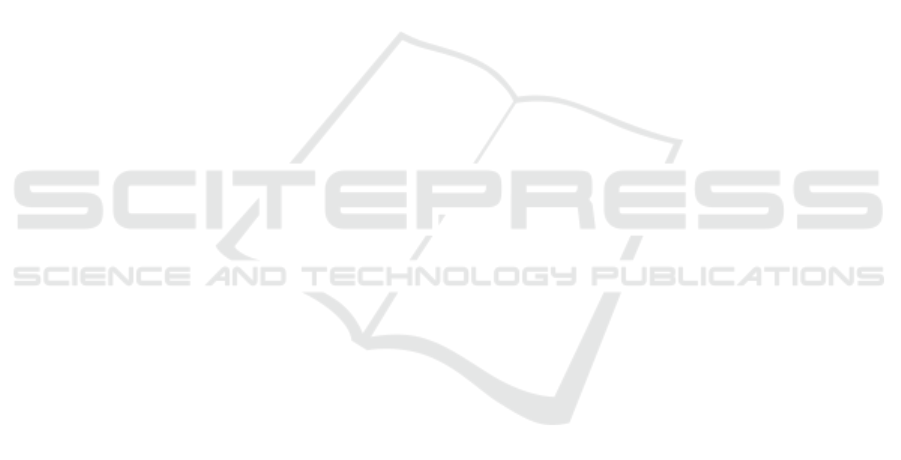
In a short term, we expect this tool be officially in-
corporated into NMCP’s portfolio and become a ref-
erence platform for malaria research. This will re-
quire the setup of a cloud-based data as a service so-
lution in conformance to performance, scalability, re-
liability and availability requisites.
As middle term goal, we aim to keep all databases
updated and to design a ”real time” data capture sys-
tem allowing users to provide information on sus-
pected cases, hot spots and any other useful data on
a daily basis. This will allow for better decision and
prompt reaction in suspect situations. We are running
a pilot study on real time data capture and alert sys-
tem in Manaus, with support of local health agents
and technical staff from the Amazonas State Founda-
tion for Health Surveillance (FVS-AM).
The proposed tool has been also used to support
research on i) visual mining/analytics and ii) fore-
casting models. The set of visual metaphors provided
by the tool has been designed having in mind the di-
versity of potential users (government staff, research,
general public) and the most useful and effective re-
sources they can use to answer their decision-making
or research queries. Regarding forecasting, this work
aimed at to verify the predictive capacity of some
machine learning algorithms over malaria data from
Brazil. The next steps comprise the addition of new
attributes to improve long-term predictive power and
comparison with other metrics and models, including
neural networks and autoregressive ones.
REFERENCES
Agrawal, R. K. and Ratnadip, A. K. (2013). An introduc-
tory study on time series modeling and forecasting.
arXiv:1302.6613, 1302.6613:1–68.
Barreto, M., Alves, A., Sena, S., Fiaccone, R., Amorim, L.,
Ichihara, M. Y., and Barreto, M. (2017). Assessing the
accuracy of probabilistic record linkage of social and
health databases in the 100 million brazilian cohort. In
Proceedings of the IPDLN Conference (August 2016).
Swansea University.
Ben Taieb, S. and Hyndman, R. J. (2014). Recursive and
direct multi-step forecasting: the best of both worlds.
International Journal of Forecasting, (September).
Carvajal, T. M., Viacrusis, K. M., Hernandez, L. F. T.,
Ho, H. T., Amalin, D. M., and Watanabe, K. (2018).
Machine learning methods reveal the temporal pat-
tern of dengue incidence using meteorological factors
in metropolitan Manila, Philippines. BMC Infectious
Diseases, 18(1):1–15.
Ch, S., Sohani, S. K., Kumar, D., Malik, A., Chahar, B. R.,
Nema, A. K., Panigrahi, B. K., and Dhiman, R. C.
(2014). A support vector machine-firefly algorithm
based forecasting model to determine malaria trans-
mission. Neurocomput., 129:279–288.
Hay, S. I. and Snow, R. W. (2006). The Malaria Atlas
Project: developing global maps of malaria risk. PLoS
medicine, 3(12):e473.
Hyndman, R. and Athanasopoulos, G. (2018). Forecasting:
Principles and Practice. OTexts, Australia, 2nd edi-
tion.
Kane, M. J., Price, N., Scotch, M., and Rabinowitz, P.
(2014). Comparison of ARIMA and Random Forest
time series models for prediction of avian influenza
H5N1 outbreaks. BMC Bioinformatics, 15(1):276.
Kouway
`
e, B. (2016). Regression Trees and Random for-
est based feature selection for malaria risk exposure
prediction. pages 1–15.
Kuhn, M. (2009). The caret package.
Lorenz, C., Virginio, F., Aguiar, B. S., Suesdek, L., and
Chiaravalloti-Neto, F. (2015). Spatial and temporal
epidemiology of malaria in extra-amazonian regions
of brazil. Malaria Journal, 14(1):408.
Modu, B., Polovina, N., Lan, Y., Konur, S., Asyhari, T., and
Peng, Y. (2017). Towards a predictive analytics-based
intelligent malaria outbreak warning system. Applied
Sciences, 7:836.
Pita, R., Pinto, C. P., Sena, S., Fiaccone, R., Amorim, L.,
Reis, S., Barreto, M. L., Denaxas, S., and Barreto,
M. E. (2018). On the accuracy and scalability of prob-
abilistic data linkage over the Brazilian 114 million
cohort. IEEE Journal of Biomedical and Health In-
formatics , 22:346 – 353.
Prettz, J., Prado, K., Almeida, L., Frizon, M., Murari,
M., and Bertolini, C. (2015). MapMal
´
aria: um sis-
tema para visualizac¸
˜
ao e monitoramento dos casos de
mal
´
aria no Brasil. Anais do Computer on the Beach,
pages 328–337.
Sena, L., Deressa, W., and Ali, A. (2015). Correlation of
climate variability and malaria: a retrospective com-
parative study, southwest Ethiopia. Ethiopian journal
of health sciences, 25(2):129–138.
Sewe, M. O., Tozan, Y., Ahlm, C., and Rockl
¨
ov, J. (2017).
Using remote sensing environmental data to forecast
malaria incidence at a rural district hospital in Western
Kenya. Scientific Reports, 7(1):2589.
WHO (2018). WHO world malaria report 2018.
Wiefels, A., Wolfarth-Couto, B., Filizola, N., Durieux, L.,
and Mangeas, M. (2016). Accuracy of the malaria
epidemiological surveillance system data in the state
of Amazonas. Acta Amazonica, 46:383 – 390.
Zinszer, K., Verma, A. D., Charland, K., Brewer, T. F.,
Brownstein, J. S., Sun, Z., and Buckeridge, D. L.
(2012). A scoping review of malaria forecasting: Past
work and future directions. BMJ Open, 2(6):1–11.
CLOSER 2019 - 9th International Conference on Cloud Computing and Services Science
522