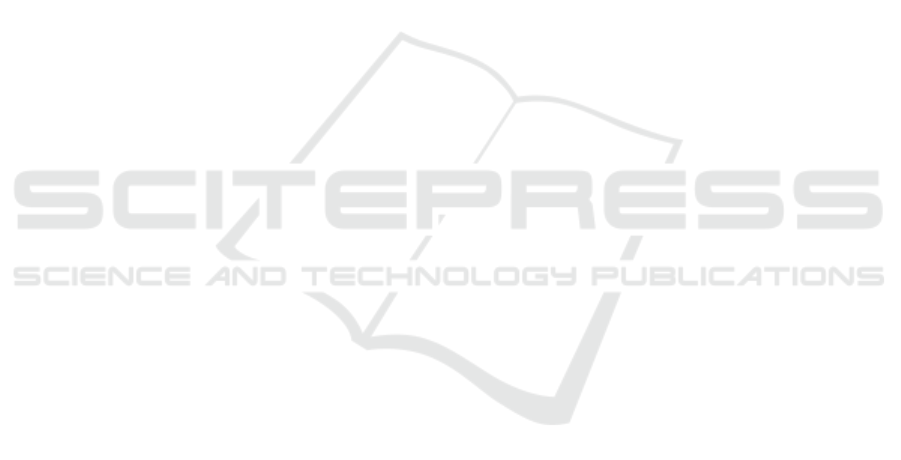
the network and by cooperation we mean data shar-
ing. To do so, MA will carry transactions that con-
tains the data and aggregate the data. Furthermore,
by dispatching MA, we reduce the amount of sensory
data by eliminating redundancy. For example, nodes
that are placed in proximity of each other are likely to
generate redundant data. Ultimately, data aggregation
is required to reduce the data traffic in the network.
Second Layer, comprise of a less unconstrained
devices running the IOTA full Node. Finally, the ap-
plication layer utilize from the proposed approach in
terms of energy efficiency, scalability, and privacy. To
this end, a number of questions should be tackled in
the future: How is data offloaded? What kind of prim-
itive mode will be used? How is the PoW outsourced
to IOTA full Node? How MA will be routed between
nodes in an energy efficient manner?
5 CONCLUSIONS
IOTA Tangle architecture has the potential of enabling
distributed intelligence in IoT, which will be benefi-
cial for a wide-range of applications, such as smart
cities, and healthcare. IOTA Tangle allows for dis-
tributed computation, making it suitable for enabling
distributed intelligence in IoT. In this article, we have
discussed the need for distributed intelligence in IoT
as well as how the IOTA platform can be utilized to
enable it. We have presented a review of the recent
state of the art distributed intelligence approaches in
IoT. Also, we have discovered that there is a need for
a a lightweight solution for enabling distributed intel-
ligence based on the identified limitations of existing
approaches. We have discussed the challenges as well
as future research directions in developing a new dis-
tributed intelligence approach and we believe that the
integration of IOTA and mobile agent would solve the
problems. Finally, we outline pathways to solutions
to the problems identified and envision that an IOTA
Tangle architecture will facilitate distributed intelli-
gence in IoT.
In the authors opinion, MA migrates between
nodes in the network and facilitates cooperation be-
tween them. This leads to several advantages, such as
reduction in the network load by moving MA carrying
data instead of sending data to a central location for
further processing, and overcomes network latency.
REFERENCES
Alsboui, T., Alrifaee, M., Etaywi, R., and Jawad, M. A.
(2017). Mobile agent itinerary planning approaches in
wireless sensor networks- state of the art and current
challenges. In Maglaras, L. A., Janicke, H., and Jones,
K., editors, Industrial Networks and Intelligent Sys-
tems, pages 143–153, Cham. Springer International
Publishing.
Angelis, E. D., Ciribini, A., Tagliabue, L., and Paneroni,
M. (2015). The brescia smart campus demonstrator.
renovation toward a zero energy classroom building.
Procedia Engineering, 118:735–743.
Ashton, K. (2009). That ’Internet of Things’ Thing. RFID
Journal.
Atzori, L., Iera, A., and Morabito, G. (2010). The internet of
things: A survey. Computer Networks, 54(15):2787–
2805.
Bondi, A. B. (2000). Characteristics of scalability and their
impact on performance. In Workshop on Software and
Performance, pages 195–203.
Byers, C. C. and Wetterwald, P. (2015). Fog computing dis-
tributing data and intelligence for resiliency and scale
necessary for iot: The internet of things (ubiquity
symposium). Ubiquity, 2015(November):4:1–4:12.
Doan, T. T., Safavi-Naini, R., Li, S., Avizheh, S., K., M. V.,
and Fong, P. W. L. (2018). Towards a resilient smart
home. In Proceedings of the 2018 Workshop on IoT
Security and Privacy, IoT S&P ’18, pages 15–21,
New York, NY, USA. ACM.
Esposito, C., Castiglione, A., Pop, F., and Choo, K. R.
(2017). Challenges of connecting edge and cloud
computing: A security and forensic perspective. IEEE
Cloud Computing, 4(2):13–17.
Foundation, I. (2016a). Iota development roadmap. (1).
(visited on 2-01-2019).
Foundation, I. (2016b). The qubic protocol. (1). (visited on
2-1-2019).
Gartner (2013). Gartner says the internet of things installed
base will grow to 26 billion units by 2020. (1).
Gillam, L., Katsaros, K., Dianati, M., and Mouzakitis,
A. (2018). Exploring edges for connected and au-
tonomous driving. In IEEE INFOCOM 2018 - IEEE
Conference on Computer Communications Workshops
(INFOCOM WKSHPS), pages 148–153.
Handy, P. (2017). Introducing masked authenticated mes-
saging. (1). (visited on 6-01-2019).
Lange, D. B. and Oshima, M. (1999). Seven good reasons
for mobile agents. Commun. ACM, 42(3):88–89.
Lepp
¨
anen, T., Riekki, J., Liu, M., Harjula, E., and Ojala,
T. (2014). Mobile Agents-Based Smart Objects for
the Internet of Things, pages 29–48. Springer Inter-
national Publishing, Cham.
Lynne, P. (2007). Distributed intelligence: Overview of the
field and its application in multi-robot systems. In The
AAAI Fall Symposium Series. AAAI Digital Library.
Nakamoto, S. et al. (2008). Bitcoin: A peer-to-peer elec-
tronic cash system.
Perera, C., Qin, Y., Estrella, J. C., Reiff-Marganiec, S., and
Vasilakos, A. V. (2017). Fog computing for sustain-
able smart cities: A survey. ACM Comput. Surv.,
50(3):32:1–32:43.
Rahman, H. and Rahmani, R. (2018). Enabling distributed
intelligence assisted future internet of things con-
Enabling Distributed Intelligence in the Internet of Things using the IOTA Tangle Architecture
397