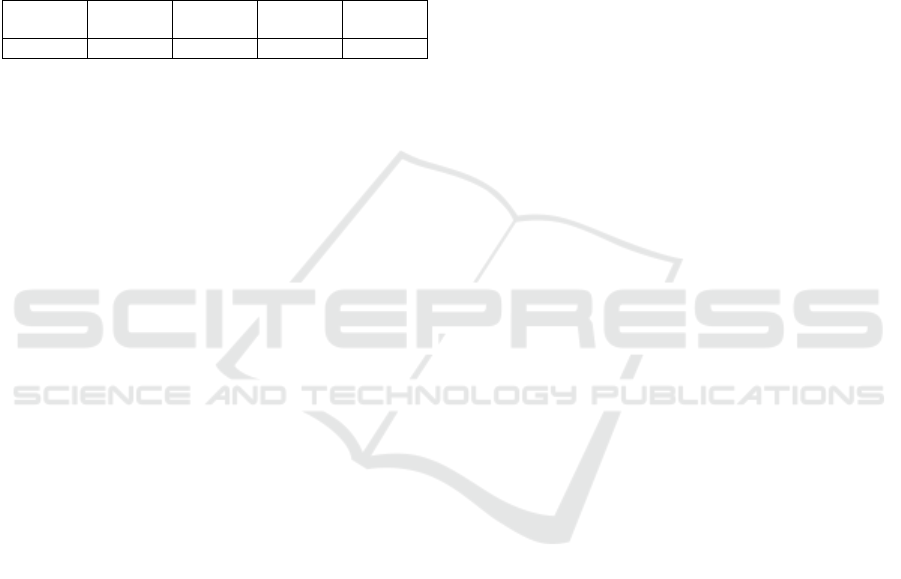
The direct comparison between the two matrices
of the second iteration shows that the object-based
classification is more suitable for the Industrial class
and for the Barley class, while the pixel-based classi-
fication better predicts Water. Naturally, the improve-
ment of both compared to the first level is due pre-
cisely to the incorporation of classes Asphalt and Ur-
ban. It can be said that, in general, the pixel-based
method offers a higher average performance than the
object-based, unless a specific class is only focused.
Table 8: Overall Accuracy Summary of the first and second
iteration.
Land cover maps for both methodologies and iter-
ations 1 and 2 are shown in Figures 5, 6.
A final remark concerns processing time. Object-
based classification takes a few minutes for segmen-
tation on a quad-code personal computer and another
few minutes for training and classification. Pixel-
based needs several hours.
5 CONCLUSIONS
The workflow presented in this study was developed
with the aim of evaluating the potentials obtainable
from the classification of remote sensing images pro-
vided by the Sentinel-2 satellites, in particular that of
creating land covers and use maps. The study area
concerned a neighboring area to the town of Pavia,
Italy. Data for training and accuracy assessment was
personally collected by interviewing farm owners,
observing a very high-resolution satellite image and
with inspection of the areas pertained to as well. The
date May 17
th
2017 was chosen for the study.
As inputs, 10 spectral bands resampled to 10 m
were used. Through ArcGIS Pro (Esri), the pixel-
based and object-based supervised classifications
were applied, using the multiclass SVM algorithm.
The procedures were iterative, to best satisfy the lev-
els of accuracy desired. Thanks to the different bands
available that allow recognizing specific spectral sig-
natures for the objects observed, the multispectral im-
age used has been well suited to the identification of
the different types of coverage present in the area of
interest. It can be said that in general the pixel-based
method offers a better average performance than the
object-based one, unless interested in specific classes.
However, the two methods offer a comparable overall
accuracy. On the other hand, it is also necessary to
take into account the processing time: a few minutes
in the case of object-based classification, several
hours for the pixel-based method. Considering the
overall accuracy results obtained in this study (Table
8), we can conclude that the supervised method is
quite effective for land cover detection.
REFERENCES
Abdi, H., Williams, L. J., 2010. Principal component analy-
sis. In Wiley Interdisciplinary Reviews: Computational
Statistics, vol. 2, no. 4, pp. 433-459.
Chhetri, N., Chaudhary, P., Tiwari, P. R., Yadaw, R. B.,
2012. Institutional and technological innovation: Under-
standing agricultural adaptation to climate change in Ne-
pal. Applied Geography, 33, 142-150.
Comaniciu, D., Meer, P., 2002. Mean shift: A robust ap-
proach toward feature space analysis. IEEE Trans. Pat-
tern Anal. Mach. Intell. , 24, 603–619.
DESA, U., 2017. World population prospects, the 2017 Re-
vision, Volume I: comprehensive tables. New York
United Nations Department of Economic & Social Af-
fairs.
Fukunaga, K., Hostetler, L., 1975. The estimation of the gra-
dient of a density function, with applications in pattern
recognition. IEEE Trans. Inf. Theory, 21, 32–40.
Immitzer, M., Vuolo, F., Atzberger, C., 2016. First Experi-
ence with Sentinel-2 Data for Crop and Tree Species
Classifications in Central Europe, Remote Sensing.
Kowalczyk, A., 2017. Support Vector Machines succinctly,
Succinctly E-book series, Syncfusion Inc.
Marais-Sicre, C., Inglada, J., Fieuzal, R., Baup, F., Valero, S.,
Cros, J., Huc, M., Demarez, V., 2016. Early Detection of
Summer Crops Using High Spatial Resolution Optical
Image Time Series, Remote Sensing.
MiPAAF, 2017. Linee Guida per lo Sviluppo
dell’Agricoltura di precisione in Italia. In Pubblicazioni
Ministero delle Politiche Agricole Alimentari e Forestali
Pierce, F. J., Nowak, P., 1999. Aspects of precision agricul-
ture. In Advances in agronomy.
Schwartz, M. D., 2003. Phenology: An Integrative Environ-
mental Science, Dordrecht: Kluwer Academic Publish-
ers.
Taskin Kaya, G., Musaoglu, N., Ersoy, O. K., 2011. Damage
assessment of 2010 Haiti earthquake with post-earth-
quake satellite image by support vector selection and ad-
aptation. Photogrammetric Engineering & Remote Sens-
ing, 77(10), 1025-1035.
URL-1: http://www.geoportale.regione.lombardia.it
Vapnik, V., 1979. Estimation of Dependences Based on Em-
pirical Data. Nauka, Moscow, pp. 5165–5184, 27 (in
Russian) (English translation: Springer Verlag, New
York, 1982).
Zarco-Tejada, P., Hubbard, N., Loudjani, P., 2014. Precision
Agriculture: An Opportunity for EU Farmers - Potential
Support with the CAP 2014-2020. In Joint Research
Centre (JRC) of the European Commission publication
Mapping Land Cover Types using Sentinel-2 Imagery: A Case Study
249