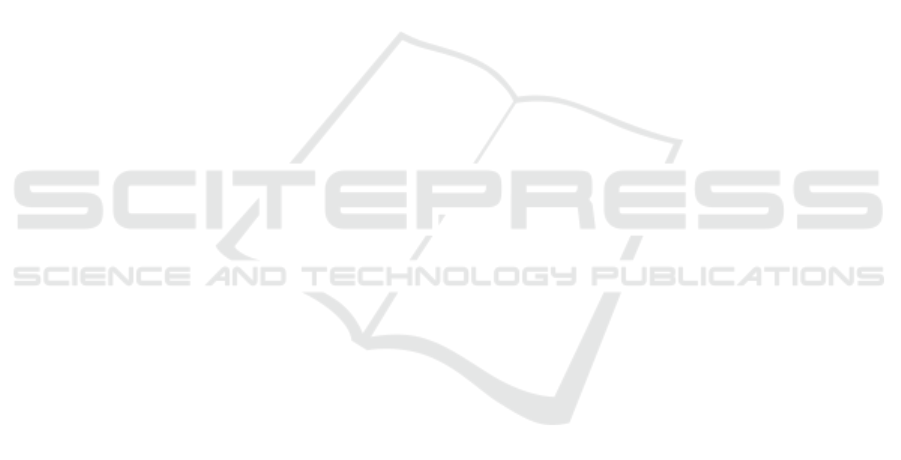
the IWSC dataset. Our best performing algorithm
TSR-e showing a hit-rate@10 of 77% on the
SL_consumers task.
The focus of this paper has been on introducing
the TSR technique and IWSC dataset and tasks. Both
contributions open new avenues for further
investigation into the properties of extra sparse, and
subset labelled datasets. Future work could examine
the effects of different embedding models on TSR
and prediction of supply chain competitors and test if
different fine-tuning of these models would further
improve results.
Additional future work could include a
comprehensive analysis of the performance of the
TSR technique on other datasets and how it compares
to or could be used in conjunction with other
recommender systems, particularly as the number of
labels is increased. TSR could also be applied to
expand the number of relationships in a partially
labelled dataset to allow the use of algorithms that
struggle with cold starts or require many training
examples.
REFERENCES
IWChamber (2018). Retrieved from IWChamber:
https://www.iwchamber.co.uk
IWTechnology (2018). Retrieved from IWTechnology:
http://iwtechnology.co.uk/
Marine Southeast (2018). Retrieved from Marine
Southeast: http://www.marinesoutheast.co.uk/
Bowman, S. R., Angeli, G., Potts, C., and Manning, C. D.
(2015). A large annotated corpus for learning natural
language inference. https://doi.org/10.18653/v1/D16-
1264
Cer, D., Yang, Y., Kong, S., Hua, N., Limtiaco, N., John,
R. St., … Kurzweil, R. (2018). Universal Sentence
Encoder. Retrieved from http://
arxiv.org/abs/1803.11175
Grady, C., and Lease, M. (2010). Crowdsourcing
Document Relevance Assessment with Mechanical
Turk, (June), 172–179.
Harper, F. M., and Konstan, J. A. (2015). The MovieLens
Datasets : History and Context r r r, 5(4), 1–19.
He, X., Liao, L., Zhang, H., Nie, L., Hu, X., and Chua, T.-
S. (2017). Neural Collaborative Filtering.
https://doi.org/10.1145/3038912.3052569
Hu, M., and Liu, B. (2004). Mining and summarizing
customer reviews. Proceedings of the 2004 ACM
SIGKDD International Conference on Knowledge
Discovery and Data Mining - KDD ’04, 168.
https://doi.org/10.1145/1014052.1014073
Järvelin, K., and Kekäläinen, J. (2002). Cumulated gain-
based evaluation of IR techniques. ACM Transactions
on Information Systems. https://
doi.org/10.1145/582415.582418
Koren, Y. (2008). Factorization meets the neighborhood: a
multifaceted collaborative filtering model. In
Proceedings of the 14th ACM SIGKDD international
conference on Knowledge discovery and data mining.
https://doi.org/10.1145/1401890.1401944
Maaten, L. Van Der, and Hinton, G. (2008). Visualizing
Data using t-SNE, 9, 2579–2605.
Pang, B., and Lee, L. (2004). A Sentimental Education:
Sentiment Analysis Using Subjectivity Summarization
Based on Minimum Cuts. https://
doi.org/10.3115/1218955.1218990
Snow, R., Connor, B. O., Jurafsky, D., Ng, A. Y., Labs, D.,
and St, C. (2008). Cheap and Fast — But is it Good ?
Evaluating Non-Expert Annotations for Natural
Language Tasks, (October), 254–263.
Suglia, A., Greco, C., Musto, C., De Gemmis, M., Lops, P.,
and Semeraro, G. (2017). A deep architecture for
content-based recommendations exploiting recurrent
neural networks. [UMAP2017]Proceedings of the 25th
Conference on User Modeling, Adaptation and
Personalization, 202–211. https://
doi.org/10.1145/3079628.3079684
Vuurens, J. B. P., Larson, M., and de Vries, A. P. (2016).
Exploring Deep Space: Learning Personalized Ranking
in a Semantic Space. Proceedings of the 1st Workshop
on Deep Learning for Recommender Systems - DLRS
2016, 23–28. https://
doi.org/10.1145/2988450.2988457
Xu, Z., Chen, C., Lukasiewicz, T., Miao, Y., and Meng, X.
(2016). Tag-Aware Personalized Recommendation
Using a Deep-Semantic Similarity Model with
Negative Sampling. Proceedings of the 25th ACM
International on Conference on Information and
Knowledge Management - CIKM ’16.
https://doi.org/10.1145/2983323.2983874
Zhang, F., Yuan, N. J., Lian, D., Xie, X., and Ma, W.-Y.
(2016). Collaborative Knowledge Base Embedding for
Recommender Systems. Proceedings of the 22nd ACM
SIGKDD International Conference on Knowledge
Discovery and Data Mining - KDD ’16, 353–362.
https://doi.org/10.1145/2939672.2939673
IoTBDS 2019 - 4th International Conference on Internet of Things, Big Data and Security
194