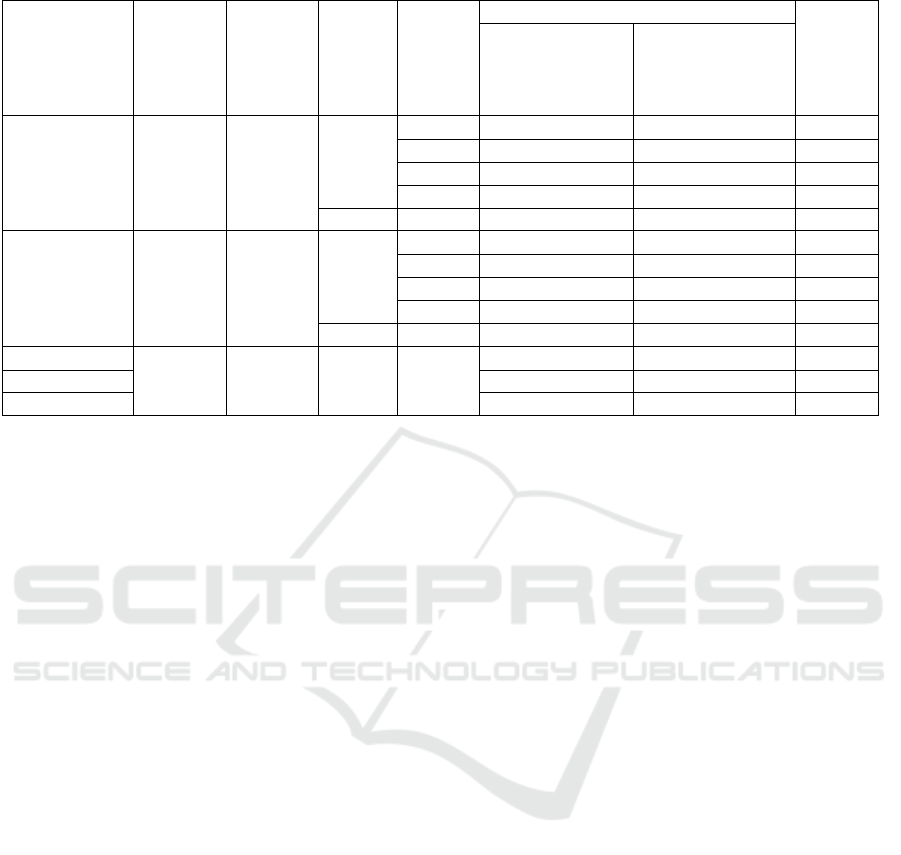
Table 2: Energy consumption for different vehicles and segment lengths.
Energy utilized
Vehicle
Forward
Power
Flow
Efficiency
Reverse
Power
Flow
Efficiency
Average
speed
(m/s)
Segment
length
(m)
Typical trajectory
(kW s)
Optimal trajectory
(kW s)
Energy
saved
Vehicle type 1 0.7 0.2
10
300 305.8 217.7 28.81 %
500 375.4 253.7 32.42 %
1,000 519.7 393.7 24.24 %
3,000 1,144.0 1,073.9 6.13 %
18 3,000 2,043.7 1,643.8 19.57 %
Vehicle type 2 0.7 0.2
10
300 235.4 179.9 23.59 %
500 290.9 203.9 29.91 %
1,000 407.7 314.4 22.88 %
3,000 915.1 853.8 6.7 %
18 3,000 1,695.7 1,392.7 17.87 %
Vehicle type 3
.7 .2 10 300
235.4 167.9 28.67 %
Vehicle type 4 449.2 291.9 35.02 %
Vehicle type 5 234.0 137.6 41.20 %
with the self-driving software in order to prevent ac-
cidents. Even though the proposed speed trajectories
have strong accelerations, the speeds in urban scenar-
ios are generally around 50km/h or less.
REFERENCES
Barth, M., Mandava, S., Boriboonsomsin, K., and Xia, H.
(2011). Dynamic eco-driving for arterial corridors.
In 2011 IEEE Forum on Integrated and Sustainable
Transportation Systems, pages 182–188.
Henriksson, M., Fl
¨
ardh, O., and M
˚
artensson, J. (2017). Op-
timal speed trajectories under variations in the driving
corridor. IFAC-PapersOnLine, 50(1):12551 – 12556.
20th IFAC World Congress.
Hooker, J. (1988). Optimal driving for single-vehicle fuel
economy. Transportation Research Part A: General,
22(3):183 – 201.
Mandava, S., Boriboonsomsin, K., and Barth, M. (2009).
Arterial velocity planning based on traffic signal in-
formation under light traffic conditions. In 2009 12th
International IEEE Conference on Intelligent Trans-
portation Systems, pages 1–6.
Nunzio, G. D., de Wit, C. C., Moulin, P., and Domenico,
D. D. (2013). Eco-driving in urban traffic networks
using traffic signal information. In 52nd IEEE Con-
ference on Decision and Control, pages 892–898.
Qi, X., Wu, G., Hao, P., Boriboonsomsin, K., and Barth,
M. J. (2017). Integrated-connected eco-driving sys-
tem for phevs with co-optimization of vehicle dynam-
ics and powertrain operations. IEEE Transactions on
Intelligent Vehicles, 2(1):2–13.
Schuricht, P., Michler, O., and B
¨
aker, B. (2011). Efficiency-
increasing driver assistance at signalized intersections
using predictive traffic state estimation. In 2011 14th
International IEEE Conference on Intelligent Trans-
portation Systems (ITSC), pages 347–352.
Stern, R. E., Cui, S., Monache, M. L. D., Bhadani, R.,
Bunting, M., Churchill, M., Hamilton, N., Haulcy,
R., Pohlmann, H., Wu, F., Piccoli, B., Seibold, B.,
Sprinkle, J., and Work, D. B. (2018). Dissipation
of stop-and-go waves via control of autonomous ve-
hicles: Field experiments. Transportation Research
Part C: Emerging Technologies, 89:205 – 221.
Yi, Z. and Bauer, P. H. (2017a). Adaptive multiresolu-
tion energy consumption prediction for electric ve-
hicles. IEEE Transactions on Vehicular Technology,
66(11):10515–10525.
Yi, Z. and Bauer, P. H. (2017b). Effects of environmen-
tal factors on electric vehicle energy consumption: a
sensitivity analysis. IET Electrical Systems in Trans-
portation, 7(1):3–13.
Yi, Z. and Bauer, P. H. (2018). Energy aware driving: Op-
timal electric vehicle speed profiles for sustainability
in transportation. IEEE Transactions on Intelligent
Transportation Systems, pages 1–12.
VEHITS 2019 - 5th International Conference on Vehicle Technology and Intelligent Transport Systems
520