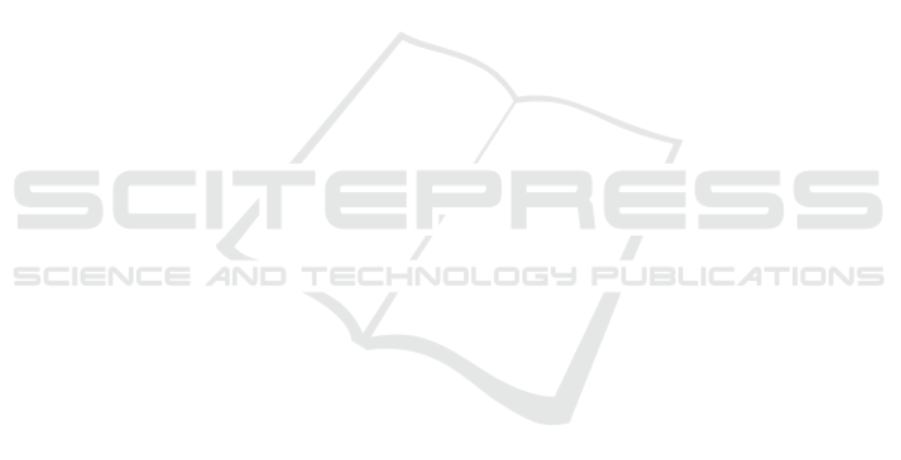
REFERENCES
Azzimonti, M. and Fernandes, M. (2018). Social media net-
works, fake news, and polarization. Technical report,
National Bureau of Economic Research.
Benevenuto, F., Magno, G., Rodrigues, T., and Almeida, V.
(2010). Detecting spammers on twitter. In Collabora-
tion, electronic messaging, anti-abuse and spam con-
ference (CEAS), volume 6, page 12.
Bilge, L., Strufe, T., Balzarotti, D., and Kirda, E. (2009).
All your contacts are belong to us: automated identity
theft attacks on social networks. In Proceedings of
the 18th international conference on World wide web,
pages 551–560. ACM.
Bogers, T. and Van den Bosch, A. (2009). Using language
modeling for spam detection in social reference man-
ager websites. In Proceedings of the 9th Belgian-
Dutch Information Retrieval Workshop (DIR 2009),
pages 87–94.
Braun, P., Cameron, J. J., Cuzzocrea, A., Jiang, F., and Le-
ung, C. K. (2014). Effectively and efficiently mining
frequent patterns from dense graph streams on disk. In
KES, volume 35 of Procedia Computer Science, pages
338–347. Elsevier.
BuzzFeed (2018). https://www.buzzfeed.com/. [Online; ac-
cessed 21-August-2018].
Cucchiarelli, A., D’Antonio, F., and Velardi, P. (2012).
Semantically interconnected social networks. Social
Netw. Analys. Mining, 2(1):69–95.
Das Sarma, A., Das Sarma, A., Gollapudi, S., and Pani-
grahy, R. (2010). Ranking mechanisms in twitter-like
forums. In Proceedings of the third ACM international
conference on Web search and data mining, pages 21–
30. ACM.
Diale, M., Walt, C. V. D., Celik, T., and Modupe, A. (2016).
Feature selection and support vector machine hyper-
parameter optimisation for spam detection. In 2016
Pattern Recognition Association of South Africa and
Robotics and Mechatronics International Conference
(PRASA-RobMech), pages 1–7.
Dormann, C., Demerouti, E., and Bakker, A. (2018). A
model of positive and negative learning: Learning de-
mands and resources, learning engagement, critical
thinking, and face news detection. In Positive learn-
ing in the age of information (PLATO): A blessing or
a curse. Springer International.
Ferrara, E., Varol, O., Davis, C., Menczer, F., and Flammini,
A. (2016). The rise of social bots. Communications of
the ACM, 59(7):96–104.
Friends, S. M. B. O. P. and Profit, R. (2018).
https://www.nytimes.com/2014/11/20/fashion/
social-media-bots-offer-phony-friends-and-real-profit.
html. [Online; accessed 21-August-2018].
Jagatic, T. N., Johnson, N. A., Jakobsson, M., and Menczer,
F. (2007). Social phishing. Communications of the
ACM, 50(10):94–100.
Lee, K., Caverlee, J., and Webb, S. (2010a). Uncovering so-
cial spammers: social honeypots+ machine learning.
In Proceedings of the 33rd international ACM SIGIR
conference on Research and development in informa-
tion retrieval, pages 435–442. ACM.
Lee, K., Eoff, B. D., and Caverlee, J. (2011). Seven months
with the devils: A long-term study of content polluters
on twitter. In ICWSM, pages 185–192.
Lee, S. M., Kim, D. S., Kim, J. H., and Park, J. S. (2010b).
Spam detection using feature selection and parame-
ters optimization. In 2010 International Conference
on Complex, Intelligent and Software Intensive Sys-
tems, pages 883–888.
Li, K., Jiang, H., Yang, L. T., and Cuzzocrea, A., editors
(2015). Big Data - Algorithms, Analytics, and Appli-
cations. Chapman and Hall/CRC.
Li, Z. and Shen, H. (2011). Soap: A social network aided
personalized and effective spam filter to clean your
e-mail box. In INFOCOM, 2011 Proceedings IEEE,
pages 1835–1843. IEEE.
Liu, B., Ni, Z., Luo, J., Cao, J., Ni, X., Liu, B., and Fu,
X. (2018). Analysis of and defense against crowd-
retweeting based spam in social networks. World Wide
Web, pages 1–23.
LiveMint (2018). Bots amplify the spread of fake
news, and harm economies, new research shows.
https://www.livemint.com/Politics/H6usHoKAZUTtU
pM4ZLxHUK/Bots-amplify-the-spread-of-fake-news
-and-harm-economies-ne.html [Online; accessed
21-August-2018].
Nilizadeh, S., Labr
`
eche, F., Sedighian, A., Zand, A., Fer-
nandez, J., Kruegel, C., Stringhini, G., and Vigna, G.
(2017). Poised: Spotting twitter spam off the beaten
paths. In Proceedings of the 2017 ACM SIGSAC Con-
ference on Computer and Communications Security,
pages 1159–1174. ACM.
Noll, M. G., Au Yeung, C.-m., Gibbins, N., Meinel, C., and
Shadbolt, N. (2009). Telling experts from spammers:
expertise ranking in folksonomies. In Proceedings
of the 32nd international ACM SIGIR conference on
Research and development in information retrieval,
pages 612–619. ACM.
Pal, S. K. and Mitra, S. (1992). Multilayer perceptron, fuzzy
sets, classifiaction.
Peters, M. A., Rider, S., Hyv
¨
onen, M., and Besley, T.
(2018). Post-truth, fake news: Viral modernity &
higher education. Springer.
Ratkiewicz, J., Conover, M., Meiss, M. R., Gonc¸alves, B.,
Flammini, A., and Menczer, F. (2011). Detecting
and tracking political abuse in social media. ICWSM,
11:297–304.
Shafahi, M., Kempers, L., and Afsarmanesh, H. (2016).
Phishing through social bots on twitter. In BigData,
pages 3703–3712. IEEE Computer Society.
Shao, C., Ciampaglia, G. L., Varol, O., Yang, K., Flam-
mini, A., and Menczer, F. (2017). The spread of
low-credibility content by social bots. arXiv preprint
arXiv:1707.07592.
Smadi, S., Aslam, N., and Zhang, L. (2018). Detection of
online phishing email using dynamic evolving neural
network based on reinforcement learning. Decision
Support Systems, 107:88–102.
ICEIS 2019 - 21st International Conference on Enterprise Information Systems
310