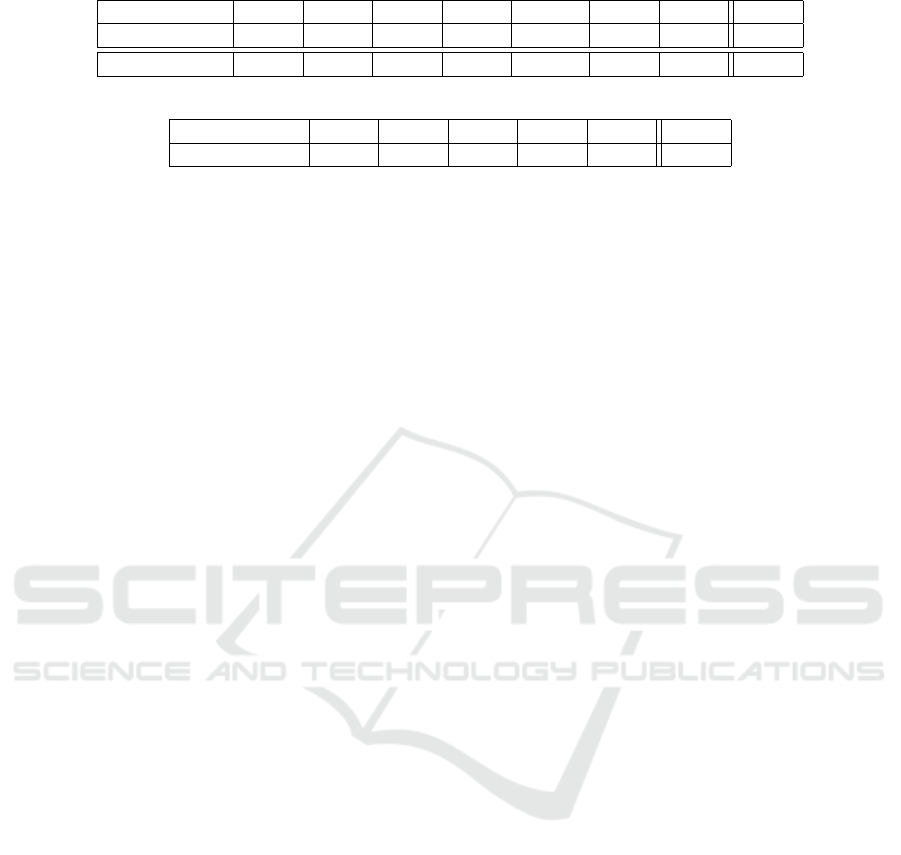
Table 4: Comparison of fault detection performance (%) for the TEP, with reduced FTrM.
FDA PCA PLS PCA DPCA BN ICA BG
Average FdR 79.13 90.60 90.82 90.86 92.39 98.92 98.95 99.94
FAR 3.11 6.13 10 1.56 10.13 1.13 2.75 0.38
Table 5: Comparison of fault diagnosis performance (%) for the TEP, with reduced FTrM.
SNN SVM PCA NN BN BG
Average FDR 60.63 62.77 74.82 79.13 95.78 88.66
(SNN) (Eslamloueyan, 2011), Support Vector Ma-
chine (SVM) (Jing and Hou, 2015), PCA (Jing and
Hou, 2015), NN (Eslamloueyan, 2011), BN (Verron,
2007)), based solely on data, and the proposed en-
hanced BG approach is addressed.
The absence of model-based approaches within this
comparison is due to the fact that no work has at-
tempted to detect and diagnose the faults affecting the
TEP by using a model. The red color indicates the
best result.
According to Table 4, it appears that the pro-
posed BG approach presents the best detection per-
formances. Indeed, it has the highest FdR (99.94%).
6 faults are perfectly detected (D
1
, D
2
, D
4
, D
5
, D
7
, D
8
)
in 100% of the observations. Furthermore, the BG ap-
proach shows the lowest FAR (0.38%).
Thus, the proposed BG approach shows the best FAR
and FdR.
Regarding the diagnosis performances, the pro-
posed BG approach shows the second best perfor-
mance, with an average FDR of 88.66%, as indicated
in Table 4.
Accordingly, the BG approach, enhanced with the
FTrA, presents better or comparable performances
than many data-driven methods reported in the litera-
ture.
5 CONCLUSION
In this work, an enhanced model-based approach was
proposed for fault detection and diagnosis of a well-
known industrial benchmark: the Tennessee Eastman
process.
The proposed approach improves the classical
fault detection and diagnosis model-based scheme by
extending it to an experimental approach, i.e. the
Fault Training Analysis, that exploits the available
historical data from nominal as well as faulty states.
The purpose of this latter is to identify the causal re-
lationships between residuals and faults. The fault
training analysis results on an experimental matrix,
called Fault Training Matrix, that enhances the theo-
retical Fault Signature Matrix.
The proposed approach was validated through the
Tennessee Eastman Process, and shows a high fault
detection rate, a high fault diagnosis rate and a small
false alarm rate.
REFERENCES
Areepong, Y. (2013). A comparison of performance of
residual control charts for trend stationary processes.
International Journal of Pure and Applied Mathemat-
ics, 85(3):583–592.
Atoui, M. A., Verron, S., and Kobi, A. (2016). A bayesian
network dealing with measurements and residuals for
system monitoring. Transactions of the Institute of
Measurement and Control, 38(4):373–384.
Chatti, N., Guyonneau, R., Hardouin, L., Verron, S.,
and Lagrange, S. (2016). Model-based approach
for fault diagnosis using set-membership formula-
tion. Engineering Applications of Artificial Intelli-
gence, 55:307–319.
Chatti, N., Ould-Bouamama, B., Gehin, A.-L., and Mer-
zouki, R. (2014). Signed bond graph for multiple
faults diagnosis. Engineering Applications of Artifi-
cial Intelligence, 36:134–147.
Ding, S. (2008). Model-based fault diagnosis techniques:
design schemes, algorithms, and tools. Springer Sci-
ence & Business Media.
Ding, S. X., Zhang, P., Naik, A., Ding, E. L., and Huang,
B. (2009). Subspace method aided data-driven design
of fault detection and isolation systems. Journal of
process control, 19(9):1496–1510.
Downs, J. J. and Vogel, E. F. (1993). A plant-wide indus-
trial process control problem. Computers & chemical
engineering, 17(3):245–255.
Eslamloueyan, R. (2011). Designing a hierarchical neural
network based on fuzzy clustering for fault diagnosis
of the tennessee–eastman process. Applied soft com-
puting, 11(1):1407–1415.
Gao, Z., Cecati, C., and Ding, S. X. (2015). A survey
of fault diagnosis and fault-tolerant techniques—part
i: Fault diagnosis with model-based and signal-based
approaches. IEEE Transactions on Industrial Elec-
tronics, 62(6):3757–3767.
Ghosh, K., Ng, Y. S., and Srinivasan, R. (2011). Evaluation
of decision fusion strategies for effective collaboration
among heterogeneous fault diagnostic methods. Com-
puters & chemical engineering, 35(2):342–355.
Fault Training Matrix for Process Monitoring based on Structured Residuals
29