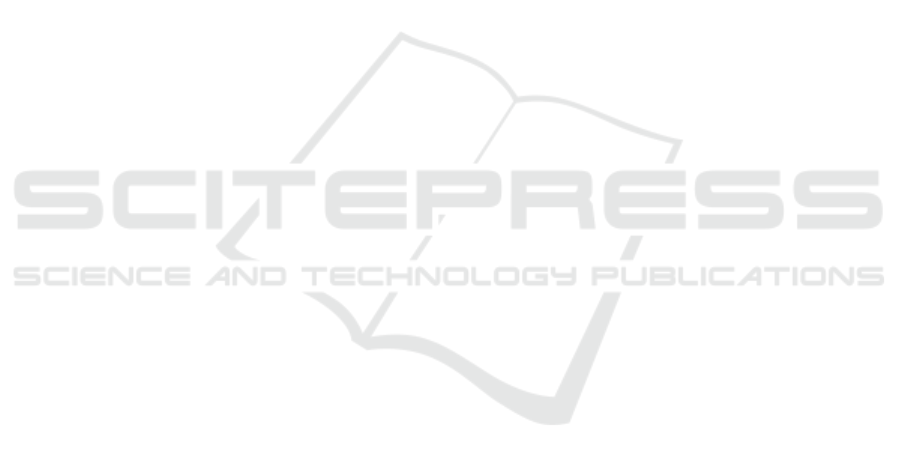
5 CONCLUSIONS
This paper introduced an emotion recognition method
by using PPG and EMG signals. In order to classify
the detailed emotion, we subdivided the valence and
arousal as 4 levels, whereas the existing method of
dividing emotion was 2 levels. For the experiment,
we obtained own dataset by extracting from 30 sub-
jects using video clips. We adopted CNN architecture
for extracting features of signals and classifying the
valence and arousal. To use the PPG and EMG sig-
nal as an input of deep learning, we segmented and
concatenated them. The proposed method identified
individual and overall result with 90 to 96% and 83%
accuracies respectively.
REFERENCES
www.physiolab.co.kr.
Ahonen, T., Hadid, A., and Pietikainen, M. (2006). Face
description with local binary patterns: Application to
face recognition. IEEE Transactions on Pattern Anal-
ysis & Machine Intelligence, (12):2037–2041.
Alarcao, S. M. and Fonseca, M. J. (2017). Emotions recog-
nition using eeg signals: A survey. IEEE Transactions
on Affective Computing.
An, S., Ji, L.-J., Marks, M., and Zhang, Z. (2017). Two sides
of emotion: exploring positivity and negativity in six
basic emotions across cultures. Frontiers in psychol-
ogy, 8:610.
Anagnostopoulos, C.-N., Iliou, T., and Giannoukos, I.
(2015). Features and classifiers for emotion recog-
nition from speech: a survey from 2000 to 2011. Ar-
tificial Intelligence Review, 43(2):155–177.
Cowie, R., Douglas-Cowie, E., Tsapatsoulis, N., Votsis,
G., Kollias, S., Fellenz, W., and Taylor, J. G. (2001).
Emotion recognition in human-computer interaction.
IEEE Signal processing magazine, 18(1):32–80.
Donahue, J., Jia, Y., Vinyals, O., Hoffman, J., Zhang, N.,
Tzeng, E., and Darrell, T. (2014). Decaf: A deep con-
volutional activation feature for generic visual recog-
nition. In International conference on machine learn-
ing, pages 647–655.
Hudlicka, E. (2003). To feel or not to feel: The role of affect
in human–computer interaction. International journal
of human-computer studies, 59(1-2):1–32.
Kim, W.-G. (2017). Emotional speaker recognition us-
ing emotional adaptation. CHONGI HAKHOE NON-
MUNJI, 66(7):1105–1110.
Lee, Y.-K., Kwon, O.-W., Shin, H. S., Jo, J., and Lee, Y.
(2011). Noise reduction of ppg signals using a par-
ticle filter for robust emotion recognition. In Con-
sumer Electronics-Berlin (ICCE-Berlin), 2011 IEEE
International Conference on, pages 202–205. IEEE.
Peng, S., Zhang, L., Ban, Y., Fang, M., and Winkler, S.
(2018). A deep network for arousal-valence emotion
prediction with acoustic-visual cues. arXiv preprint
arXiv:1805.00638.
Russell, J. A. (1980). A circumplex model of affect. Journal
of personality and social psychology, 39(6):1161.
Seyeditabari, A., Tabari, N., and Zadrozny, W. (2018).
Emotion detection in text: a review. arXiv preprint
arXiv:1806.00674.
Tabar, Y. R. and Halici, U. (2016). A novel deep learning
approach for classification of eeg motor imagery sig-
nals. Journal of neural engineering, 14(1):016003.
Wang, H.-M. and Huang, S.-C. (2014). Musical rhythms af-
fect heart rate variability: Algorithm and models. Ad-
vances in Electrical Engineering, 2014.
Wu, G., Liu, G., and Hao, M. (2010). The analysis of
emotion recognition from gsr based on pso. In Intel-
ligence Information Processing and Trusted Comput-
ing (IPTC), 2010 International Symposium on, pages
360–363. IEEE.
Yin, Z., Zhao, M., Wang, Y., Yang, J., and Zhang, J.
(2017). Recognition of emotions using multimodal
physiological signals and an ensemble deep learn-
ing model. Computer methods and programs in
biomedicine, 140:93–110.
Yoo, G., Seo, S., Hong, S., and Kim, H. (2018). Emo-
tion extraction based on multi bio-signal using back-
propagation neural network. Multimedia Tools and
Applications, 77(4):4925–4937.
Zhang, D., Yao, L., Zhang, X., Wang, S., Chen, W.,
and Boots, R. (2017). Eeg-based intention recogni-
tion from spatio-temporal representations via cascade
and parallel convolutional recurrent neural networks.
arXiv preprint arXiv:1708.06578.
ICINCO 2019 - 16th International Conference on Informatics in Control, Automation and Robotics
600