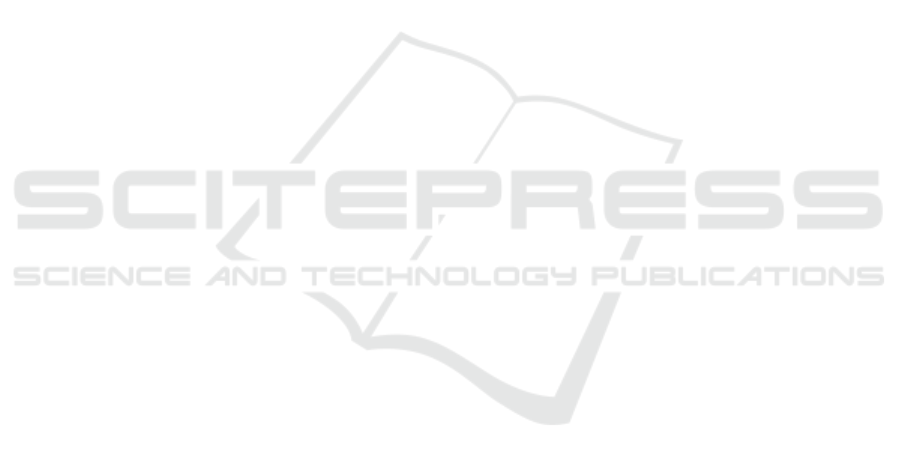
6.6 Assign Status
The threshold parameters i.e., min, mean, and
percentile (75) (Table 1) are used to assign a status,
"no cut yet", "in progress", and "finish" in the final
step. Then, status information is sending to the GI
System for utilization in the final step.
7 CONCLUSIONS
This paper presents a proof of concept of using
Sentinel-2 data in the harvest monitoring of
sugarcane fields. SAVI can be used as a cutting
indicator in harvest period of farm plants. In rainfed
agriculture such as Thailand, recommended the
minimum of 15 days composite for cloud-free image
analysis. Geostatistics is a powerful tool for
interpretation in various ways to identify the human
activities which perform on farmland as
demonstrated in this paper.
REFERENCES
European Space Agency, (n.d.). Open Access Hub.
(European Union) Retrieved 1230 2018, from
https://scihub.copernicus.eu/userguide
/ODataAPI
European Space Agency, (n.d.). ESA Sentinels Help
Monitor Grasslands for Agricultural Subsidy Checks
in Europe. Retrieved 12 25, 2018, from
http://www.dhi-gras.com/news/2017/4/7
/successful-demonstration-of-using-sentinel-1-and-2
Thai Meteorological Department, (2015). The Climate of
Thailand.
Rondeaux, G., Steven, M., Baret, F., (1996). Optimization
of Soil-Adjusted Vegetation Indices. Remote Sensing
of Environment, 55(2), 95-107.
Holben, B. N., 2007. Characteristics of maximum-value
composite images from temporal AVHRR data.
International Journal of Remote Sensing, 7(11), 1417-
1434.
Huete, A. R., 1988. A soil-adjusted vegetation index
(SAVI). Remote Sensing of Environment, 25(3), 295-
309.
Louis, J., Debaecker, V., Pflug, B., Main-Knorn, M.,
Bieniarz, J., Mueller-Wilm, U., Cadau, E., Gascon, F.,
2016. SENTINEL-2 SEN2COR: L2A PROCESSOR
FOR USERS. Living Planet Symposium. Prague,
Czech Republic.
Jasinski, M. F., Eagleson, P. S., 1990. Estimation of
Subpixel Vegetation Cover Using Red-Infrared
Scattergrams. IEEE TRANSACTIONS ON
GEOSCIENCE AND REMOTE SENSING, 28(2), 253-
267.
Chetthamrongchai, P., Auansakul, A., Decha Supawan,
D., 2001. assessing the transportation problems of the
sugar cane. Economic and Social Commission for
Asia and the Pacific.
Sentinel Hub. (n.d.). Retrieved 12 30, 2018, from
https://www.sentinel-
hub.com/develop/documentation/eo_products/Sentinel
2EOproducts
Bontemps, S., Arias, M., Cara, C., Dedieu, G., Guzzonato,
E., Hagolle, O., Inglada, J., Matton, N., Morin, D.,
Popescu, R., Rabaute, T., Savinaud, M., Sepulcre, G.,
Valero, S., Ahmad, I., Bégué, A., Wu, B., Abelleyra,
D., Diarra, A., Dupuy, S., French, A., Akhtar, I. H.,
Kussul, N., Levourgeois, V., Page, M. L., Newby, T.,
Savin, I., Verón, S. R., Koetz, B., Defourny, P.,
(2015). Building a Data Set over 12 Globally
Distributed Sites to Support the Development of
Agriculture Monitoring Applications with Sentinel-2.
Remote Sensing, 7, 16062-16090.
Kanjir, U., Đurić, N., Veljanovski, T., 2018. Sentinel-2
Based Temporal Detection of Agricultural Land Use
Anomalies in Support of Common Agricultural Policy
Monitoring. Geo-Information.
Devos, W., Fasbender, D., Lemonine, G., Loudjani, P.,
Milenov, P., Wimhardt, C., 2017. Discussion
document on the introduction of monitoring to
substitute OTSC. European Commission.
Sentinel-2 based Remote Evaluation System for a Harvest Monitoring of Sugarcane Area in the Northeast Thailand Contract Farming
241