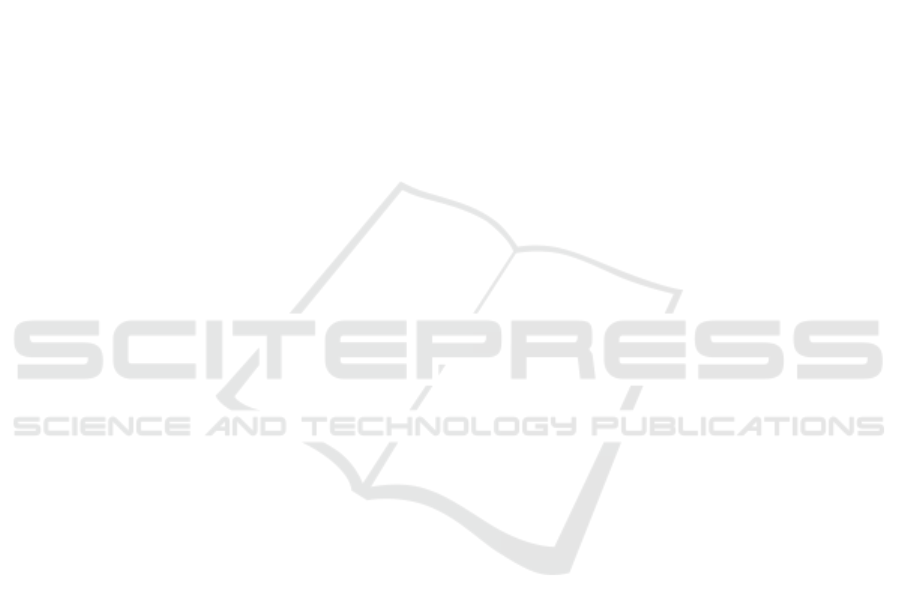
ad texts by hints to missing information in the user
text. An evaluation of the support functionality in the
field could not be carried out within the scope of the
present work.
The validation examined the determination of text
modules based on changing input texts, which are
returned to the caller (client). It could be shown that
AS recognizes keywords in the input texts and
correctly recognizes the content to be checked. Hints,
which are assigned as text modules to a text category,
were selected by AS and transmitted to the client if
there were no information in the input text. The hint
texts do not always fit into the context of the input
text, which was already clear when defining the text
categories.
The validation showed that the prototype meets
the requirements of an independent solution (R1),
which can process and analyse the input texts
independently (R6) based on a self-administered
database (R3). Missing information in the input text
is recognized and corresponding information texts
(R5) are returned to the client. AS learns by storing
new ad text data (R4) in its own database. No user
data is analysed (R7) or stored (R8) for processing.
With an average response time of 1.6 seconds, AS
can promptly generate a response, enabling the client
to provide the user with the information during ad text
capturing (R2). By providing the services as a web
service, the assistant can be easily integrated into
existing interfaces (R9).
The support function of AS is limited by its rough
rasterization into four text categories. This is partly
due to an insufficient named entity recognition of the
German language model. Currently, this model can
only detect locations relatively reliably. Other
information must be searched with predefined
keywords in the input text.
8 CONCLUSIONS
The solution presented in this paper will enable users
in the future (i.e. after integration into the app) to be
supported in entering ad texts in the meinDorf55+
app. For this purpose, questions about potentially
missing aspects of the content should create
incentives to supplement the missing information.
This should improve both the quality of the ads and
the recommendations generated by the app in the
"Handeln" area. A detailed evaluation of the assistant
must be carried out.
The object of further research activities is the
acquisition of additional external data and the
integration of user-specific information from the
system in order to be able to open up the context of
the individual user and to enable adequate support.
Future work will also focus on improving German
language processing.
REFERENCES
Abdul-Kader, S. A., Woods, J. C., 2015. Survey on chatbot
design techniques in speech conversation
systems. International Journal of Advanced Computer
Science and Applications, 6(7).
Agarwal, D. K., Chen, B. C., 2015. Statistical methods for
recommender systems. Cambridge University Press.
Carstensen, K. U., Ebert, C., Ebert, C., Jekat, S., Langer,
H., Klabunde, R. (Eds.)., 2010. Computerlinguistik und
Sprachtechnologie: Eine Einführung. Springer-Verlag.
Conrad, C. S. 2018. Kann die Künstliche Intelligenz den
Menschen entschlüsseln? - Neue Forderungen zum
Datenschutz. Datenschutz und Datensicherheit-DuD,
42(9), 541-546.
Jiang, S., de Rijke, M., 2018. Why are Sequence-to-
Sequence Models So Dull? Understanding the Low-
Diversity Problem of Chatbots. arXiv preprint
arXiv:1809.01941.
Naw, N., Hlaing, E. E., 2013. Relevant words extraction
method for recommendation system. Bulletin of
Electrical Engineering and Informatics, 2(3), 169-176.
Schein, A. I., Popescul, A., Ungar, L. H., Pennock, D. M.,
2002. Methods and metrics for cold-start
recommendations. In Proceedings of the 25th annual
international ACM SIGIR conference on Research and
development in information retrieval (pp. 253-260).
ACM.
Skowron, M., Irran, J., Krenn, B., 2008. Computational
framework for and the realization of cognitive agents
providing intelligent assistance capabilities. In 18th
European Conference on Artificial Intelligence,
Cognitive Robotics Workshop (pp. 88-96).
Sordoni, A., Galley, M., Auli, M., Brockett, C., Ji, Y.,
Mitchell, M., Dolan, B., 2015. A neural network
approach to context-sensitive generation of
conversational responses. arXiv preprint
arXiv:1506.06714.
Stanoevska-Slabeva, K., 2018. Conversational Interfaces –
die Benutzerschnittstelle der Zukunft?
Wirtschaftsinformatik & Management, 10(6), 26-37.
Stäcker, O., Stanoevska-Slabeva, K., 2018. Quo vadis
Chatbots? Wirtschaftsinformatik &
Management, 10(6), 38-46.
Thelwall, M., Buckley, K., Paltoglou, G., Cai, D., Kappas,
A., 2010. Sentiment strength detection in short informal
text. Journal of the American Society for Information
Science and Technology, 61(12), 2544-2558.
Vinyals, O., Le, Q., 2015. A neural conversational model.
arXiv preprint arXiv:1506.05869.
ICEIS 2019 - 21st International Conference on Enterprise Information Systems
430