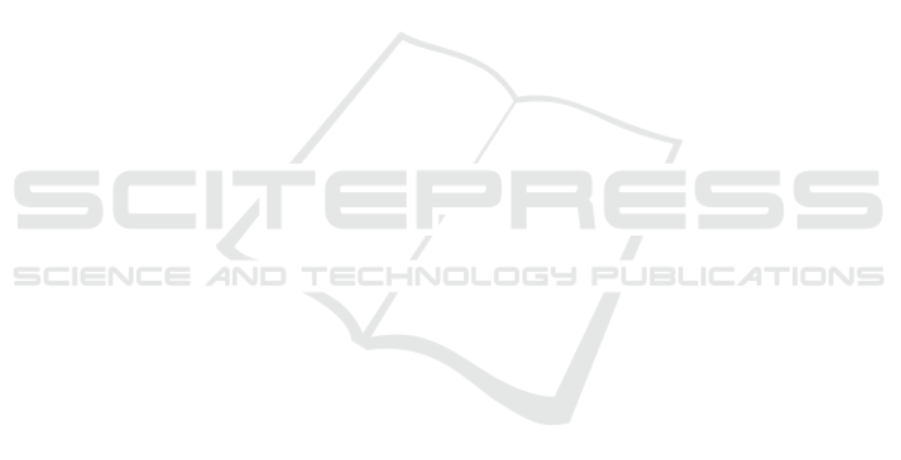
5 CONCLUSIONS
This paper presented a framework for personalised in-
teraction in ADAS systems, taking into account the
driver profile and state as well as the situational and
environmental context. The framework relies on a
rule engine that uses a customisable and extensible
set of personalisation and adaptation rules to produce
the decisions for selecting the most appropriate HMI
elements and personalising GUI applications.
Personalised HMI modality selection is realised
by taking into account all input and output modalities
of the vehicle, classifying them into categories asso-
ciated with HMI element interfaces and maintaining
bindings for their activation. Multimodal input is also
supported by separating virtual input and the related
application interaction commands from physical in-
put, and by allowing to connect multiple physical in-
put devices to each HMI element interface.
GUI application personalisation is achieved
through an extensible GUI toolkit of adaptive and per-
sonalisable user controls that is offered by the frame-
work to be employed in the development of applica-
tions requiring personalisation features. The toolkit
integrates personalisation and adaptation capabilities
thus abstracting these features from developers of
software for automotive applications.
The framework has been developed and adopted
in two case studies to validate its applicability. As
the ADAS&ME project progresses, the framework
will be further integrated in demonstrator vehicles and
eventually evaluated in the project pilot sites along
with the HMI interaction and its personalisation and
adaptation features. Future work includes the explo-
ration of a machine learning approach for triggering
HMI personalisation that also considers driver feed-
back and responses to prior system actions.
ACKNOWLEDGEMENTS
This project has received funding from the Euro-
pean Union’s Horizon 2020 research and innova-
tion programme under grant agreement No. 688900
(ADAS&ME). The original design for the HMI of
Use Case B of ADAS&ME was conducted by Valeo.
REFERENCES
Amditis, A., Bekiaris, E., Montanari, R., Baligand, B.,
et al. (2001). An innovative in-vehicle multimedia
HMI based on an intelligent information manager ap-
proach: the Comunicar design process. In 8th World
Congress on Intelligent Transport Systems.
Brouwer, R. F., Hoedemaeker, M., and Neerincx, M. A.
(2009). Adaptive interfaces in driving. In FAC 2009,
pages 13–19. Springer Berlin Heidelberg.
Craig, J. (2012). Map Data for ADAS. In Handbook of
Intelligent Vehicles, pages 881–892. Springer London.
Fischer, P. and N
¨
urnberger, A. (2010). myCOMAND au-
tomotive user interface: Personalized interaction with
multimedia content based on fuzzy preference model-
ing. In UMAP 2010, pages 315–326.
Garzon, S. and Poguntke, M. (2011). The personal adap-
tive in-car HMI: integration of external applications
for personalized use. In UMAP 2012, pages 35–46.
Garzon, S. R. (2012). Intelligent In-Car-Infotainment Sys-
tems: A Contextual Personalized Approach. In IE
2012, pages 315–318.
Hassel, L. and Hagen, E. (2006). Adaptation of an automo-
tive dialogue system to users’ expertise and evaluation
of the system. Language resources and evaluation,
40(1):67–85.
H
´
el
´
ene, T. V., Thierry, B., et al. (2005). Development
of a driver situation assessment module in the AIDE
project. IFAC Proceedings Volumes, 38(1):97–102.
Knauss, A., Diederichs, F., Wilbrink, M., et al. (2018).
An HMI Framework for Driver/Rider States Adaptive
Transition and ADAS. In 25th ITS World Congress.
Lee, J. D., Hoffman, J. D., and Hayes, E. (2004). Collision
warning design to mitigate driver distraction. In CHI
2004, pages 65–72. ACM.
Lilis, Y., Zidianakis, E., Partarakis, N., Antona, M., and
Stephanidis, C. (2017). Personalizing HMI Elements
in ADAS Using Ontology Meta-Models and Rule
Based Reasoning. In UAHCI 2017, pages 383–401.
Lu, M., Wevers, K., and Van Der Heijden, R. (2005). Tech-
nical feasibility of advanced driver assistance systems
(ADAS) for road traffic safety. Transportation Plan-
ning and Technology, 28(3):167–187.
Mueller, M. (2014). Deficiency drive. Vision Zero Interna-
tional.
Piao, J. and McDonald, M. (2008). Advanced driver as-
sistance systems from autonomous to cooperative ap-
proach. Transport Reviews, 28(5):659–684.
Recarte, M. A. and Nunes, L. M. (2008). Mental work-
load while driving: effects on visual search, discrimi-
nation, and decision making. Journal of experimental
psychology: Applied, 9(2):119.
Tigadi, A., Gujanatti, R., and Gonchi, A. (2016). Ad-
vanced Driver Assistance Systems. International
Journal of Engineering Research and General Sci-
ence, 4(3):2091–2730.
Tonnis, M., Lange, C., and Klinker, G. (2007). Visual Lon-
gitudinal and Lateral Driving Assistance in the Head-
Up Display of Cars. In ISMAR 2007, pages 91–94.
Werner, K. (2018). Five Short Display Stories from CES
2018. Information Display, 34(2):28–34.
Zidianakis, E., Antona, M., and Stephanidis, C. (2017).
ACTA: A general purpose Finite State Machine
(FSM) description language for smart game design.
In IHCI 2017, pages 143–150. IADIS Press.
A Framework for Personalised HMI Interaction in ADAS Systems
593