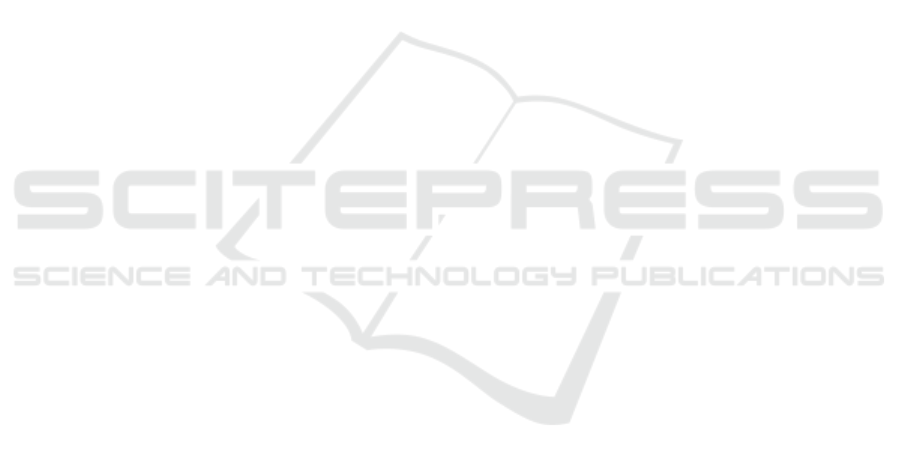
5 CONCLUSIONS
In this paper, we introduced a new solution that aims
to improve the knowledge representation and rea-
soning with uncertain knowledge in fuzzy ontolo-
gies. The proposed solution is described by a gen-
eral process, which takes as an input a fuzzy ontol-
ogy and outputs a probabilistic fuzzy ontology. The
merit of our proposal is that it can represent and rea-
son with rich-uncertainty domains, where it models
the vague, imprecise and probabilistic knowledge si-
multaneously and combines the ontological inference
with the fuzzy probabilistic inference.
As future works, we are looking to present real cases
studies with our proposed approach to show its poten-
tial applicability in terms of modeling and reasoning.
Moreover, we are looking to implement an interactive
prot
´
eg
´
e-plugin in order to help ontology developers to
follow our proposed process.
REFERENCES
Bobillo, F., Delgado, M., and G
´
omez-Romero, J. (2012).
Delorean: A reasoner for fuzzy owl 2. Expert Systems
with Applications, 39(1):258–272.
Bobillo, F. and Straccia, U. (2011). Fuzzy ontology rep-
resentation using owl 2. International Journal of Ap-
proximate Reasoning, 52(7):1073–1094.
Bobillo, F. and Straccia, U. (2016). The fuzzy ontology
reasoner fuzzydl. Knowledge-Based Systems, 95:12–
34.
Carvalho, R. N., Laskey, K. B., and Costa, P. C. (2017).
Pr-owl–a language for defining probabilistic ontolo-
gies. International Journal of Approximate Reason-
ing, 91:56–79.
Costa, P. C., Ladeira, M., Carvalho, R. N., Laskey, K. B.,
Santos, L. L., and Matsumoto, S. (2008). A first-order
bayesian tool for probabilistic ontologies. In Proceed-
ings of the Twenty-First International Florida Artifi-
cial Intelligence Research Society Conference, pages
631–636.
Dempster, A. P., Laird, N. M., and Rubin, D. B. (1977).
Maximum likelihood from incomplete data via the em
algorithm. Journal of the Royal Statistical Society:
Series B (Methodological), 39(1):1–22.
Ding, Z., Peng, Y., and Pan, R. (2006). Bayesowl: Uncer-
tainty modeling in semantic web ontologies. In Soft
computing in ontologies and semantic web, pages 3–
29. Springer.
Emna, H., Salma, J., Mohamed, T., and Abdelmajid, B. H.
(2016a). Building probabilistic ontologies based on
meta-model podm. In International Conference on
Hybrid Intelligent Systems, pages 288–297. Springer.
Emna, H., Salma, J., Mohamed, T., and Abdelmajid,
B. H. (2016b). Probabilistic ontology definition meta-
model. In Intelligent Decision Technologies 2016,
pages 243–254. Springer.
Hlel, E., Jamoussi, S., and Hamadou, A. B. (2018). A new
method for building probabilistic ontology (prob-ont).
In Information Retrieval and Management: Concepts,
Methodologies, Tools, and Applications, pages 1409–
1434. IGI Global.
Ishak, M. B., Leray, P., and Amor, N. (2011). Ontology-
based generation of object oriented bayesian net-
works. In Bayesian Modeling Applications Workshop
(BMAW-11). Citeseer.
Laskey, K. B. (2008). Mebn: A language for first-order
bayesian knowledge bases. Artificial intelligence,
172(2-3):140–178.
Li, X. (2009). On the use of virtual evidence in conditional
random fields. In Proceedings of the 2009 Conference
on Empirical Methods in Natural Language Process-
ing: Volume 3-Volume 3, pages 1289–1297. Associa-
tion for Computational Linguistics.
Mohammed, A.-W., Xu, Y., and Liu, M. (2016).
Knowledge-oriented semantics modelling towards un-
certainty reasoning. SpringerPlus, 5(1):706.
Mrad, A. B., Delcroix, V., Maalej, M. A., Piechowiak, S.,
and Abid, M. (2012). Uncertain evidence in bayesian
networks: Presentation and comparison on a simple
example. In International Conference on Informa-
tion Processing and Management of Uncertainty in
Knowledge-Based Systems, pages 39–48. Springer.
Pan, R., Ding, Z., Yu, Y., and Peng, Y. (2005). A bayesian
network approach to ontology mapping. In Inter-
national Semantic Web Conference, pages 563–577.
Springer.
Peng, Y., Zhang, S., and Pan, R. (2010). Bayesian network
reasoning with uncertain evidences. International
Journal of Uncertainty, Fuzziness and Knowledge-
Based Systems, 18(05):539–564.
Ryhajlo, N., Sturlaugson, L., and Sheppard, J. W. (2013).
Diagnostic bayesian networks with fuzzy evidence. In
2013 IEEE AUTOTESTCON, pages 1–8. IEEE.
Setiawan, F. A., Wibowo, W. C., and Ginting, N. B. (2015).
Handling uncertainty in ontology construction based
on bayesian approaches: A comparative study. In
International Conference on Soft Computing, Intel-
ligence Systems, and Information Technology, pages
234–246. Springer.
Yang, Y. and Calmet, J. (2005). Ontobayes: An ontology-
driven uncertainty model. In International Conference
on Computational Intelligence for Modelling, Control
and Automation and International Conference on In-
telligent Agents, Web Technologies and Internet Com-
merce (CIMCA-IAWTIC’06), volume 1, pages 457–
463. IEEE.
Zadeh, L. A. (1975). The concept of a linguistic variable
and its application to approximate reasoning-iii. In-
formation sciences, 9(1):43–80.
ICEIS 2019 - 21st International Conference on Enterprise Information Systems
414