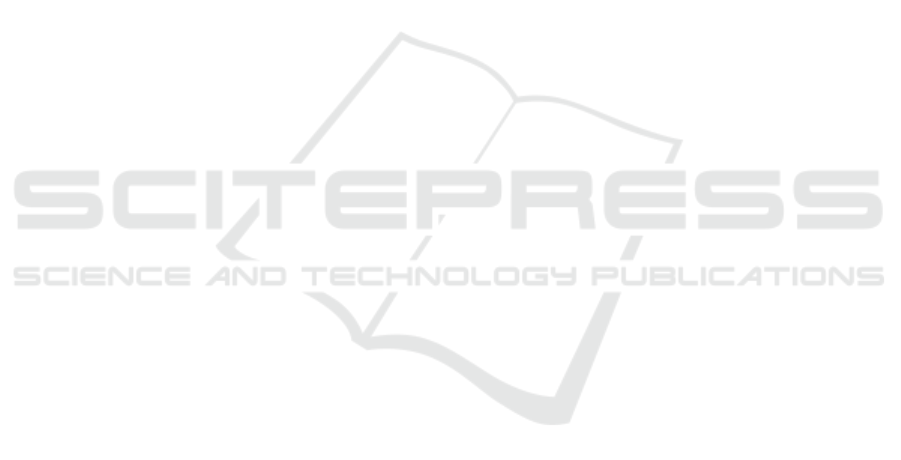
maximum drawdown. Expert Systems with Applica-
tions, 87:267–279.
Berutich, J. M., L
´
opez, F., Luna, F., and Quintana, D.
(2016). Robust technical trading strategies using gp
for algorithmic portfolio selection. Expert Systems
with Applications, 46:307–315.
Chang, P.-C., Liao, T. W., Lin, J.-J., and Fan, C.-Y.
(2011). A dynamic threshold decision system for
stock trading signal detection. Applied Soft Comput-
ing, 11(5):3998–4010.
Cheng, C.-H., Chen, T.-L., and Wei, L.-Y. (2010). A hybrid
model based on rough sets theory and genetic algo-
rithms for stock price forecasting. Information Sci-
ences, 180(9):1610–1629.
Chien, Y.-W. C. and Chen, Y.-L. (2010). Mining associative
classification rules with stock trading data–a ga-based
method. Knowledge-Based Systems, 23(6):605–614.
Dulac-Arnold, G., Evans, R., van Hasselt, H., Sunehag,
P., Lillicrap, T., Hunt, J., Mann, T., Weber, T., De-
gris, T., and Coppin, B. (2015). Deep reinforcement
learning in large discrete action spaces. arXiv preprint
arXiv:1512.07679.
Guresen, E., Kayakutlu, G., and Daim, T. U. (2011). Us-
ing artificial neural network models in stock market
index prediction. Expert Systems with Applications,
38(8):10389–10397.
jpmorgan. jpmorgan. https://www.businessinsider.com/jpm
organ-takes-ai-use-to-the-next-level-2017-8.
Koutn
´
ık, J., Schmidhuber, J., and Gomez, F. (2014). Online
evolution of deep convolutional network for vision-
based reinforcement learning. In International Con-
ference on Simulation of Adaptive Behavior, pages
260–269. Springer.
Krollner, B., Vanstone, B., and Finnie, G. (2010). Financial
time series forecasting with machine learning tech-
niques: A survey.
Lange, S. and Riedmiller, M. (2010). Deep auto-encoder
neural networks in reinforcement learning. In The
2010 International Joint Conference on Neural Net-
works (IJCNN), pages 1–8. IEEE.
Lange, S., Riedmiller, M., and Voigtlander, A. (2012). Au-
tonomous reinforcement learning on raw visual input
data in a real world application. In Neural Networks
(IJCNN), The 2012 International Joint Conference on,
pages 1–8. IEEE.
Liao, Z. and Wang, J. (2010). Forecasting model of global
stock index by stochastic time effective neural net-
work. Expert Systems with Applications, 37(1):834–
841.
Lillicrap, T. P., Hunt, J. J., Pritzel, A., Heess, N., Erez, T.,
Tassa, Y., Silver, D., and Wierstra, D. (2015). Contin-
uous control with deep reinforcement learning. arXiv
preprint arXiv:1509.02971.
Mabu, S., Obayashi, M., and Kuremoto, T. (2015). En-
semble learning of rule-based evolutionary algorithm
using multi-layer perceptron for supporting decisions
in stock trading problems. Applied soft computing,
36:357–367.
Mnih, V., Kavukcuoglu, K., Silver, D., Graves, A.,
Antonoglou, I., Wierstra, D., and Riedmiller, M.
(2013). Playing atari with deep reinforcement learn-
ing. arXiv preprint arXiv:1312.5602.
Mnih, V., Kavukcuoglu, K., Silver, D., Rusu, A. A., Veness,
J., Bellemare, M. G., Graves, A., Riedmiller, M., Fid-
jeland, A. K., Ostrovski, G., et al. (2015). Human-
level control through deep reinforcement learning.
Nature, 518(7540):529.
Schaul, T., Quan, J., Antonoglou, I., and Silver, D.
(2015). Prioritized experience replay. arXiv preprint
arXiv:1511.05952.
Schulman, J., Levine, S., Abbeel, P., Jordan, M., and
Moritz, P. (2015). Trust region policy optimization.
In International Conference on Machine Learning,
pages 1889–1897.
Shibata, K. and Iida, M. (2003). Acquisition of box
pushing by direct-vision-based reinforcement learn-
ing. In SICE 2003 Annual Conference, volume 3,
pages 2322–2327. IEEE.
Shibata, K. and Okabe, Y. (1997). Reinforcement learn-
ing when visual sensory signals are directly given as
inputs. In Neural Networks, 1997., International Con-
ference on, volume 3, pages 1716–1720. IEEE.
Silver, D., Lever, G., Heess, N., Degris, T., Wierstra, D., and
Riedmiller, M. (2014). Deterministic policy gradient
algorithms. In ICML.
Van Hasselt, H., Guez, A., and Silver, D. (2016). Deep rein-
forcement learning with double q-learning. In AAAI,
volume 2, page 5. Phoenix, AZ.
Vanstone, B., Finnie, G., and Hahn, T. (2012). Creating
trading systems with fundamental variables and neu-
ral networks: The aby case study. Mathematics and
computers in simulation, 86:78–91.
Wang, J., Hou, R., Wang, C., and Shen, L. (2016). Improved
v-support vector regression model based on variable
selection and brain storm optimization for stock price
forecasting. Applied Soft Computing, 49:164–178.
Wang, J.-Z., Wang, J.-J., Zhang, Z.-G., and Guo, S.-P.
(2011). Forecasting stock indices with back propa-
gation neural network. Expert Systems with Applica-
tions, 38(11):14346–14355.
Wang, Z., Schaul, T., Hessel, M., Van Hasselt, H., Lanc-
tot, M., and De Freitas, N. (2015). Dueling network
architectures for deep reinforcement learning. arXiv
preprint arXiv:1511.06581.
Wymann, B., Espi
´
e, E., Guionneau, C., Dimitrakakis, C.,
Coulom, R., and Sumner, A. (2000). Torcs, the
open racing car simulator. Software available at
http://torcs. sourceforge. net, 4:6.
COMPLEXIS 2019 - 4th International Conference on Complexity, Future Information Systems and Risk
58