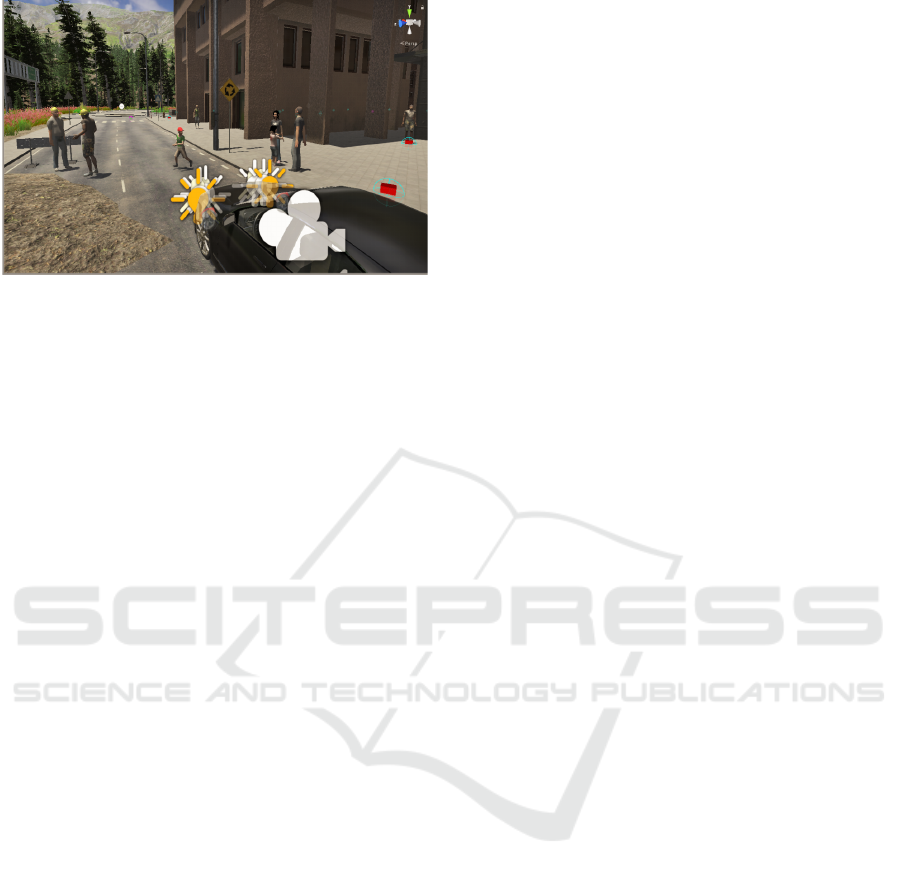
Figure 2: Example of high mindreading event.
can either use automatic control, trusting traffic rules,
or shift to inferring the intentions of other road users,
independently from rule following, by mentalizing.
There are situations designed to forse mentalization,
as in Fig. 2, when suddenly a child crosses the street
without looking at the incoming vehicle.
REFERENCES
Bengtsson, P. (2018). Attuning the pedestrian-vehicle and
driver-vehicle – why attributing a mind to a vehicle
matters. In Karwowski, W. and Ahram, T., editors, In-
ternational Conference on Intelligent Human Systems
Integration, pages 308–319.
Bojarski, M., Yeres, P., Choromanaska, A., Choromanski,
K., Firner, B., Jackel, L., and Muller, U. (2017). Ex-
plaining how a deep neural network trained with end-
to-end learning steers a car. CoRR, abs/1704.07911.
Brooks, R. and Meltzoff, A. N. (2002). The importance of
eyes: how infants interpret adult looking behaviour.
Developmental Psychology, 38:958–966.
Broth, M., Cromdal, J., and Levin, L. (2018). Telling the
other’s side. formulating others’ mental states in driver
training. Language & Communication.
Call, J. and Tomasello, M. (2005). What chimpanzee
know about seeing, revisited: An explanation of the
third kind. In Eilian, N., Hoerl, C., McCormack, T.,
and Roessler, J., editors, Joint Attention: communica-
tion and other minds, pages 45–64. Oxford University
Press, Oxford (UK).
Chui, M., Manyika, J., Miremadi, M., Henke, N., Chung,
R., Nel, P., and Malhotra, S. (2018). Notes from the AI
frontier: insights from hundreds of use cases. Techni-
cal Report April, McKinsey Global Institute.
Dennett, D. C. (1987). The Intentional Stance. MIT Press,
Cambridge (MA).
Gallese, V. and Goldman, A. (1998). Mirror neurons and the
simulation theory of mind-reading. Trends in Cogni-
tive Sciences, 2:493–501.
Goffman, E. (1963). Behavior in public places: Notes on
the social organisation of gatherings. Free Press, New
York.
Goldman, A. (2006). Simulating Minds: The Philosophy,
Psychology, and Neuroscience of Mindreading. Ox-
ford University Press, Oxford (UK).
Goodfellow, I., Bengio, Y., and Courville, A. (2016). Deep
Learning. MIT Press, Cambridge (MA).
Gopnik, A. and Meltzoff, A. N. (1997). Words, Thoughts
and Theories. Oxford University Press, Oxford (UK).
Gopnik, A. and Meltzoff, A. N. (1999). The Scientist in the
Crib. HarperCollins, London.
Gopnik, A. and Wellman, H. M. (2012). Reconstruct-
ing constructivism: Causal models, bayesian learn-
ing mechanisms, and the theory-theory. Psychological
Bulletin, 138:1085–1108.
Gurghian, A., Koduri, T., Bailur, S. V., Carey, K. J., and
Murali, V. N. (2016). DeepLanes: End-to-end lane
position estimation using deep neural networks. In
Proc. of IEEE International Conference on Computer
Vision and Pattern Recognition, pages 38–45.
Haddington, P. and Rauniomaa, M. (2014). Interaction be-
tween road users: Offering space in traffic. Space and
Culture, 17:176–190.
Hazelwood, K., Bird, S., Brooks, D., Chintala, S., Diril, U.,
Dzhulgakov, D., Fawzy, M., Jia, B., Jia, Y., Kalro, A.,
Law, J., Lee, K., Lu, J., Noordhuis, P., Smelyanskiy,
M., Xiong, L., and Wang, X. (2018). Applied machine
learning at Facebook: A datacenter infrastructure per-
spective. In IEEE International Symposium on High
Performance Computer Architecture (HPCA), pages
620–629.
Heider, F. (1958). The Psychology of Interpersonal Rela-
tions. John Wiley, New York.
Hesslow, G. (2012). The current status of the simulation
theory of cognition. Brain, 1428:71–79.
Hutto, D. D. (2008). Folk Psychological Narratives: The
Sociocultural Basis of Understanding Reasons. MIT
Press, Cambridge (MA).
Jensen, O. B. (2010). Negotiation in motion: Unpacking a
geography of mobility. Space and Culture, 13:389–-
402.
Johnson, C. (2017). Readiness of the road network for con-
nected and autonomous vehicles.
Jorge, C. C. and Rossetti, R. J. F. (2018). On social interac-
tions and the emergence of autonomous vehicles. In
International Conference on Vehicle Technology and
Intelligent Transport Systems, pages 423–430.
Karndacharuk, A., Wilson, D. J., and Dunn, R. (2014). A re-
view of the evolution of shared (street) space concepts
in urban environments. Transport reviews, 34:190–
220.
Kircher, K. and Ahlstrom, C. (2017). Minimum required
attention: A human-centered approach to driver inat-
tention. Human factors, 59:471–484.
Laurier, E. (2018). Civility and mobility: Drivers (and pas-
sengers) appreciating the actions of other drivers. Lan-
guage & Communication.
Li, J., Cheng, H., Guo, H., and Qiu, S. (2018). Survey on
artificial intelligence for vehicles. Automotive Innova-
tion, 1:2–14.
VEHITS 2019 - 5th International Conference on Vehicle Technology and Intelligent Transport Systems
540