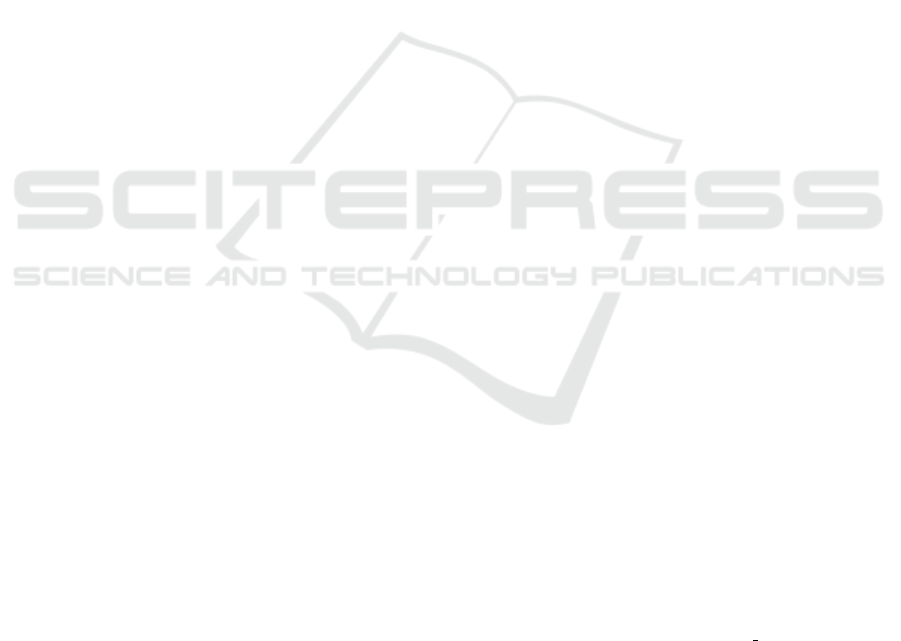
REFERENCES
Bahl, P. and Padmanabhan, V. N. (2000). Radar: An in-
building RF-based user location and tracking system.
In Proc. IEEE INFOCOM 2000, volume 2, pages
775–784.
Battiti, R., Thang Le, N., and Villani, A. (2002). Location-
aware computing: A neural network model for de-
termining location in wireless LANs. Int. Semicond.
Conf., 4.
Bocicor, M. I. et al. (2017). Cyber-physical system for as-
sisted living and home monitoring. In 13th IEEE In-
ternational Conference on Intelligent Computer Com-
munication and Processing, pages 487–493.
Bulten, W., Rossum, A. C. V., and Haselager, W. F. G.
(2016). Human slam, indoor localisation of devices
and users. In IEEE First International Conference on
Internet-of-Things Design and Implementation.
Deng, Z. A., Hu, Y., Yu, J., and Na, Z. (2015). Extended
kalman filter for real time indoor localization by fus-
ing wifi and smartphone inertial sensors. Microma-
chines, 6:523–543.
Dong, Q. and Dargie, W. (2012). Evaluation of the reliabil-
ity of RSSI for indoor localization. In Proceedings of
the IEEE International Conference on Wireless Com-
munications in Unusual and Confined Areas.
Fariz, N. et al. (2018). An improved indoor location tech-
nique using kalman filter. International Journal of En-
gineering & Technology, 7.
Haigh, P. A. et al. (2014). Visible light communications:
real time 10 mb/s link with a low bandwidth poly-
mer light-emitting diode. Optics express, 22(3):2830–
2838.
Kingma, D. P. and Ba, J. (2014). Adam: A method for
stochastic optimization. CoRR, abs/1412.6980.
Li, G. et al. (2018). Indoor positioning algorithm based on
the improved RSSI distance model. Sensors, 18(9).
Lukito, Y. and Chrismanto, A. R. (2017). Recurrent neu-
ral networks model for WiFi-based indoor positioning
system. In 2017 International Conference on Smart
Cities, Automation & Intelligent Computing Systems
(ICON-SONICS).
Luo, X., O’Brien, W. J., and Julien, C. (2011). Comparative
evaluation of received signal strength index (RSSI)-
based indoor localization techniques for construction
jobsites. Adv. Eng. Inform., 25:355–363.
Lymberopoulos, D. et al. (2015). A realistic evaluation and
comparison of indoor location technologies: Experi-
ences and lessons learned. In The 14th ACM/IEEE
Conference on Information Processing in Sensor Net-
works (IPSN ’15).
Maduskar, D. and Tapaswi, S. (2017). RSSI based adap-
tive indoor location tracker. Scientific Phone Apps and
Mobile Devices, 3.
Marin, I. et al. (2018). i-Light - Intelligent Luminaire Based
Platform for Home Monitoring and Assisted Living.
Electronics, 7(220).
Mittal, A., Tiku, S., and Pasricha, S. (2018). Adapting Con-
volutional Neural Networks for Indoor Localization
with Smart Mobile Devices. In Proceedings of the
2018 on Great Lakes Symposium on VLSI.
Nair, V. and Hinton, G. E. (2010). Rectified linear units im-
prove restricted boltzmann machines. In Proceedings
of the 27th International Conference on International
Conference on Machine Learning, ICML’10, pages
807–814, USA. Omnipress.
Ozsoy, K., Bozkurt, A., and Tekin, I. (2013). Indoor po-
sitioning based on global positioning system signals.
Microwave and Optical Technology Letters, 55:1091–
1097.
Pu, C. C., Pu, C.-H., and Lee, H.-J. (2011). Indoor location
tracking using received signal strength indicator. In
Emerging Communications for Wireless Sensor Net-
works.
Robesaat, J., Zhang, P., Abdelaal, M., and Theel, O. (2017).
An improved ble indoor localization with kalman-
based fusion: An experimental study. Sensors, 17.
Sadowski, S. and Spachos, P. (2018). RSSI-based indoor
localization with the internet of things. In IEEE Ac-
cess.
Sung, Y. (2016). RSSI-based distance estimation frame-
work using a kalman filter for sustainable indoorcom-
puting environments. Sustainability, 8.
Ta, V.-C. (2017). Smartphone-based indoor positioning
using Wi-Fi, inertial sensors and Bluetooth. https://
tel.archives-ouvertes.fr/tel-01883828/document. Ma-
chine Learning, Universit
´
e Grenoble Alpes.
Tarrio, P. et al. (2011). An energy-efficient strategy for
combined rss-pdr indoor localization. In IEEE In-
ternational Conference on Pervasive Computing and
Communications Workshops (PERCOM Workshops),
pages 619–624.
Tarzia, S. P. et al. (2011). Indoor localization without in-
frastructure using the acoustic background spectrum.
In Proceedings of the 9th international conference on
Mobile systems, applications, and services, page 155.
Wang, J.-Y. et al. (2012). High-precision RSSI-based in-
door localization using a transmission power adjust-
ment strategy for wireless sensor networks. In 2012
IEEE 14th International Conference on High Perfor-
mance Computing and Communication, 2012 IEEE
9th International Conference on Embedded Software
and Systems.
Welch, G. and Bishop, G. (1995). An introduction to the
kalman filter. Technical report, Department of Com-
puter Science, University of North Carolina at Chapel
Hill, Chapel Hill, NC, USA.
World Health Organization (2015). World report on age-
ing and health. http://apps.who.int/iris/bitstream/
10665/186463/1/9789240694811 eng.pdf?ua=1.
Xiao, J. and Zhou, Z. (2016). A survey on wireless indoor
localization from the device perspective. ACM Com-
puting Surveys, 49.
Zhanga, W., Liua, K., Zhang, W., Zhang, Y., and Gu, J.
(2016). Deep neural networks for wireless localiza-
tion in indoor and outdoor environments. Neurocom-
puting, 194:279–287.
Indoor Localisation with Intelligent Luminaires for Home Monitoring
471