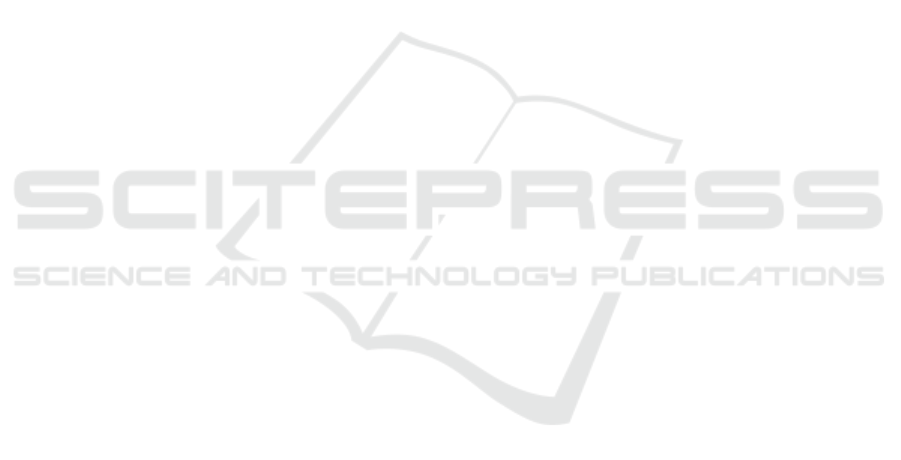
The experimentation shows that significant differ-
ences where found on five variables: animation, like-
ability, attractiveness, safety and usability. No signif-
icant differences were found on anthropomorphism,
perceived intelligence and hedonic quality.
The analysis of the participants’ recommendations
shows that they can potentially accept social robots in
the classroom if we come up with a better design. The
major improvements to be made are to support he-
donic qualities of identification and stimulation. The
stimulation goal could be achieved by using anthropo-
morphic characteristics such as voice, movement and
expression of emotion in order to make the robot more
interactive. The identification goal could be achieved
with the intelligence and animation characteristics of
the robot. They can be used to provide the students
with personalized feedback or to play an adaptive role
in collaborative situations.
In the next steps of our work we will implement
the discovered critical improvements and conduct a
new and larger study to confirm the insights that were
exposed in this work. This next study will also pro-
vide us with the opportunity to explore the idea to use
the social robot as a regulating tool for collaborative
learning activities.
REFERENCES
Bartneck, C., Croft, E., and Kulic, D. (2009). Measurement
instruments for the anthropomorphism, animacy, like-
ability, perceived intelligence, and perceived safety
of robots. International Journal of Social Robotics,
1(1):71–81.
Braun, V. and Clarke, V. (2006). Using thematic analysis in
psychology. Qualitative research in psychology, 3:77–
101.
Breazeal, C. (2003). Toward sociable robots. Robotics and
Autonomous Systems, 42(3):167–175.
Church, W. J., Ford, T., Perova, N., and Rogers, C. (2010).
Physics with robotics - using lego mindstorms in high
school education. In AAAI Spring Symposium: Edu-
cational Robotics and Beyond.
Dillenbourg, P. (1999). What do you mean by collaborative
learning? Oxford: Elsevier.
Hassenzahl, M., Burmester, M., and Koller, F. (2003).
AttrakDiff: Ein Fragebogen zur Messung
wahrgenommener hedonischer und pragmatis-
cher Qualit
¨
at, pages 187–196. Vieweg+Teubner
Verlag, Wiesbaden.
Jermann, P., Soller, A., and Lesgold, A. (2004). Computer
software support for collaborative learning.
Kelley, J. F. (1984). An iterative design methodology for
user-friendly natural language office information ap-
plications. ACM Trans. Inf. Syst., 2(1):26–41.
Kennedy, J., Baxter, P., and Belpaeme, T. (2017). Nonverbal
immediacy as a characterisation of social behaviour
for human–robot interaction. International Journal of
Social Robotics, 9(1):109–128.
Kennedy, J., Lemaignan, S., and Belpaeme, T. (2016). The
cautious attitude of teachers towards social robots in
schools. In Proceedings of the 21th IEEE Interna-
tional Symposium in Robot and Human Interactive
Communication, Workshop on Robots for Learning.
Laal, M. and Ghodsi, S. M. (2012). Benefits of collaborative
learning. Procedia - Social and Behavioral Sciences,
31:486–490.
Lallemand, C., Koenig, V., Gronier, G., and Martin, R.
(2015). Cr
´
eation et validation d’une version franc¸aise
du questionnaire attrakdiff pour l’
´
evaluation de
l’exp
´
erience utilisateur des syst
`
emes interactifs. Re-
vue Europ
´
eenne de Psychologie Appliqu
´
ee/European
Review of Applied Psychology, 65(5):239 – 252.
Mitnik, R., Nussbaum, M., and Soto, A. (2008). An au-
tonomous educational mobile robot mediator. Au-
tonomous Robots, 25(4):367–382.
Mubin, O., Stevens, C. J., Shahid, S., Al Mahmud, A., and
Dong, J.-J. (2013). A review of the applicability of
robots in education. Journal of Technology in Educa-
tion and Learning, 1:209–0015.
Saerbeck, M., Schut, T., Bartneck, C., and Janse, M. D.
(2010). Expressive robots in education: varying the
degree of social supportive behavior of a robotic tu-
tor. In Proceedings of the SIGCHI Conference on
Human Factors in Computing Systems, pages 1613–
1622. ACM.
Sharkey, A. J. C. (2016). Should we welcome robot teach-
ers? Ethics and Information Technology, 18(4):283–
297.
Short, E. and Mataric, M. (2015). Multi-party socially as-
sistive robotics: Defining the moderator role.
Short, E. and Mataric, M. J. (2017). Robot moderation
of a collaborative game: Towards socially assistive
robotics in group interactions. In 2017 26th IEEE In-
ternational Symposium on Robot and Human Interac-
tive Communication (RO-MAN).
Strohkorb, S., Fukuto, E., Warren, N., Taylor, C., Berry, B.,
and Scassellati, B. (2016). Improving human-human
collaboration between children with a social robot. In
Robot and Human Interactive Communication (RO-
MAN), 2016 25th IEEE International Symposium on,
pages 551–556. IEEE.
Tanaka, F., Takahashi, T., Matsuzoe, S., Tazawa, N., and
Morita, M. (2014). Telepresence robot helps children
in communicating with teachers who speak a different
language. In Proceedings of the 2014 ACM/IEEE In-
ternational Conference on Human-robot Interaction,
pages 399–406. ACM Press.
CSEDU 2019 - 11th International Conference on Computer Supported Education
556