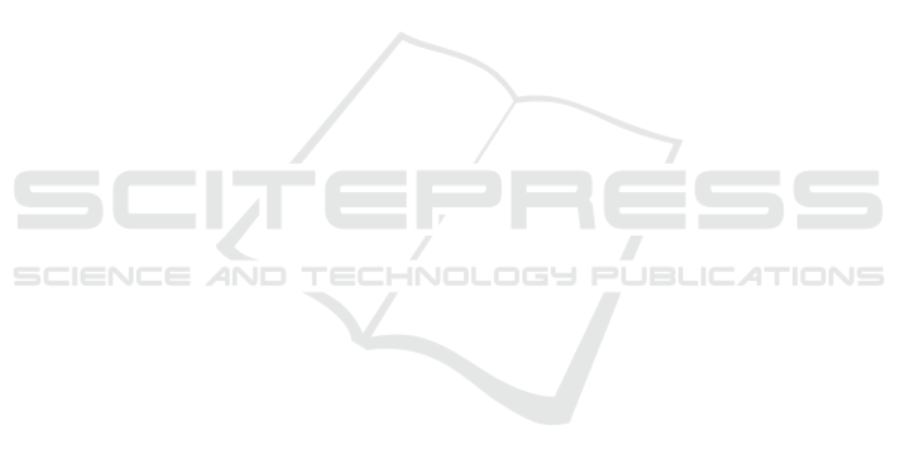
Eq. 10 to take into account the chosen distribution.
Further, a specific perceptual estimator dedicated to
the identification of phase using a feed-forward neu-
ral network was described and implemented. The es-
timator led to a performance in terms of classification
and prediction accuracy that was comparable to that
of the system exploiting the empirical probability dis-
tribution of the phase directly. An advantage of us-
ing a neural network is, that it avoids the potentially
costly computation of the integral in Eq.9 at each inte-
gration step. Future work on phase recognition will be
focus on the identification of state of dynamic system
models tasked with generating phases, e.g. phase-
state machine models (Deimel, 2019). Such mod-
els could provide phase profiles and could be used
in conjunction with a library of ProMPs to control a
task. Furthermore, current research on ProMPs in-
cludes the design of feedback control systems consid-
ering physical interaction with the environment and
the users (Paraschos et al., 2018; Paraschos et al.,
2013). Time scaling does not apply to the control of
arm kinematics and the external contact forces in the
same way as for movements (this holds for each kind
of rescaling, also the linear one). A general frame-
work that can scale kinematics and contact instants in
time should be integrated with a consistent control of
applied forces and torques.
ACKNOWLEDGEMENTS
We gratefully acknowledge financial support for the
project MTI-engAge (16SV7109) by BMBF.
REFERENCES
Avizzano, C. A. and Lippi, V. (2011). A regression model
for digital representation of juggling. In BIO Web of
Conferences, volume 1, page 00006. EDP Sciences.
Colom
´
e, A., Neumann, G., Peters, J., and Torras, C. (2014).
Dimensionality reduction for probabilistic movement
primitives. In Humanoid Robots (Humanoids), 2014
14th IEEE-RAS International Conference on, pages
794–800. IEEE.
Colom
´
e, A. and Torras, C. (2018). Dimensionality reduc-
tion in learning gaussian mixture models of move-
ment primitives for contextualized action selection
and adaptation. IEEE Robotics and Automation Let-
ters, 3(4):3922–3929.
Deimel, R. (2019). A dynamical system for governing con-
tinuous, sequential and reactive behaviors. In Pro-
ceedings of the Austrian Robotics Workshop, page (in
press).
Dermy, O., Charpillet, F., and Ivaldi, S. (2019). Multi-
modal intention prediction with probabilistic move-
ment primitives. In Human Friendly Robotics, pages
181–196. Springer.
Ewerton, M., Neumann, G., Lioutikov, R., Amor, H. B.,
Peters, J., and Maeda, G. (2015). Modeling spatio-
temporal variability in human-robot interaction with
probabilistic movement primitives. In Workshop on
Machine Learning for Social Robotics, ICRA.
Ewerton, M., Rother, D., Weimar, J., Kollegger, G.,
Wiemeyer, J., Peters, J., and Maeda, G. (2018). As-
sisting movement training and execution with visual
and haptic feedback. Frontiers in neurorobotics,
12:24.
Levenberg, K. (1944). A method for the solution of cer-
tain non-linear problems in least squares. Quarterly
of applied mathematics, 2(2):164–168.
Lioutikov, R., Neumann, G., Maeda, G., and Peters,
J. (2017). Learning movement primitive libraries
through probabilistic segmentation. The International
Journal of Robotics Research, 36(8):879–894.
Lippi, V., Avizzano, C. A., and Ruffaldi, E. (2012). A
method for digital representation of human move-
ments. In RO-MAN, 2012 IEEE, pages 404–410.
IEEE.
Maeda, G. J., Neumann, G., Ewerton, M., Lioutikov,
R., Kroemer, O., and Peters, J. (2017). Probabilis-
tic movement primitives for coordination of multi-
ple human–robot collaborative tasks. Autonomous
Robots, 41(3):593–612.
Marquardt, D. W. (1963). An algorithm for least-squares
estimation of nonlinear parameters. Journal of
the society for Industrial and Applied Mathematics,
11(2):431–441.
Oguz, O. S., Sari, O. C., Dinh, K. H., and Wollherr, D.
(2017). Progressive stochastic motion planning for
human-robot interaction. In Robot and Human In-
teractive Communication (RO-MAN), 2017 26th IEEE
International Symposium on, pages 1194–1201. IEEE.
Paraschos, A., Daniel, C., Peters, J., and Neumann, G.
(2018). Using probabilistic movement primitives in
robotics. Autonomous Robots, 42(3):529–551.
Paraschos, A., Daniel, C., Peters, J. R., and Neumann,
G. (2013). Probabilistic movement primitives. In
Advances in neural information processing systems,
pages 2616–2624.
Rueckert, E., Mundo, J., Paraschos, A., Peters, J., and Neu-
mann, G. (2015). Extracting low-dimensional control
variables for movement primitives. In Robotics and
Automation (ICRA), 2015 IEEE International Confer-
ence on, pages 1511–1518. IEEE.
Zhang, X. and Chaffin, D. B. (1999). The effects of
speed variation on joint kinematics during multiseg-
ment reaching movements. human movement science,
18(6):741–757.
ICINCO 2019 - 16th International Conference on Informatics in Control, Automation and Robotics
578