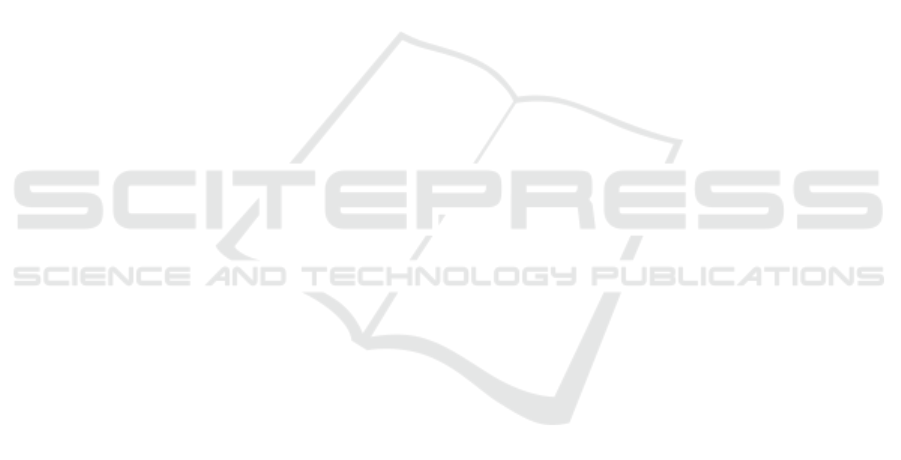
applications. Manchester University Press and Prince-
ton University Press.
H
¨
anninen, J. and K
¨
arkk
¨
ainen, T. (2016). Comparison
of four-and six-layered configurations for deep net-
work pretraining. In European Symposium on Artifi-
cial Neural Networks, Computational Intelligence and
Machine Learning.
Hbali, Y., Sadgal, M., and Fazziki, A. E. (2013). Mark-
erless augmented reality based on local binary pat-
tern. In Signal Processing and Multimedia Applica-
tions (SIGMAP), 2013 International Conference on,
pages 137–141. IEEE.
Huynh, T., Gao, Y., Kang, J., Wang, L., Zhang, P., Lian,
J., and Shen, D. (2016). Estimating ct image from
mri data using structured random forest and auto-
context model. IEEE transactions on medical imag-
ing, 35(1):174.
Japkowicz, N., Hanson, S. J., and Gluck, M. A. (2000).
Nonlinear autoassociation is not equivalent to pca.
Neural computation, 12(3):531–545.
Jia, F., Lei, Y., Lin, J., Zhou, X., and Lu, N. (2016). Deep
neural networks: A promising tool for fault character-
istic mining and intelligent diagnosis of rotating ma-
chinery with massive data. Mechanical Systems and
Signal Processing, 72:303–315.
Jiang, G., Xie, P., He, H., and Yan, J. (2018). Wind turbine
fault detection using a denoising autoencoder with
temporal information. IEEE/ASME Transactions on
Mechatronics, 23(1):89–100.
Lee, C.-C. (2014). Gender classification using m-estimator
based radial basis function neural network. In Signal
Processing and Multimedia Applications (SIGMAP),
2014 International Conference on, pages 302–306.
IEEE.
Liaw, A. and Wiener, M. (2002). Classification and regres-
sion by randomforest. R news, 2(3):18–22.
Liu, Z., Tang, B., He, X., Qiu, Q., and Liu, F. (2017).
Class-specific random forest with cross-correlation
constraints for spectral–spatial hyperspectral image
classification. IEEE Geoscience and Remote Sensing
Letters, 14(2):257–261.
Maha, C., Maher, E., Mohamed, K., and Chokri, B. A.
(2010). Dct based blind audio watermarking scheme.
In Signal Processing and Multimedia Applications
(SIGMAP), Proceedings of the 2010 International
Conference on, pages 139–144. IEEE.
Mart
´
ınez-Rego, D., Fontenla-Romero, O., and Alonso-
Betanzos, A. (2011). Power wind mill fault detection
via one-class ν-svm vibration signal analysis. In Neu-
ral Networks (IJCNN), The 2011 International Joint
Conference on, pages 511–518. IEEE.
Mishra, K. M., Krogerus, T., and Huhtala, K. (2019). Fault
detection of elevator systems using deep autoencoder
feature extraction. In Research Challenges in Infor-
mation Science (RCIS), 2019 International Confer-
ence on. IEEE.
Nam, S.-H. and Yang, M.-Y. (2004). A study on a
generalized parametric interpolator with real-time
jerk-limited acceleration. Computer-Aided Design,
36(1):27–36.
Rhudy, M. (2014). Time alignment techniques for experi-
mental sensor data. Int. J. Comput. Sci. Eng. Survey,
5(2):1–14.
Rivas-Perez, M., Linares-Barranco, A., Jimenez-
Fernandez, A., Civit, A., and Jimenez, G. (2011). Aer
spike-processing filter simulator: Implementation of
an aer simulator based on cellular automata. In Signal
Processing and Multimedia Applications (SIGMAP),
2011 Proceedings of the International Conference on,
pages 1–6. IEEE.
Sahba, F. and Venetsanopoulos, A. (2010). Breast mass de-
tection using bilateral filter and mean shift based clus-
tering. In Signal Processing and Multimedia Appli-
cations (SIGMAP), Proceedings of the 2010 Interna-
tional Conference on, pages 88–94. IEEE.
Soyka, F., Giordano, P. R., Beykirch, K., and B
¨
ulthoff,
H. H. (2011). Predicting direction detection thresh-
olds for arbitrary translational acceleration profiles in
the horizontal plane. Experimental brain research,
209(1):95–107.
Sun, W., Shao, S., Zhao, R., Yan, R., Zhang, X., and Chen,
X. (2016). A sparse auto-encoder-based deep neural
network approach for induction motor faults classifi-
cation. Measurement, 89:171–178.
Vincent, P., Larochelle, H., Bengio, Y., and Manzagol, P.-
A. (2008). Extracting and composing robust features
with denoising autoencoders. In Proceedings of the
25th international conference on Machine learning,
pages 1096–1103. ACM.
Wang, X., Wu, S., and Wang, K. (2015). A run-to-run
profile control algorithm for improving the flatness of
nano-scale products. IEEE Transactions on Automa-
tion Science and Engineering, 12(1):192–203.
Xia, M., Li, T., Xu, L., Liu, L., and de Silva, C. W.
(2018). Fault diagnosis for rotating machinery us-
ing multiple sensors and convolutional neural net-
works. IEEE/ASME Transactions on Mechatronics,
23(1):101–110.
Yang, Z.-X. and Zhang, P.-B. (2016). Elm meets rae-elm:
A hybrid intelligent model for multiple fault diagnosis
and remaining useful life predication of rotating ma-
chinery. In Neural Networks (IJCNN), 2016 Interna-
tional Joint Conference on, pages 2321–2328. IEEE.
SIGMAP 2019 - 16th International Conference on Signal Processing and Multimedia Applications
320