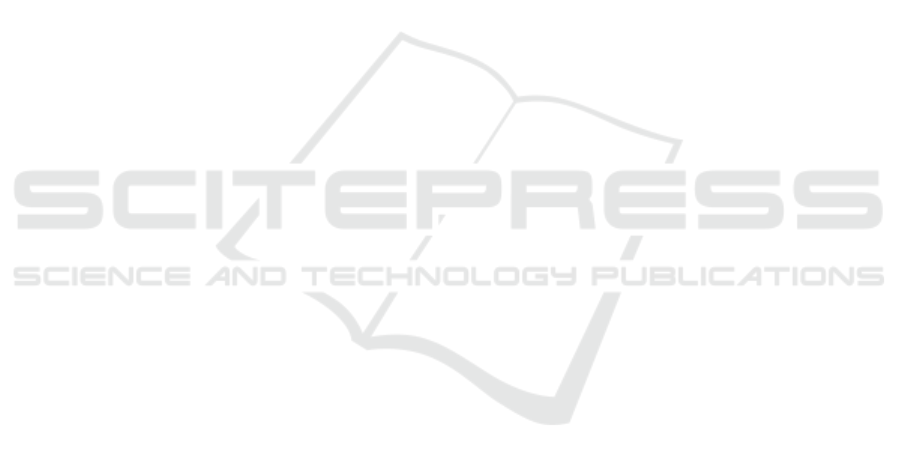
REFERENCES
Arcade, J., Godet, M., Meunier, F., Roubelat, F., 1999.
Structural analysis with the MICMAC method &
Actor's strategy with MACTOR method. Futures
Research Methodology, American Council for the
United Nations University: The Millennium Project, 1-
69.
Attri, R., Grover, S., Dev, N., Kumar, D., 2013. Analysis
of barriers of total productive maintenance (TPM).
International Journal of System Assurance
Engineering & Management, 4(4), 365-377.
Boshier, R., 1971. Motivation Orientation of Adult
Education Participants: A Factor Analytic Exploration
of Houle’s Typify. Adult Education, 21(2), 3-26.
Bouzon, M., Govindan, K., Rodriguez, C.M.T., 2015.
Reducing the extraction of minerals: Reverse logistics
in the machinery manufacturing industry sector in
Brazil using ISM approach. Resources Policy, 46, 27-
36.
Chang, C.C., Wang, C.M., 2008. Influencing Factors of
Teachers’ Integrating IT Technology into Teaching in
Taipei Municipal Vocational High Schools. Journal of
Education Practice and Research, 21(1), 97-132.
Chang, C.S., 2000. The effect of attitudes and self-efficacy
on college student performance in online instruction.
UMI ProQuest Digital Dissertations, AAT 9994597.
Chen, C.M., Liang, J.J., 2009. A Study of Recurrent
Education Students' Perception and Measurement
Instrument upon Self-directed Learning in Higher
Education. Journal of National Taichung University:
Education, 23(2), 205-230.
Chen, C.Y., 2007. A Survey of Weekend Program
Students' Learning Motivation and Satisfaction of
Applied Foreign Languages Department at Meiho
Institute of Technology. Journal of Mei Ho Institute of
Technology, 26(2), 139-160.
Chen, I.H., Lin, H.C., 2018. A Study of Scientific
Research Personnel in the Learning Motivation of the
Academic Ethics Learning on the Research Ethical
Attitude and Learning Satisfaction. Journal
Technology and Human Resource Education, 4(4), 88-
109.
Chen, S.H., Hsieh, C.H., 1999. Ranking generalized fuzzy
number with graded mean integration representation.
Proceedings of the English International Conference
of Fuzzy Sets and Systems Association World
Congress, 2, 551-555.
Deshmukh, S.G., Mandal, A., 1994. Vendor selection
using Interpretive Structural Modelling (ISM).
International Journal of Operations & Production
Management, 14(6), 52-59.
Driscoll, M.P., 1994. Psychology of learning for
instruction. Needham Heights, MA: Allyn & Bacon.
Duperrin, J.C., Godet, M., 1973. Méthode de
hiérarchisation des éléments d'un système: essai de
prospective du système de l'énergie nucléaire dans son
contexte sociétal.
Farris, D.R., Sage, A.P., 1975. On the use of interpretive
structural modeling for worth assessment. Computers
& Electrical Engineering, 2(2-3), 149-174.
Fredricks, J.A., Blumenfeld, P.C., Paris, A.H., 2004.
School engagement: Potential of the concept, state of
the evidence. Review of Educational Research, 74(1),
59-109.
Gardner, R.C., Lambert, W.E., 1972. Attitudes and
Motivation in Second language learning. Newbury
House: Rowley, Mass.
Gendron, B., 2006. Economic analysis of continued
education by holders of short-cycle technical diplomas
in French higher education. European Journal of
Vocational Training,3(39 ), 80-104.
Glanville, J.L., Wildhagen, T., 2007. The Measurement of
School Engagement Assessing Dimensionality and
Measurement Invariance Across Race and Ethnicity.
Educational and Psychological Measurement, 67(6),
1019-1041.
Graff, M., 2003. Cognitive style and attitudes towards
using online learning and assessment methods.
Electronic Journal of e-Learning, 1, 21-28.
Guglielmino, L.M., 1977. Development of the self-directed
learning readiness scale, doctoral dissertation, GA:
University of Georgia.
Handelsman, M.M., Briggs, W.L., Sullivan, N., Towler,
A., 2005. A Measure of College Student Course
Engagement. The Journal of Educational Research,
98(3), 184-191.
Hignite, M.A., 1990. The relationship between computer
attitudes and computer literacy among prospective
business education teachers at missouri's public four-
year colleges and universities. Doctoral Dissertation:
University of Missouri – Columbia.
Hu, H.Y., Shao, I., Chiu, Yen, T.M., 2009. Modified IPA
for Order-Winner Criteria improvement: a MICMAC
approach. Journal of Applied Sciences, 9(21), 3792-
3803.
Huang, H.H., 2003. Research on Learning Attitude
Toward Elective General Courses of Two-Year
College Students-Using Takming College as an
Example. Shih Chien Journal of General
Education, 1, 207-235.
Jia, P., Diabat, A., Mathiyazhagan, K., 2015. Analyzing
the SSCM practices in the mining and mineral
industry by ISM approach. Resources Policy, 46, 76-
85.
Kanungo, S., Duda, S., Srinivas, Y., 1999. A structured
model for evaluating information systems
effectiveness. Behavioral Science, 16(6), 495-518.
Katiyar, R., Barua, M.K., Meena, P.L., 2017. Analysing
the Interactions Among the Barriers of Supplu Chain
Performance Measurement: An ISM with Fuzzy
MICMAC Aprroach. Global Business Review, 19(1),
1-21.
Klir, G.J., Yuan, B., 1995. Fuzzy sets and fuzzy logic:
Theory and Application, Prentice-Hall.
Knowles, M.S., 1975. Self-directed learning: A guild for
leaders and teachers. New York: Cambridge
Association Press.
CSEDU 2019 - 11th International Conference on Computer Supported Education
430