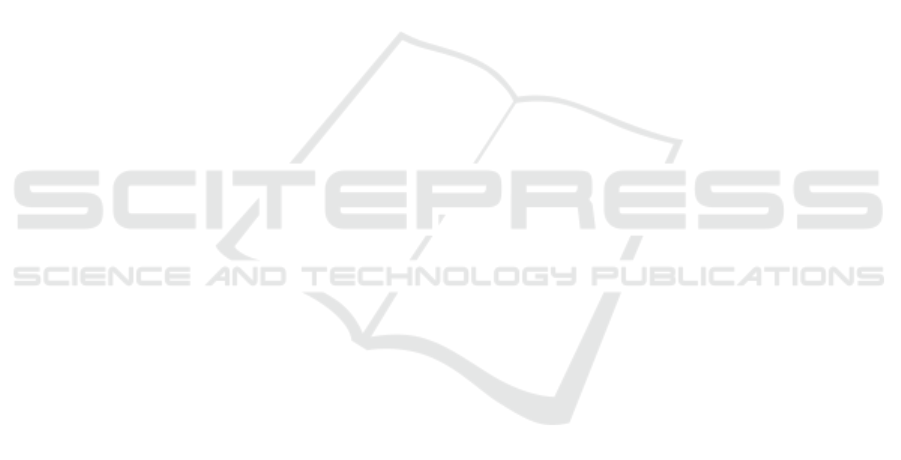
timization outcome can be done using any notion of
social welfare, as all the social welfare methods se-
lect the same outcome where all the CGGs are able to
meet their demand requirements without making any
compromise over production.
In constrained resource settings, we deduce some
facts about the selection of social welfare method with
respect to plant growth in CGGs. First, the inequality
based SWO can be applied to select the final outcome
specifically for long-day photoperiodic plants which
are sensitive to the duration of day-night like rud-
beckia and california poppy. That is to ensure at least
one of the CGGs gets the required amount of light,
instead of making all the CGGs affected by the con-
strained resources, as seen in figure 4a. Second, over-
all utility based SWOs can be used to select the allo-
cation for day-neutral plants which do not require any
specific day length like rose and tomato growing. This
is because all these methods take into account overall
well-being of CGGs (see figure 4c). The CGG, which
takes the consequences of constrained resources will
not be affected too much due to their day-neutral sen-
sitivity. Third, for short-day plants like chrysanthe-
mum, christmas cactus, and poinsettia, it is recom-
mended to use equality based SWOs (figure 4b). This
is because they do not require long lighting hours. In
case of insufficient resources, CGGs can easily make
a fair compromise with respect to their demand pref-
erence without affecting plant growth (Kumpf, 2019).
6 CONCLUSION
This paper discusses the properties and impact of dif-
ferent notions of social welfare on an optimization
outcome in MOMI optimization problems. In this
regard, a GA based MOMI optimization approach is
used to describe a complex, real world problem. To
study the impact of social welfare methods, two sets
of experiments are conducted in unconstrained and
constrained resource settings respectively. The results
show that different notions of social welfare lead to
different optimization outcomes and selection of the
social welfare method depends on the behavior we ex-
pect from the system.
In future works, we intend to investigate the im-
pact of social welfare methods on different real-
world problems which exhibit additional complexity
in terms of number of objectives. We aim to focus
on 1) combining different notions of social welfare
to select the final optimization outcome, i.e., in cases
where multiple contracts yield same utilitarian score,
equality based SWOs can be applied to select the most
fair contract out of the resulting set of contracts yield-
ing high utilitarian score, 2) applying different notion
of social welfare at each level of RIG, i.e., in cases
where each level of RIG comprises more than one
concern.
REFERENCES
Chevaleyre, Y., Dunne, P. E., Endriss, U., Lang, J.,
Lemaitre, M., Maudet, N., Padget, J., Phelps, S.,
Rodriguez-Aguilar, J. A., and Sousa, P. (2006). Is-
sues in multiagent resource allocation. Informatica,
30(1).
Clausen, A., Demazeau, Y., and Jørgensen, B. N. (2014).
An agent-based framework for aggregation of man-
ageable distributed energy resources. In International
Conference on PAAMS, pages 214–225. Springer.
Darmann, A. and Schauer, J. (2015). Maximizing
nash product social welfare in allocating indivisible
goods. European Journal of Operational Research,
247(2):548–559.
Finkelstein, A., Harman, M., Mansouri, S. A., Ren, J., and
Zhang, Y. (2009). A search based approach to fair-
ness analysis in requirement assignments to aid nego-
tiation, mediation and decision making. Requirements
Engineering, 14(4):231–245.
Fujita, K., Ito, T., and Klein, M. (2012). A secure and fair
protocol that addresses weaknesses of the nash bar-
gaining solution in nonlinear negotiation. Group De-
cision and Negotiation, 21(1):29–47.
Huaizhou, S., Prasad, R. V., Onur, E., and Niemegeers, I.
(2014). Fairness in wireless networks: Issues, mea-
sures and challenges. IEEE Communications Surveys
& Tutorials, 16(1):5–24.
Kumpf, J. (2019). Lighting systems. [Online; accessed on
4-January-2019].
Li, M., Vo, Q. B., and Kowalczyk, R. (2009). Searching
for fair joint gains in agent-based negotiation. In Pro-
ceedings of The 8th International Conference on Au-
tonomous Agents and Multiagent Systems-Volume 2,
pages 1049–1056.
Liu, Y., Yuen, C., Huang, S., Hassan, N. U., Wang, X.,
and Xie, S. (2014). Peak-to-average ratio constrained
demand-side management with consumer’s prefer-
ence in residential smart grid. IEEE Journal of Se-
lected Topics in Signal Processing, 8(6):1084–1097.
Shannon, C. E. (2001). A mathematical theory of commu-
nication. ACM SIGMOBILE, 5(1):3–55.
Sørensen, J. C. and Jørgensen, B. N. (2017). An extensi-
ble component-based multi-objective evolutionary al-
gorithm framework. In Proceedings of the 6th ICSCA,
pages 191–197. ACM.
Umair, A. (2018). An Agent Based Approach to Co-
ordination of Resource Allocation and Process Per-
formance. PhD thesis, Syddansk Universitet. Det
Tekniske Fakultet.
SMARTGREENS 2019 - 8th International Conference on Smart Cities and Green ICT Systems
186