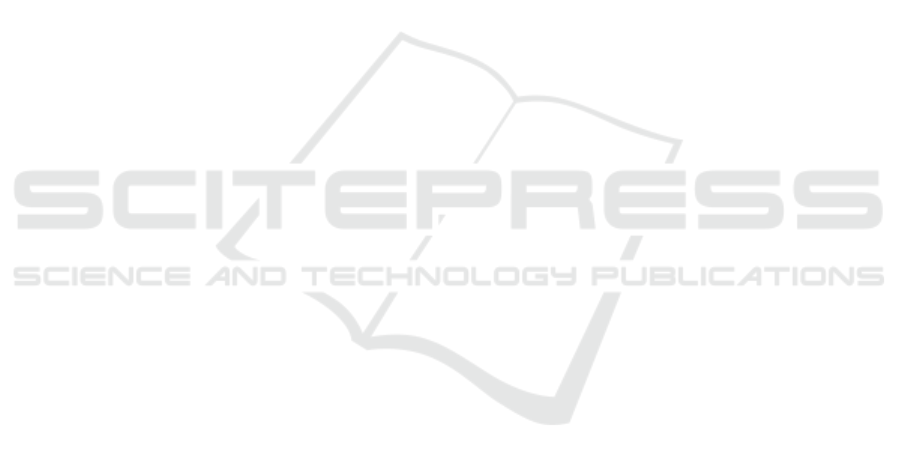
ACKNOWLEDGEMENTS
This work was supported by the University of Latvia
and LMT Ltd. [grant number AAP2016/B089].
REFERENCES
Ahas, R., Aasa, A., Silm, S., Tiru, M., 2010. Daily
rhythms of suburban commuters’ movements in the
Tallinn metropolitan area: Case study with mobile
positioning data. Transportation Research Part C,
18(1), pp.45–54.
Ahas, R., Aasa, A., Roose, A., Mark, U., Silm, S., 2008.
Evaluating passive mobile positioning data for tourism
surveys: An Estonian case study. Tourism
Management, 29, pp.469–486.
Ahas, R., Aasa, A., Mark, U., Pae, T., Kull, A., 2007.
Seasonal tourism spaces in Estonia: Case study with
mobile positioning data. Tourism Management, 28,
pp.898–910.
Ahas, R., Mark, U., 2005. Location based services — new
challenges for planning and public administration?
Futures, 37, pp.547–561.
Alexander, L., Jiang, S., Murga, M., Gonzalez, M. C.,
2015. Origin – destination trips by purpose and time of
day inferred from mobile phone data. Transportation
Research Part C, 58, pp.240–250.
Arhipova, I., Berzins, G., Brekis, E., Opmanis, M., Binde,
J., Steinbuka, I., Kravcova, J., 2019. Pattern
Identification by Factor Analysis for Regions with
Similar Economic Activity Based on Mobile
Communication Data. Advances in Intelligent Systems
and Computing, 886, pp.561–569.
Arhipova, I., Berzins, G. Brekis, E., Kravcova, J., Binde,
J., 2017. The methodology of region economic
development evaluation using mobile positioning data.
In D. Cingula, ed. 20th International Scientific
Conference on Economic and Social Development.
Prague: Varazdin Development and Entrepreneurship
Agency, Varazdin, Croatia. City of Prague. University
North, Koprivnica, Croatia. Faculty of Management
University of Warsaw, Warsaw, Poland, pp. 111–120.
Balzotti, C., Bragagnini, A., Briani, M., Cristiani, E.,
2018. Understanding Human Mobility Flows from
Aggregated Mobile Phone Data. IFAC-PapersOnLine,
51(9), pp.25–30.
Bellù, L.G., Liberati, P., 2006. Describing Income
Inequality. Theil Index and Entropy Class Indexes,
Available at: www.fao.org/tc/easypol.
Bonnel, P., Hombourger, E., Olteanu-Raimond, A.-M.,
Smoreda, Z., 2015. Passive mobile phone dataset to
construct origin-destination matrix: potentials and
limitations. Transportation Research Procedia, 11,
pp.381–398.
Bwambale, A., Choudhury, C.F., Hess, S., 2017.
Modelling trip generation using mobile phone data: A
latent demographics approach. Journal of Transport
Geography, (August), p.article in press.
Chen, C., Bian, L., Ma, J., 2014. From traces to
trajectories: How well can we guess activity locations
from mobile phone traces? Transportation Research
Part C, 46, pp.326–337.
Chetty, G., White, M., Akther, F., 2015. Smart Phone
Based Data Mining For Human Activity Recognition.
Procedia Computer Science, 46, pp.1181–1187.
Deville, P., Linard, C., Martin, S., Gilbert, M., Stevens,
F.R., Gaughan, A.E., Blondel, V.D., Tatem, A.J.,
2014. Dynamic population mapping using mobile
phone data. PNAS (Proceedings of the National
Academy of Sciences of the United States of America),
111(45), pp.15888–15893.
Dong, H., Wu, M., Ding, X., Chu, L., Jia, L., Qin, Y.,
Zhou, X., 2015. Traffic zone division based on big
data from mobile phone base stations. Transportation
Research Part C, 58, pp.278–291.
Elias, D., Nadler, F., Stehno, J., Krosche, J., Lindorfer, M.,
2016. SOMOBIL – improving public transport
planning through mobile phone data analysis.
Transportation Research Procedia, 14, pp.4478–4485.
European Parliament, 2016. REGULATION (EU)
2016/679 OF THE EUROPEAN PARLIAMENT
AND OF THE COUNCIL of 27 April 2016 on the
protection of natural persons with regard to the
processing of personal data and on the free movement
of such data, and repealing Directive 95/46/EC
(General Da. Official Journal of the European Union,
L 119, pp.1–88.
Hatziioannidu, F., Polydoropoulou, A., 2017. Passenger
Demand And Patterns Of Tourists’ Mobility In The
Aegean Archipelago With Combined Use Of Big
Datasets from Mobile Phones and Statistical Data
from Ports and Airports. Transportation Research
Procedia, 25, pp.2309–2329.
Hongyan, G., Fasheng, L., 2013. Estimating freeway
traffic measures from mobile phone location data.
European Journal of Operational Research, 229(1),
pp.252–260.
Hoteit, S., Secci, S., Sobolevsky, S., Ratti, C., Pujolle, G.,
2014. Estimating human trajectories and hotspots
through mobile phone data. Computer Networks, 64,
pp.296–307.
Jonge, E. de, Pelt, M. van, Roos, M., 2012. Time patterns,
geospatial clustering and mobility statistics based on
mobile phone network data. The Hague: Statistics
Netherlands.
Kuusik, A., Nilbe, K., Mehine, T., Ahas, R., 2014.
Country as a free sample: the ability of tourism events
to generate repeat visits. Case study with mobile
positioning data in Estonia. Procedia - Social and
Behavioral Sciences, 148, pp.262–270.
Larijani, A.N., Olteanu-Raimond, A.-M., Perret, J., Bredif,
M., Ziemlicki, C., 2015. Investigating the mobile
phone data to estimate the origin destination flow and
analysis; case study: Paris region. Transportation
Research Procedia, 6, pp.64–78.
Lee, K.-S., You, S.Y., Eom, J.K., Song, J., Min, J.H.,
2018. Urban spatiotemporal analysis using mobile
phone data: Case study of medium- and large-sized
Mobile Phone Data Statistics as Proxy Indicator for Regional Economic Activity Assessment
35