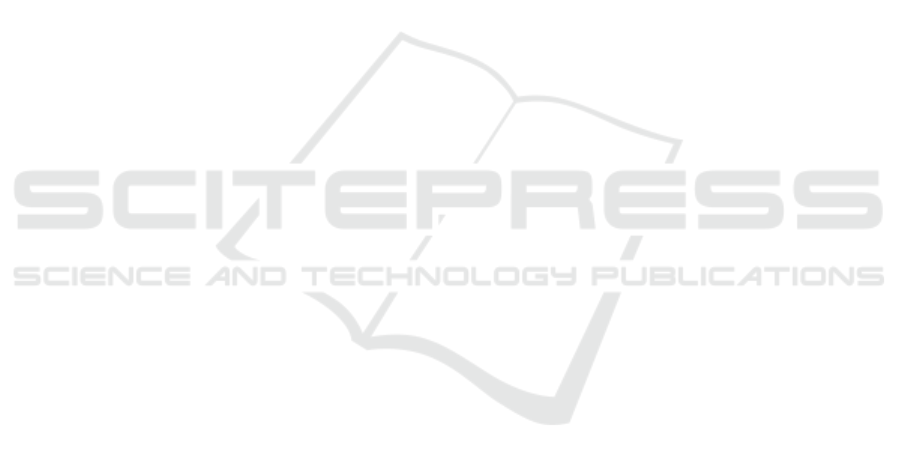
REFERENCES
Allcott, H. (2009). Real time pricing and electricity mar-
kets. Harvard University, 7.
Aslam, T. and Arshad, N. (2018). Soft load shedding: An
efficient approach to manage electricity demand in a
renewable rich distribution system. In Proceedings of
the 7th International Conference on Smart Cities and
Green ICT Systems - Volume 1: SMARTGREENS,,
pages 101–107. INSTICC, SciTePress.
Bashir, N., Sharani, Z., Qayyum, K., and Syed, A. A.
(2015). Delivering smart load-shedding for highly-
stressed grids. In Smart Grid Communications
(SmartGridComm), 2015 IEEE International Confer-
ence on, pages 852–858. IEEE.
Chandan, V., Ganu, T., Wijaya, T. K., Minou, M., Sta-
moulis, G., Thanos, G., and Seetharam, D. P. (2014).
idr: Consumer and grid friendly demand response sys-
tem. In Proceedings of the 5th international confer-
ence on Future energy systems, pages 183–194. ACM.
Chen, H., Cong, T. N., Yang, W., Tan, C., Li, Y., and Ding,
Y. (2009). Progress in electrical energy storage sys-
tem: A critical review. Progress in natural science,
19(3):291–312.
Chrysikou, V., Alamaniotis, M., and Tsoukalas, L. H.
(2015). A review of incentive based demand response
methods in smart electricity grids. International Jour-
nal of Monitoring and Surveillance Technologies Re-
search (IJMSTR), 3(4):62–73.
Erdinc, O., Tascikaraoglu, A., Paterakis, N., and Catal
˜
ao,
J. P. (2018). Novel incentive mechanism for end-
users enrolled in dlc-based demand response pro-
grams within stochastic planning context. IEEE
Transactions on Industrial Electronics.
Holyhead, J. C., Ramchurn, S. D., and Rogers, A. (2015).
Consumer targeting in residential demand response
programmes. In Proceedings of the 2015 ACM Sixth
International Conference on Future Energy Systems,
pages 7–16. ACM.
Hussain, I., Mohsin, S., Basit, A., Khan, Z. A., Qasim, U.,
and Javaid, N. (2015). A review on demand response:
Pricing, optimization, and appliance scheduling. Pro-
cedia Computer Science, 52:843–850.
Lu, R., Hong, S. H., and Zhang, X. (2018). A dynamic
pricing demand response algorithm for smart grid:
Reinforcement learning approach. Applied Energy,
220:220–230.
McKenna, E., Pless, J., and Darby, S. J. (2018). Solar pho-
tovoltaic self-consumption in the uk residential sec-
tor: New estimates from a smart grid demonstration
project. Energy Policy, 118:482–491.
Obi, M., Jensen, S., Ferris, J. B., and Bass, R. B. (2017).
Calculation of levelized costs of electricity for vari-
ous electrical energy storage systems. Renewable and
Sustainable Energy Reviews, 67:908–920.
Raza, M. Q., Nadarajah, M., and Ekanayake, C. (2016). On
recent advances in pv output power forecast. Solar
Energy, 136:125–144.
Stenner, K., Frederiks, E. R., Hobman, E. V., and Cook, S.
(2017). Willingness to participate in direct load con-
trol: The role of consumer distrust. Applied energy,
189:76–88.
Xia, B., Ming, H., Lee, K.-Y., Li, Y., Zhou, Y., Bansal, S.,
Shakkottai, S., and Xie, L. (2017). Energycoupon:
A case study on incentive-based demand response in
smart grid. In Proceedings of the Eighth International
Conference on Future Energy Systems, pages 80–90.
ACM.
Zou, P., Chen, Q., Yu, Y., Xia, Q., and Kang, C. (2017).
Electricity markets evolution with the changing gener-
ation mix: An empirical analysis based on china 2050
high renewable energy penetration roadmap. Applied
energy, 185:56–67.
SMARTGREENS 2019 - 8th International Conference on Smart Cities and Green ICT Systems
142