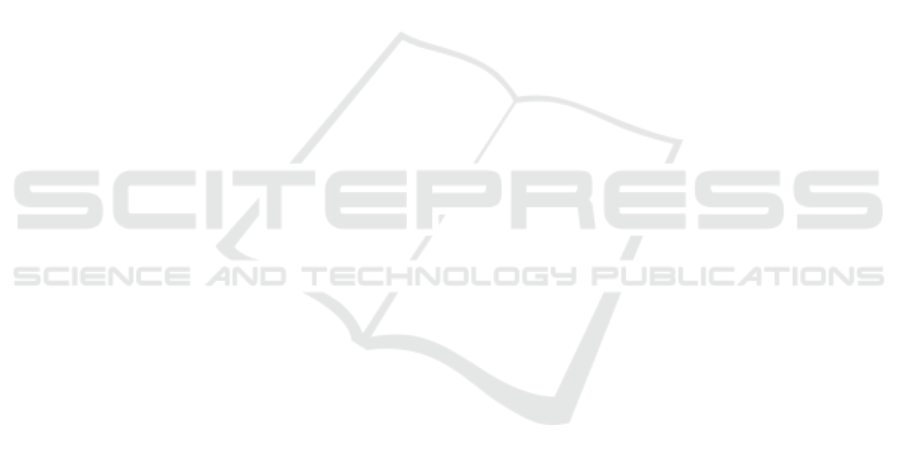
Almishari, M.; Gasti, P.; Tsudik, G. & Oguz, E., 2013.
Privacy-Preserving Matching of Community-
Contributed Content Computer Security - ESORICS
2013: Proceedings of the 18th European Symposium on
Research in Computer Security, Egham, UK, 2013,
Springer, 8134, 443-462.
Anderson, L. A. & Dedrick, R. F., 1990. Development of
the Trust in Physician Scale: A Measure to Assess
Interpersonal Trust in Patient-physician Relationships.
Psychological Reports, Los Angeles, CA, USA, 67(3),
1091-1100.
Bäumer, F. S.; Grote, N.; Kersting, J. & Geierhos, M., 2017.
Privacy Matters: Detecting Nocuous Patient Data
Exposure In Online Physician Reviews. Proceedings of
the 23rd International Conf. on Information and
Software Technologies, Communications in Computer
and Information Science, 756, 77-89, Springer.
Druskininkai, Lithuania.
Bäumer, F. S.; Kersting, J. & Geierhos, M., 2018. Rate
Your Physician: Findings from a Lithuanian Physician
Rating Website. Proceedings of the 24th International
Conf. on Information and Software Technologies,
Communications in Computer and Information
Science, 920, 43-58, Springer, Vilnius, Lithuania.
Bernstam, E. V.; Shelton, D. M.; Walji, M. & Meric-
Bernstam, F., 2005. Instruments to Assess the Quality
of Health Information on the World Wide Web: What
Can Our Patients Actually Use? International J. of
Medical Informatics, 74(1), 13-19.
Chaitin, E.; Stiller, R.; Jacobs, S.; Hershl, J.; Grogen, T. &
Weinberg, J., 2003. Physician-patient Relationship in
the Intensive Care Unit: Erosion of the Sacred Trust?
Critical Care Medicine. Ovid Technologies (Wolters
Kluwer Health), 31(5), 367-372.
Dickerson, S. S. & Brennan, P. F., 2002. The internet as a
catalyst for shifting power in provider-patient
relationships. Nursing Outlook, 50(5), 195-203.
Dugan, E.; Trachtenberg, F. & Hall, M. A., 2005.
Development of abbreviated measures to assess patient
trust in a physician, a health insurer, and the medical
profession. BMC Health Services Research, Springer
Nature, 5(1).
Emmert, M. & Meier, F., 2013. An Analysis of Online
Evaluations on a Physician Rating Website: Evidence
From a German Public Reporting Instrument. Journal
of Medical Internet Research, JMIR Publications Inc.,
15(8), e157.
Emmert, M.; Meier, F.; Pisch, F. & Sander, U., 2013.
Physician Choice Making and Characteristics
Associated With Using Physician-Rating Websites:
Cross-Sectional Study Journal of Medical Internet
Research. JMIR Publications, 15(8), e187.
Eysenbach, G. & Jadad, A. R., 2001. Evidence-based
Patient Choice and Consumer health informatics in the
Internet age Journal of Medical Internet Research.
JMIR Publications Inc., 3(2), e19 .
Filieri, R.; Alguezaui, S. & McLeay, F., 2015. Why do
travelers trust TripAdvisor? Antecedents of trust
towards consumer-generated media and its influence
on recommendation adoption and word of mouth
Tourism Management, 51, 174-185.
Fischer, S. & Emmert, M. Gurtner, S. & Soyez, K. (Eds.),
2015. A Review of Scientific Evidence for Public
Perspectives on Online Rating Websites of Healthcare
Providers Challenges and Opportunities in Health
Care Management, Springer, 279-290.
Gal, T. S.; Chen, Z. & Gangopadhyay, A., 2008. A Privacy
Protection Model for Patient Data with Multiple Sensitive
Attributes. International Journal of Information Security
and Privacy, IGI Global, 2(3), 28-44.
Gao, G. G.; McCullough, J. S.; Agarwal, R. & Jha, A. K.,
2012. A Changing Landscape of Physician Quality
Reporting: Analysis of Patients’ Online Ratings of
Their Physicians Over a 5-Year Period. Journal of
Medical Internet Research, 14.
Geierhos, M., Bäumer, F.S., 2015. Erfahrungsberichte aus
zweiter Hand: Erkenntnisse über die Autorschaft von
Arztbewertungen in Online-Portalen. In: Book of
Abstracts der DHd-Tagung 2015, pp. 69–72, Graz.
Horton, J. & Golden, J., 2015. Reputation Inflation:
Evidence from an Online Labor Market. Working
Paper. New York University.
Huang, F., 2007. Building social trust: A human-capital
approach. Journal of Institutional and Theoretical
Economics, Mohr Siebeck, 163(4), 552-573.
International Organization for Migration. Migracija skaičiais
– EMN, http://123.emn.lt/en, Accessed 2019-02-19.
Jacobson, P., 2007. Empowering the physician-patient
relationship: The effect of the Internet. Partnership:
The Canadian Journal of Library and Information
Practice and Research, 2(1).
Jameda.de. jameda übernimmt mit Patientus deutschen
Marktführer für Videosprechstunde, Accessed 2019-02-
19. German: https://jameda.de/presse/pressemeldungen/
?meldung=165.
Jøsang, A.; Hayward, R. & Pope, S., 2006. Trust network
analysis with subjective logic. Proceedings of the 29th
Australasian Computer Science Conference, 48, 85-94 .
Lenert, L., 2010. Transforming healthcare through patient
empowerment Studies in Health Technology and
Informatics. IOS Press, 153, 159-175.
Lu, T.; Chen, H.; Xu, Y. & Zhang, C., 2016. Internet Usage,
Physician Performances and Patient’s Trust in
Physician During Diagnoses: Investigating Both Pre-
Use and Not-Use Internet Groups. 49th Hawaii
International Conference on System Sciences, HICSS,
Koloa, HI, USA, 3189-3198.
Lu, T.; Xu, Y. C. & Wallace, S., 2018. Internet usage and
patient’s trust in physician during diagnoses: A
knowledge power perspective. Journal of the
Association for Information Science and Technology,
69(1), 110-120.
Luca, M. & Zervas, G., 2016. Fake It Till You Make It:
Reputation, Competition, and Yelp Review Fraud.
Management Science, 62, 3412-3427.
Ma, X.; Ma, J.; Li, H.; Jiang, Q. & Gao, S., 2018. ARMOR:
A trust-based privacy-preserving framework for
decentralized friend recommendation in online social
IoTBDS 2019 - 4th International Conference on Internet of Things, Big Data and Security
154