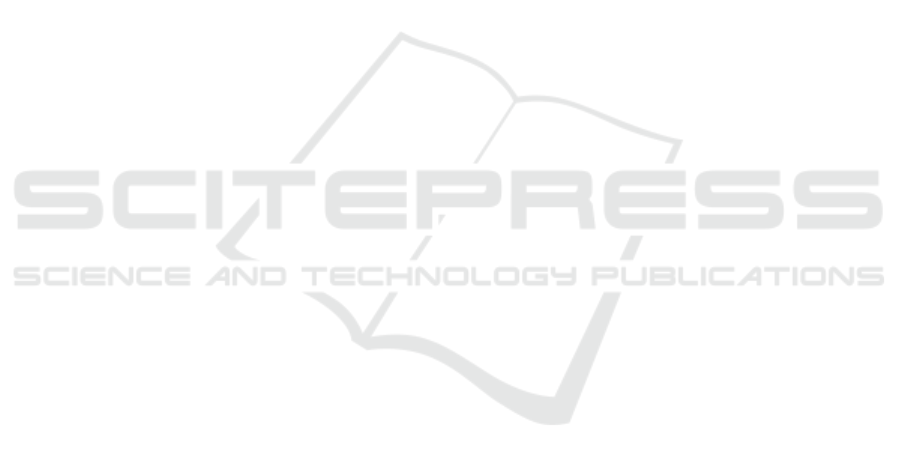
performance in terms of AUC, of up to 0.91 for
dropout prediction and 0.93 for result prediction in
case of Gradient Boosting Machine. The results also
showed that considering student demographics info
and assessment scores along with the VLE
interactions leads to a small 0.01 increase in AUC.
This research has focused on aggregate features
and did not made use of the date attribute available
for student assessments and VLE interactions. Future
work directions would focus on feature selection and
engineering, including time based metrics related to
assessments and student interactions to improve the
dropout and result prediction performance.
REFERENCES
Alshabandar, R., Hussain, A., Keight, R., Laws, A., and
Baker, T. (2018). The Application of Gaussian Mixture
Models for the Identification of At-Risk Learners in
Massive Open Online Courses. In 2018 IEEE Congress
on Evolutionary Computation (CEC) (pp. 18).
https://doi.org/10.1109/CEC.2018.8477770
Al-Shabandar, R., Hussain, A., Laws, A., Keight, R., Lunn,
J., and Radi, N. (2017). Machine learning approaches to
predict learning outcomes in Massive open online
courses. In 2017 International Joint Conference on
Neural Networks (IJCNN) (pp. 713720).
https://doi.org/10.1109/IJCNN.2017.7965922
Ashenafi, M. M., Riccardi, G., and Ronchetti, M. (2015).
activities. In 2015 IEEE Frontiers in Education
Conference (FIE) (pp. 19). https://doi.org/10.1109/
FIE.2015.7344081
Balakrishnan, G., and Coetzee, D. (2013). Predicting
student retention in massive open online courses using
hidden markov models (No. UCB/EECS-2013-109).
Electrical Engineering and Computer Sciences
University of California at Berkeley. Retrieved from
https://www2.
eecs.berkeley.edu/Pubs/TechRpts/2013/EECS-2013-1
09.pdf
Boyer, S., and Veeramachaneni, K. (2015). Transfer
Learning for Predictive Models in Massive Open
Online Courses. In C. Conati, N. Heffernan, A.
Mitrovic, and M. F. Verdejo (Eds.), Artificial
Intelligence in Education (AIED 2015) (pp. 5463).
Springer International Publishing.
Breslow, L., Pritchard, D. E., DeBoer, J., Stump, G. S., Ho,
A. D., and Seaton, D. T. (2013). Studying Learning in
MOOC. Research & Practice in Assessment, 8, 1325.
Chaplot, D. S., Rhim, E., and Kim, J. (2015). Predicting
Student Attrition in MOOCs using Sentiment Analysis
and Neural Networks. In Proceedings of the Workshops
at the 17th International Conference on Artificial
Intelligence in Education AIED 2015; Volume 3:
Fourth Workshop on Intelligent Support for Learning
in Groups (ISLG) (pp. 712). Madrid, Spain. Retrieved
from http://ceur-ws.org/Vol-1432/islg_pap2.pdf
Cobos, R., Wilde, A., and Zaluska, E. (2017). Predicting
attrition from massive open online courses in
FutureLearn and edX. In Joint MOOCs workshops from
the Learning Analytics and Knowledge (LAK)
Conference 2017 (pp. 7493). Simon Fraser University,
Vancouver, BC, Canada. Retrieved from http://ceur-
ws.org/Vol-1967/FLMOOCS_Paper5.pdf
Dalipi, F., Imran, A. S., and Kastrati, Z. (2018). MOOC
dropout prediction using machine learning techniques:
Review and research challenges. In 2018 IEEE Global
Engineering Education Conference (EDUCON) (pp.
10071014). https://doi.org/10.1109/EDUCON.2018.
8363340
Haiyang, L., Wang, Z., Benachour, P., and Tubman, P.
(2018). A Time Series Classification Method for
Behaviour-Based Dropout Prediction. In 2018 IEEE
18th International Conference on Advanced Learning
Technologies (ICALT) (pp. 191195). https://doi.org/
10.1109/ICALT.2018.00052
Heuer, H., and Breiter, A. (2018). Student Success
Prediction and the Trade-Off between Big Data and
Data Minimization. In D. Krömker and U. Schroeder
(Eds.), DeLFI 2018 - Die 16. E-Learning Fachtagung
Informatik. Bonn, Germany: Gesellschaft für
Informatik e.V. Retrieved from http://dl.gi.de/handle
/20.500.12116/16955
Hlosta, M., Zdrahal, Z., and Zendulka, J. (2017).
Ouroboros: Early Identification of At-risk Students
Without Models Based on Legacy Data. In Proceedings
of the Seventh International Learning Analytics &
Knowledge Conference (pp. 615). New York, NY,
USA: ACM. https://doi.org/10.1145/3027385.3027449
Hong, B., Wei, Z., and Yang, Y. (2017). Discovering
learning behavior patterns to predict dropout in MOOC.
In 2017 12th International Conference on Computer
Science and Education (ICCSE) (pp. 700704).
Houston, TX, USA.
https://doi.org/10.1109/ICCSE.2017.8085583
Kennedy, G., Coffrin, C., de Barba, P., and Corrin, L.
(2015).
Knowledge, Skills and Activities Predict MOOC
Performance. In Proceedings of the Fifth International
Conference on Learning Analytics And Knowledge (pp.
136140). New York, NY, USA: ACM.
https://doi.org/10.1145/ 2723576.2723593
Kuzilek, J., Hlosta, M., and Zdrahal, Z. (2017). Open
University Learning Analytics dataset. Scientific Data,
4, 170171. https://doi.org/10.1038/sdata.2017.171
Li, W., Gao, M., Li, H., Xiong, Q., Wen, J., and Wu, Z.
(2016). Dropout prediction in MOOCs using behavior
features and multi-view semi-supervised learning. In
2016 International Joint Conference on Neural
Networks (IJCNN) (pp. 31303137). Vancouver, BC,
Canada. https://doi.org/10.1109/IJCNN.2016.7727598
Liang, J., Li, C., and Zheng, L. (2016). Machine learning
application in MOOCs: Dropout prediction. In 2016
11th International Conference on Computer Science
OULAD MOOC Dropout and Result Prediction using Ensemble, Deep Learning and Regression Techniques
163