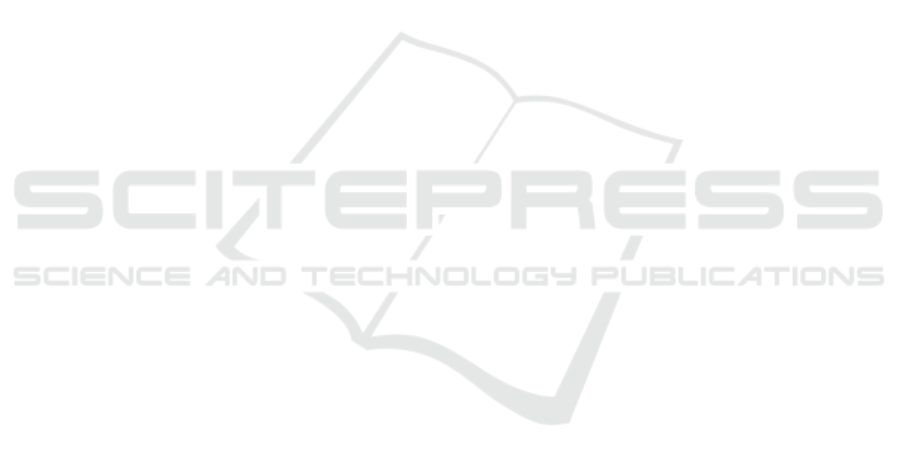
of 91 % and 89.8 % as shown in Table 2. In our future
analysis, we plan to test the performance of the
proposed model on a larger dataset.
REFERENCES
Artan, Y., Bulan, O., Loce, R. P., Paul, P., (2016) Passenger
compartment violation detection in HOV/HOT lanes, in
IEEE Transactions on Intelligent Transportation
Systems, vol. 17, no. 2, 395-405, 2016.
Seshadri, K., Juefei-Xu, F., Pal, D. K., Savvides, M., Thor,
C., (2015). Driver cell phone usage detection on
Strategic Highway Research Program (shrp2) face view
videos", in CVPR’2015, IEEE Conf. CVPRW, pp. 35-
43, 2015.
Elihos, A., Alkan, B., Balci, B., Artan, Y., (2018).
Comparison of Image Classification and Object
Detection for Passenger Seat Belt Violation Detection
Using NIR & RGB Surveillance Camera Images, In
AVSS’2018, IEEE Conference on Advanced Visual
Surveillance Systems, 2018.
Balci, B., Alkan, B., Elihos, A., Artan, Y., (2018). Front
Seat Child Occupancy Detection in Roadway
Surveillance Images, In ICIP’2018, IEEE Conference
on Image Processing, 2018.
Simonyan, K., Zisserman, A., (2014). Very Deep
Convolutional Networks for Large-Scale Image
Recognition, arXiv:1409.1556, 2014.
Liu, W., Anguelov, D., Erhan, D., Szegedy, C., Reed, S.,
Fu, C., Berg., A., (2016). “SSD: Single shot multibox
detector.” In ECCV’2016. European Conference on
Computer Vision, pp. 21–37, Springer, 2016.
Huang, J., Rathod, V., Sun, C., Zhu, M., Korattika, A.,
Fathi, A., Fischer, I., Wojna, Z., Song, Y., Guadarrama,
S., Murphy, K. (2017). Speed/accuracy trade-offs for
modern convolutional object detectors, arXiv:
1611.10012v3, 2017.
IBM, (2012). IBM Smarter Cities Public Safety, Law
Enforcement Report, 2012.
Patil, K., Kulkarn, M., Sriraman, A., Karande, S., (2017).
Deep Learning Based Car Damage Classification, In
ICMLA’2017, IEEE Conference on Mac. Learn. App.,
2017.
Kanacı, A., Zhu, X., Gong, S., (2017). Vehicle
reidentification by Fine grained cross-level deep
learning, In British Machine Vision Conference
(BMVC), 2017.
Blacoe, W., Lapata, M., (2012). A comparison of Vector
based Representation for Semantic Composition, In
Proceedings of 2012 Joint Conference on Emprical
Methods in natural Language Processing and
Computational Natural Language Learning, pp. 546-
556, 2012.
Jayawardena, S., (2013). Image based automatic vehicle
damage detection. Ph.D. Dissertation, Australian
National University, 2013.
Li, P., Shen, B.Y., Dong, W., (2018). An Anti-fraud System
for Car Insurance Claim Based on Visual Evidence,
arXiv:1804.11207v1, 2018.
Cha, Y.J., Choi, W., Buyukozturk, O., (2017). Deep
Learning based crack damage detection using
convolutional neural networks, Computer Aided Civil
and Infrastructure Engineering, 32(5), 361-378, 2017.
MaskRCNN,(2018).https://www.analyticsvidhya.com/blo
g/2018/07/building-mask-r-cnn-model-detecting-
damage-cars-python
Tractable, (2018). https://www.tractable.io
Szegedy, C., Vanhoucke, V., Ioffe, S., Shlens J., Wojna, Z.,
(2015). Rethinking the Inception Architecture in
Computer Vision, arXiv: 1512.00567v3, 2015.
Deng, J., Dong, W., et al.,, (2009). ImageNet: A large scale
hierarchical image database, in CVPR’2009. IEEE
Conference on Computer Vision and Pattern
Recognition (CVPR), 2009.
Everingham, M., Gool, L.V., Williams, W.K.I, Winn, J.,
Zisserman, A., (2010). The PASCAL Visual Object
Classes (VOC) Challenge, IJCV, 2010.
Razavian, A.S., et al. (2014). CNN features off the shelf:
An astounding Baseline for Recognition, arXiv:
1403.6382v3.
VEHITS 2019 - 5th International Conference on Vehicle Technology and Intelligent Transport Systems
198