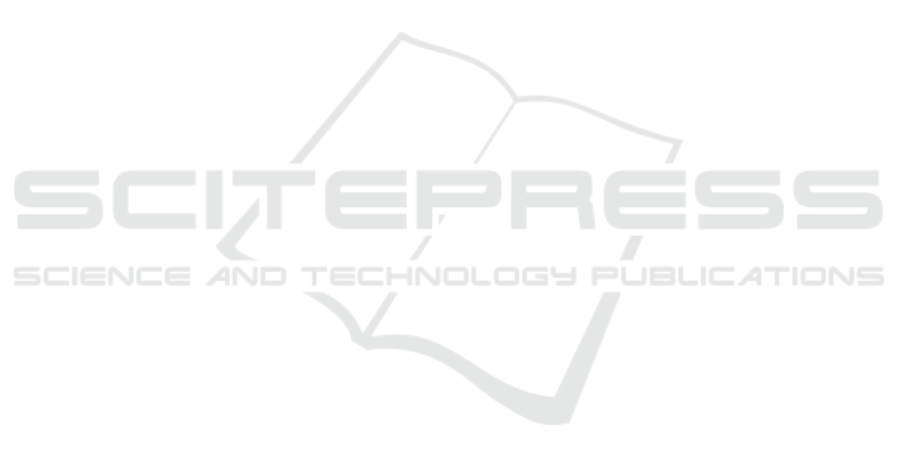
the coffee issue related mainly to prices and service,
which was changing his status.
In conclusion, this work contributes in the emerging
study of reputational risk through the Twitter network
is a very nascent topic in Latin America; therefore, it
should be considered as a new field of research to be
used by different organizations, since the reputation
is of the main assets intangible of a company.
For future work, the reputation risk can be supported
by supervised techniques of polarity classification,
this would help to improve the calculation of risk in
digital social networks. It has structural information
which can be integrated into the training data set as
the number of followers or the average number of
words and sentences for tweets, adding more
characteristics in the models of polarity classification.
ACKNOWLEDGEMENTS
This research was carried out by the Center of
Excellence and Appropriation in Big Data and
Data Analytics (CAOBA). It is being led by the
Pontificia Universidad Javeriana Colombia and it
was funded by the Ministry of Information
Technologies and Telecommunications of the
Republic of Colombia (MinTIC) through the
Colombian Administrative Department of Science,
Technology and Innovation (COLCIENCIAS)
within contract No. FP44842-anex46-2015.
REFERENCES
Aggarwal C.C. (2011) An Introduction to Social Network
Data Analytics. In: Aggarwal C. (eds) Social Network
Data Analytics. Springer, Boston, MA.
Becerra Bonza, D. C., and Guerrero García, T. M. (2012).
Social representations workers of a public organization
have about psychosocial risks and harassment in the
workplace towards the Promotion of Health, 17 (1),
118-132.
Bodin, Ö., and Crona, B. I. (2009). The role of social
networks in natural resource governance: What
relational patterns make a difference ?. Global
environmental change, 19 (3), 366-374.
Bodin, Ö., and Prell, C. (Eds.). (2011). Social networks and
natural resource management: uncovering the social
fabric of environmental governance. Cambridge
University Press.
Bourcier, D. (2003). Artificial intelligence and law.
Editorial UOC
Burt, R. S., Kilduff, M., and Tasselli, S. (2013). Social
Network Analysis: Foundations and Frontiers on
Advantage. http://doi.org/10.1146/annurev-psych-
113011-143828.
Contreras Barrera, M. (2014). Text mining: a current vision
University Library, vol. 17, no. 2, July-December,
2014, pp. 129-138 National Autonomous University of
Mexico Federal District, Mexico. University Library,
17 (2), 129-138
From Albornoz, J.C., Plaza, L., Gervás, P., and Díaz, A.
(2011, April). A joint model of feature mining and
sentiment analysis for product review rating. In
European conference on retrieval information (pp. 55-
66). Springer, Berlin, Heidelberg
E., from the Sabaté Source, J. M., and Garcia, J. B. D.
(2005). Corporate reputation and value creation. A
theoretical framework of a circular relationship.
European Investigations of Management and Economy
of the Company, 11 (2), 81-97.
Fernández-Gámez, M.A., Gil-Corral, A.M., and Galán-
Valdivieso, F. (2016). Corporate reputation and market
value: Evidence with generalized regression neural
networks. Expert Systems with Applications.
http://doi.org/10.1016/j.eswa.2015.10.028
Freeman, L. (2004). The development of social network
analysis. A Study in the Sociology of Science, 1.
Garicano, T. (2011). Corporate governance and corporate
reputation. Corporate Governance Center
http://doi.org/10.1016/j.dss.2013.09.004Hernández
Velasco, J. (2012). Reputational risk: Assess to prevent;
prevent to act. Give in, 32, 1-10.
Hernández M, and Gómez, J. (2013). Natural Language
Processing Applications. Polytechnic Journal, Vol. 32,
N, 87-96.
Kanayama, H., and Nasukawa, T. (2006, July). Fully
automatic lexicon expansion for domain-oriented
sentiment analysis. In Proceedings of the 2006
conference on empirical methods in natural language
processing (pp. 355-363). Association for
Computational Linguistics.
Kim, S. M., and Hovy, E. (2004, August). Determining the
sentiment of opinions. In Proceedings of the 20th
international conference on Computational Linguistics
(page 1367). Association for Computational
Linguistics.
Lamont, J. (2015). Risk Management: Reputation is
everything. KMWorld, 60 (1), 8. Retrieved from
http://ra.ocls.ca/ra/login.aspx?inst=conestoga&url=htt
p://search.ebscohost.com/login.aspx?direct=true&db=
bth&AN = 100327144 & site = bsi-live & scope = site.
Martín De Castro, G. (2008). Business reputation and
competitive advantage. Esic Editorial. Madrid. pp. 32-35.
Mika, P. (2004). Social networks and the semantic web.
Proceedings of the 2004 IEEE / WIC / ACM
International Conference on Web Intelligence.
http://doi.org/10.1109/WI.2004.10039
Mislove, A., Campus, E., Marcon, M., Gummadi, K. P., and
Druschel, P. (2007). Measurement and Analysis of
Online Social Networks.
Montes-y-Gómez, M., Gelbukh, A., and López-López, A.
(2002, July). Text mining at detail level using
conceptual graphs. In International Conference on
Conceptual Structures (pp. 122-136). Springer, Berlin,
Heidelberg.
ICEIS 2019 - 21st International Conference on Enterprise Information Systems
494