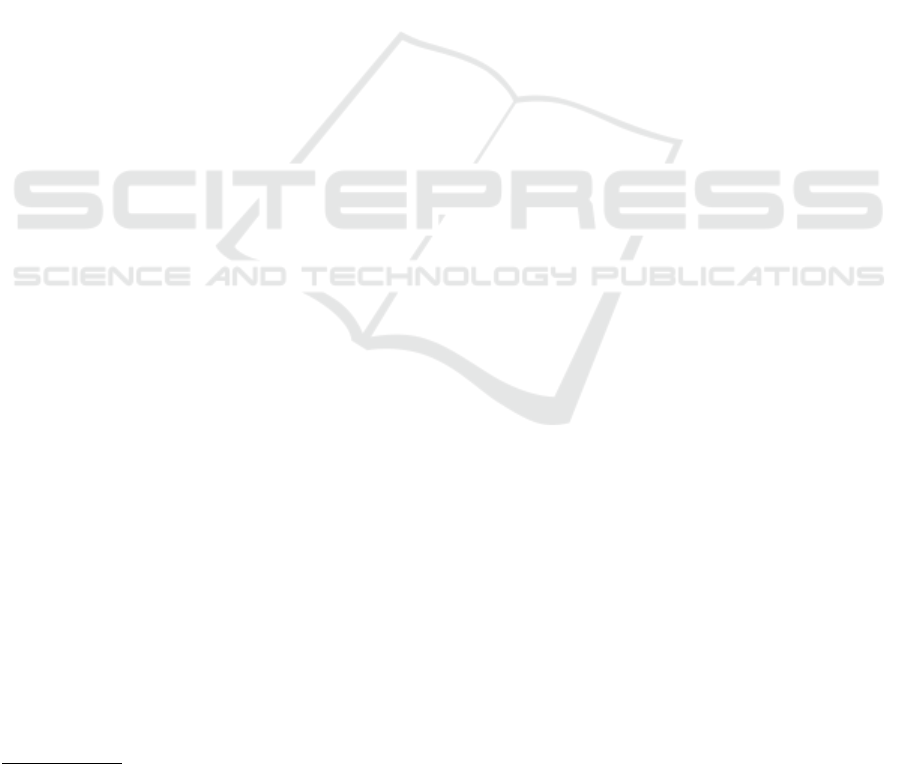
proaches (fuzzy logic reasoning) are a very promising
tool for emotion prediction. However as this approach
depends on rather vague “domain knowledge” with
relationships between physiological data and emo-
tional state (e.g IF eda IS high THEN arousal IS high),
it may not be sufficient to obtain precise arousal or
valence values. Better rules which include features
like skewness of EDA values, SCR latency or HRV,
might be derived from insights obtained by quantita-
tive analysis and/or machine learning – however, for
some learning setting it may be perfectly fine to only
identify rough tendencies in emotional states instead
of fine-grained insights. The required granularity of
the detection certainly depends on the intended use
within the educational software.
The results of the work presented in this paper
directly feed into several industrial learning applica-
tions of LISA project partners that design learning
analytics dashboards incorporating emotional infor-
mation
14
or make use of the emotional state in or-
der to adapt difficulty levels of educational games
15
.
Our next steps include the use of data collected in a
similar study conducted by one of our LISA project
partners
16
, who combined an emotional picture ex-
periment with a cognitive task, using the identical de-
vice to record physiological data (EDA, Heart Rate
and Skin temperature). In parallel to getting more
insight into emotion detection, we will also try to
analyze cognitive states using features from sensor
data. Combining indicators for emotional and cog-
nitive states could be a big step towards our goal of
finding learning indicators.
REFERENCES
Ayata, D. D., Yaslan, Y., and Kamas¸ak, M. (2017). Emotion
recognition via galvanic skin response: Comparison
of machine learning algorithms and feature extraction
methods. Istanbul University-Journal of Electrical &
Electronics Engineering, 17(1):3147–3156.
Boucsein, W. (2012). Electrodermal activity. Springer Sci-
ence & Business Media.
Bradley, M. M. and Lang, P. J. (2007a). The interna-
tional affective picture system (iaps) in the study of
emotion and attention. In Coan, J. A. and Allen, J.
J. B., editors, Handbook of Emotion Elicitation and
Assessment, chapter 29, pages 29–46. Oxford Univer-
sity Press, New York.
Bradley, M. M. and Lang, P. J. (2007b). Motivation
and emotion. In Cacioppo, J., Tssinary, L. G., and
Berntson, G. G., editors, Handbook of psychophysi-
14
Neocosmo, Saarbr
¨
ucken.
15
Serious Games Solutions, Potsdam.
16
Leibniz Institut f
¨
ur Wissensmedien, Tuebingen.
ology, chapter 25, pages 581–607. Oxford University
Press, New York.
Cacioppo, J. T., Berntson, G. G., Larsen, J. T., Poehlmann,
K. M., Ito, T. A., et al. (2000). The psychophysiology
of emotion. Handbook of emotions, 2:173–191.
Camm, A., Malik, M., Bigger, J., Breithardt, G., Cerutti,
S., Cohen, R., Coumel, P., Fallen, E., Kennedy, H.,
Kleiger, R., et al. (1996). Heart rate variability:
standards of measurement, physiological interpreta-
tion and clinical use. task force of the european society
of cardiology and the north american society of pacing
and electrophysiology. Circulation, 93(5):1043–1065.
Chanel, G. and M
¨
uhl, C. (2015). Connecting brains and
bodies: applying physiological computing to sup-
port social interaction. Interacting with Computers,
27(5):534–550.
Conati, C., Chabbal, R., and Maclaren, H. (2018). A
Study on Using Biometric Sensors for Monitoring
User Emotions in Educational Games. Technical re-
port.
Cox, E. (1992). Fuzzy fundamentals. IEEE spectrum,
29(10):58–61.
Ekman, P., Levenson, R. W., and Friesen, W. V. (1983). Au-
tonomic nervous system activity distinguishes among
emotions. Science, 221(4616):1208–1210.
Ferdinando, H., Sepp
¨
anen, T., and Alasaarela, E. (2018).
Emotion recognition using neighborhood components
analysis and ECG/HRV-based features. In Lecture
Notes in Computer Science (including subseries Lec-
ture Notes in Artificial Intelligence and Lecture Notes
in Bioinformatics).
Gruber, J., Mennin, D. S., Fields, A., Purcell, A., and Mur-
ray, G. (2015). Heart rate variability as a potential in-
dicator of positive valence system disturbance: a proof
of concept investigation. International Journal of Psy-
chophysiology, 98(2):240–248.
Heathers, J. and Goodwin, M. (2017). Dead science in live
psychology: A case study from heart rate variability
(hrv).
Kreibig, S. D. (2010). Autonomic nervous system activ-
ity in emotion: A review. Biological psychology,
84(3):394–421.
Lang, P. and Bradley, M. M. (2007). The international af-
fective picture system (iaps) in the study of emotion
and attention. Handbook of emotion elicitation and
assessment, 29.
Lang, P. J. (1995). The emotion probe: studies of motivation
and attention. American psychologist, 50(5):372.
Lang, P. J., Bradley, M. M., and Cuthbert, B. N. (1997). In-
ternational affective picture system (iaps): Technical
manual and affective ratings. NIMH Center for the
Study of Emotion and Attention, pages 39–58.
Lanzetta, J. T., Cartwright-Smith, J., and Eleck, R. E.
(1976). Effects of nonverbal dissimulation on emo-
tional experience and autonomic arousal. Journal of
Personality and Social Psychology, 33(3):354.
Levenson, R. W., Ekman, P., and Friesen, W. V. (1990).
Voluntary facial action generates emotion-specific au-
tonomic nervous system activity. Psychophysiology,
27(4):363–384.
CSEDU 2019 - 11th International Conference on Computer Supported Education
120