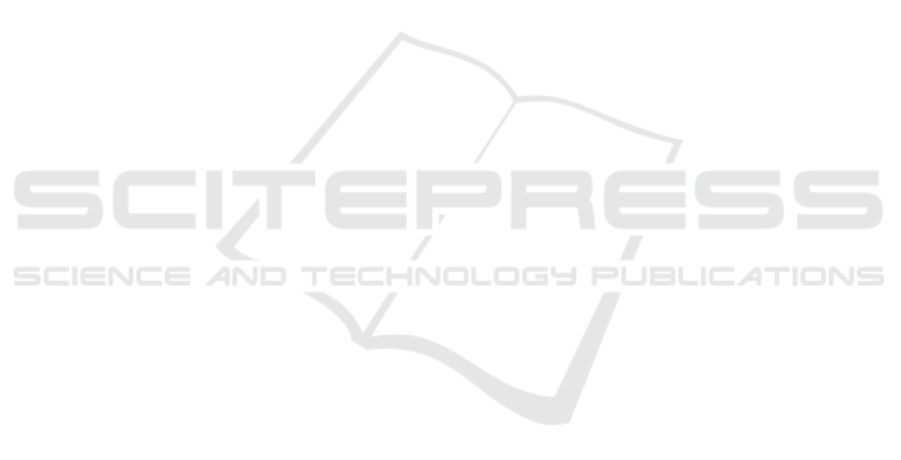
Hybrid Energy Storage Considering Degradation Costs.
In IEEE Transactions on Smart Grid, vol. 9, pp. 6047-
6057.
Calderaro, V., Galdi, V., Graber, G., Graditi, G., Lamberti,
F., 2014. Impact assessment of energy storage and
electric vehicles on smart grids. In Proc. Electric Power
Quality and Supply Reliability Conf., pp 15-18.
Graber, G., Lamberti, F., Calderaro, V., Galdi, V., Piccolo,
A., 2017. Stochastic characterization of V2G parking
areas for the provision of ancillary services. In Proc.
Innovative Smart Grid Technologies Conf. Europe,
pp.1-6.
Viswanathan, V. V., Kintner-Meyer, M., 2011. Second Use
of Transportation Batteries: Maximizing the Val ue of
Batteries for Transportation and Grid Services. In IEEE
Trans. Vehicular Technology, vol. 60, pp. 2963-2970.
Saez-de-Ibarra, A., Martinez-Laserna, E., Stroe, D.,
Swierczynski, M., Rodriguez, P., 2016. Sizing Study of
Second Life Li-ion Batteries for Enhancing Renewable
Energy Grid Integration. In IEEE Trans. on Industry
Applications, vol. 52, pp. 4999-5008.
Lacey, G., Putrus, G., Salim, A., 2013. The use of second
life electric vehicle batteries for grid support. In Proc.
IEEE EUROCON Conf., pp. 1255-1261.
Gladwin, D. T., Gould, C. R., Stone, D. A., Foster, M. P.,
2013. Viability of “second-life” use of electric and
hybridelectric vehicle battery packs. In Proc. IECON
Industrial Electronics Society Conf., pp. 1922-1927.
Koch-Ciobotaru, C., Saez-de-Ibarra, A., Martinez-Laserna,
E., Stroe, D. I., Swierczynski M., Rodriguez, P., 2015.
Second life battery energy storage system for enhancing
renewable energy grid integration. In Proc. Energy
Conversion Congress and Exposition Conf., pp. 78-84.
Mukherjee N., Strickland, D., 2015. Control of Second-Life
Hybrid Battery Energy Storage System Based on
Modular Boost-Multilevel Buck Converter. In IEEE
Trans. on Industrial Electronics, vol. 62, pp. 1034-
1046.
Gohla-Neudecker, B., Bowler M., Mohr, S., 2015. Battery
2nd life: Leveraging the sustainability potential of EVs
and renewable energy grid integration. In Proc. Clean
Electrical Power Conf., pp. 311-318.
Strickland, D., Chittock, L., Stone D. A., Foster, M. P.,
Price, B., 2014. Estimation of Transportation Battery
Second Life for Use in Electricity Grid Systems. In
IEEE Trans. on Sustainable Energy, vol. 5, pp. 795-
803.
Tong, S., Fung T., Park, J. W., 2015. Reusing electric
vehicle battery for demand side management
integrating dynamic pricing. In Proc. IEEE Smart Grid
Communications Conf., pp. 325-330.
Hamidi, A., Weber L., Nasiri, A., 2013. EV charging station
integrating renewable energy and second-life battery. In
Proc. Renewable Energy Research and Applications
Conf. pp. 1217-1221.
Julien, C., Mauger, A., Vijh, A., Zaghib, K., 2016. Lithium
Batteries - Science and Technology. Springer, 1
st
edition.
SAFT, May 2014. Lithium-ion battery life. Document
N°21893-2-0514 [Online]. Available: http://www.saft
batteries.com/force_download/li_ion_battery_life__Te
chnicalSheet_en_0514_Protected.pdf&prev=search
Masters, G., 2013. Renewable and Efficient Electric Power
Systems. Wiley, 2
nd
edition.
Calderaro, V., Galdi, V., Graber, G., Massa, G., Piccolo, A.,
2014. Plug-in EV charging impact on grid based on
vehicles usage data. In Proc. International Electric
Vehicle Conf., pp. 1-7.
Calderaro, V., Galdi, V., Graber, G., Lamberti, F., Piccolo,
A., 2017. A sizing method for economic assessment of
II-life batteries for power system applications. In Proc.
Power & Energy Society General Meeting, pp.1-5.
Graber, G., V., Galdi, Calderaro, V., Mancarella, P., 2017.
A stochastic approach to size EV charging stations with
support of second life battery storage systems. In Proc.
IEEE Manchester PowerTech, pp.1-6.
Reid, G., Julve, J., 2016. Second Life-Batteries as Flexible
Storage for Renewables Energies. Report. [Online].
Available: https://www.bee-ev.de/fileadmin/Publikatio
nen/Studien/201604_Second_Life-Batterien_als_flexi
ble_Speicher.pdf
Thirugnanam K., Kerk S. K., Yuen C., Liu N., Zhang M.,
2018. Energy Management for Renewable Micro-Grid
in Reducing Diesel Generators Usage with Multiple
Types of Battery. In IEEE Transactions on Industrial
Electronics, vol. 65, pp. 6772-6786.
SMARTGREENS 2019 - 8th International Conference on Smart Cities and Green ICT Systems
88