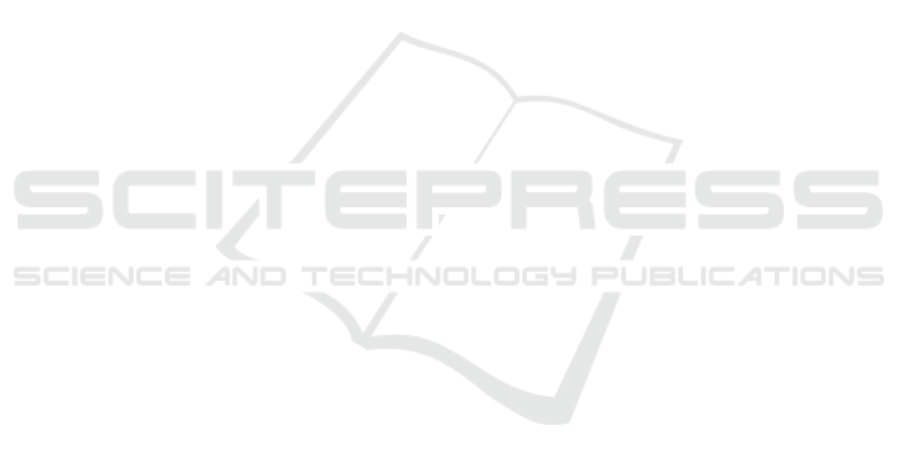
REFERENCES
Branch, J. W., Giannella, C., Szymanski, B., Wolff, R., and
Kargupta, H. (2013). In-network outlier detection in
wireless sensor networks. Knowledge and information
systems, vol. 34, no. 1.
Brotherton, T. and Mackey, R. (2001). Anomaly detector
fusion processing for advanced military aircraft. pages
3125–3137. IEEE Proceedings on Aerospace Confer-
ence.
Chakravarty, T., G. A. B. C. C. A. (2013). Mo-bi drive
score a system for mobile sensor based driving analy-
sis: A risk assessment model for improving one’s driv-
ing. pages 338–344. 7th International Conference on
Sensing Technology (ICST),IEEE.
Checkoway, S., McCoy, D., Kantor, B., Anderson, D.,
Shacham, H., Savage, S., Koscher, K., Czeskis, A.,
Roesner, F., and Kohno, T. (2011). Comprehensive
experimental analyses of automotive attack surfaces.
San Francisco, USA. USENIX Security Symposium.
Chen, Z., Y. J. Z. Y. C. Y. L. M. . (2015). Abnormal driv-
ing behaviors detection and identification using smart-
phone sensors. pages 524–532. 12th Annual IEEE In-
ternational Conference on Sensing, Communication,
and Networking (SECON), IEEE.
Cork, L. and Walker, R. (2007). Sensor fault detection for
uavs using a nonlinear dynamic model and the imm-
ukf algorithm. pages 230–235. IDC.
Goel, P., Dedeoglu, G., Roumeliotis, S. I., and Sukhatme,
G. S. (2000). Fault-detection and identification in
a mobile robot using multiple model estimation and
neural network. ICRA-00.
Hochreiter, S. and Schmidhuber, J. (1997). Long short-term
memory. Neural computation, 9(8):1735–1780.
Hong, J. H., M. B. D. A. K. (2014). A smartphone-based
sensing platform to model aggressive driving behav-
iors. pages 4047–4056. 32nd Annual ACM confer-
ence on Human Factors in Computing Systems.
Koscher, K., Czeskis, A., Roesner, F., Patel, S., Kohno, T.,
Checkoway, S., McCoy, D., Kantor, B., Anderson, D.,
and Shacham, H. (2010). Experimental security anal-
ysis of a modern auto- mobile. in security and privacy
(sp). page 447–462, Stamford, CT, USA. IEEE Sym-
posium.
Laurikkala, J., Juhola, M., and Kentala, E. (2000). Infor-
mal identification of outliers in medical data. Fifth
International Workshop on Intelligent Data Analysis
in Medicine and Pharmacology.
Lin, R., Khalastchi, E., and Kaminka, G. A. (2010). Detect-
ing anomalies in unmanned vehicles using the maha-
lanobis distance. pages 3038–3044. ICRA.
Malhotra, P., Vig, L., Shroff, G., and Agarwal, P. (2015).
Long short term memory networks for anomaly de-
tection in time series. 23rd Euro- pean Symposium on
Artificial Neural Networks.
Miller, C. and Valasek, C. (2015). Remote exploitation of
an unaltered passenger vehicle.
Petit, J. and Shladover, S. E. (2015). Potential cyberattacks
on automated vehicles. IEEE Transactions on Intelli-
gent Transportation Systems, 16(2):546–556.
Pokrajac, D. (2007). Incremental local outlier detection for
data streams. IEEE Symposium on Computational In-
telligence and Data Mining.
Rajasegarar, S., Leckie, C., and Palaniswami, M. (2008).
Anomaly detection in wireless sensor networks. IEEE
Wireless Communications, vol. 15, no. 4.
Simulator, A. C. (2018). University of waterloo.
Sundvall, P. and Jensfelt, P. (2006). Fault detection for
mobile robots using redundant positioning systems.
ICRA-06.
Tian, Y., Pei, K., Jana, S., and Ray, B. (2018). Deeptest:
Automated testing of deep-neural-network-driven au-
tonomous cars. In Proceedings of the 40th Inter-
national Conference on Software Engineering, ICSE
’18, pages 303–314, New York, NY, USA. ACM.
Velosa, A., Hines, J., LeHong, H., and Perkins, E. (2014).
Predicts 2015: The Internet of Things. Gartner, Stam-
ford, CT, USA.
Zhang, Y., Meratnia, N., and Havinga, P. J. (2013). Dis-
tributed online outlier detection in wireless sensor net-
works using ellipsoidal support vector machine. Ad
hoc networks, vol. 11, no. 3.
VEHITS 2019 - 5th International Conference on Vehicle Technology and Intelligent Transport Systems
482