargo, L., Dorner, W., 2018. A
review of spatial localization methodologies for the
electric vehicle charging infrastructure. Int. J. Sustain.
Transp. 1–17.
https://doi.org/10.1080/15568318.2018.1481243
Passey, R., Spooner, T., MacGill, I., Watt, M., & Syngellakis,
K. (2011). The potential impacts of grid-connected
distributed generation and how to address them: A
review of technical and non-technical factors. Energy
Policy, 39(10), 6280–6290.
http://dx.doi.org/10.1016/j.enpol.2011.07.027
Ramirez Camargo, L., Gruber, K., Nitsch, F., 2018.
Assessing variables of regional reanalysis data sets
relevant for modelling small-scale renewable energy
systems. Renew. Energy.
https://doi.org/10.1016/j.renene.2018.09.015
Ramirez Camargo, L., Stoeglehner, G., 2018. Spatiotemporal
modelling for integrated spatial and energy planning.
Energy Sustain. Soc. 8. https://doi.org/10.1186/s13705-
018-0174-z
Rivera, J., Leimhofer, J., Jacobsen, H.-A., 2017.
OpenGridMap: towards automatic power grid simulation
model generation from crowdsourced data. Comput. Sci.
- Res. Dev. 32, 13–23. https://doi.org/10.1007/s00450-
016-0317-4
Triebke, H., Göhler, G., Wagner, D.S., 2016. Data analysis
of PEV charging events in rural and business
environments – a load behaviour comparison - 20.
Valdes, J., Wuth, J., Zink, R., Schröck, S., Schmidbauer, M.,
2019. Extracting relevant Points of Interest from Open
Street Map to support E-Mobility Infrastructure Models.
Bavar. J. Appl. Sci. 4.
Voulis, N., Warnier, M., Brazier, F.M.T., 2018.
Understanding spatio-temporal electricity demand at
different urban scales: A data-driven approach. Appl.
Energy 230, 1157–1171.
https://doi.org/10.1016/j.apenergy.2018.08.121
Zink, R., Pagany, R., Marquardt, A., 2017. OSM-basierte
Standortmodellierung von Ladesäulen für
Elektromobilität am Beispiel des Bayerischen Waldes,
in: Tagungsband Der FOSSGISS 2017:
Anwenderkonferenz Für Freie Und Open Source
Software Für Geoinformationssysteme (Universität Pas-
sau; 22.-25.03.2017). FOSSGISS e.V. (Ed.), pp. 59–64.
Energy Modelling in Rural Areas with Spatial and Temporal Data in Germany and Czech Republic
227