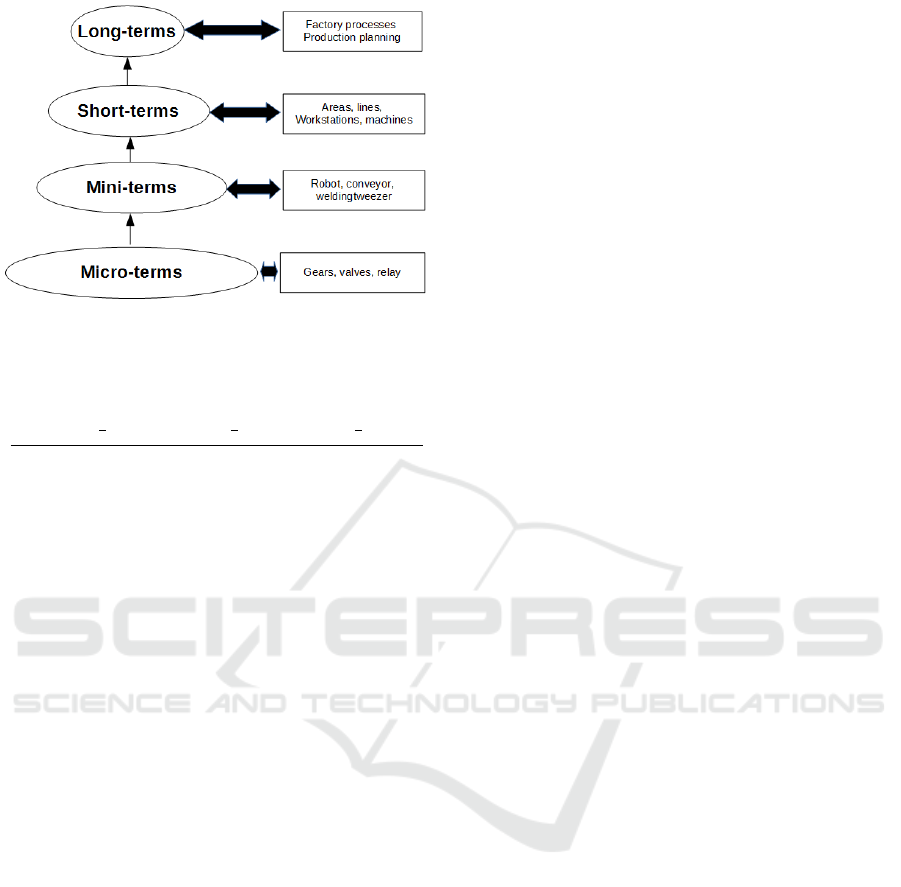
Figure 2: From Micro-term to Long-term.
Table 2: Measurements of the Experimental Test for each
mini-term and pathology.
Robot Movement Clamp movement Welding clamp
(x, S) (x, S) (x, S)
C 35.5497;0.0215 0.4158;0.0061 1.4373;0.0109
P
1
35.5472;0.0336 0.4302;0.0060 4.0523;0.1585
P
2
35.5496;0.0257 1.4087;0.0488 1.1391;0.0783
P
3
35.5492;0.0361 0.4643;0.0070 1.4389;0.0119
P
4
35.5485;0.0302 1.5594;0.0489 1.2945;0.0665
P
5
46.3314;0.0314 0.4185;0.0060 1.4489;0.0110
used to measure time. To carry out this study, compo-
nents with an advanced lifetime were selected. These
components are in normal production where nobody
notices a failure in their behavior. These pathologies
are: the failure of the proportional valve, the cylin-
der stiffness, loss of insulation in the welding trans-
former, loss of pneumatic pressure and loss of robot
speed. Table 2 shows the measurements of experi-
mental results for each mini-term and for each one
of the pathologies. C are the measurements without
pathology and P
1
,P
2
,P
3
,P
4
,P
5
are the measurements
obtained for each of the pathologies analyzed.
In our previous work, (E. Garcia et al., 2018),
the experimental samples were analyzed to under-
stand how the pathologies affect the cycle time and to
generate rules that allow us to determine the pathol-
ogy. The statistical tests used in our previous work
were ANOVA, Shaphiro-Wilk, Tukey, Levene, χ
2
tests and variance contrast hypothesis. A summary
of the statistical rules obtained is shown in Table 1
where the first two columns show the rules that clas-
sify mean and variance values according to the pathol-
ogy. Column four shows threshold values to deter-
mine whether there are pathologies or not and the
last column shows extra rules like for instance, when
pathology 4 occurs, the data do not pass the normality
test.
By means of these rules, a Bayesian model that
mixes the gaussians was proposed in our previous
work, (M. Alacreu et al., 2018), to determine which
pathology occurs in real-time.
3 GOAL OF THE PAPER
Industry 4.0 is a current trend and data exchange
in manufacturing technologies. It includes cyber-
physical systems, the internet of things and cloud
computing creating what has been called a ”smart
factory”. Following this tendency, the ideal way for
maintenance prognosis would be to do it continuously
and automatically. However, as indicated in (R. Ah-
mad and S. Kamaruddini, 2012) it is very expensive
since many sensors and devices are needed to carry
it out. The most used sensors to perform the main-
tenance prognosis are: vibration, noise, temperature,
pressure, flow, etc. Fortunately, as we have explained
in (E. Garcia et al., 2018), when components have an
advanced lifetime, it affects the cycle time but with an
important advantage: the mini-term is easy and cheap
to be installed than other sensors. It is cheap because
no additional hardware installation is required to mea-
sure the sub-cycle time, just the use of the PLC and
sensors installed for the automated production pro-
cess, and it is easy because we only need to code extra
timers into the PLC.
The results presented in (E. Garcia et al., 2018)
generated a great expectation in Ford Motor Com-
pany, allowing us to analyze in depth the capabili-
ties of the mini-term for failure prognosis. Section 4
shows the setup to measure mini-terms at Ford plant in
Almussafes factory, the so-called Mini-term 4.0. The
system was switched on in April 2018 and began to
monitor thousands of mini-terms. Section 5 shows
a summary of the different kinds of pathologies that
through the mini-terms we have been able to detect
since the system was switched on. In section 6 we
can see the conclusions showing special emphasys on
future works.
4 MINI-TERM 4.0 DEFINITION
4.1 Machine Learning Techniques
Pattern recognition and machine learning can be
viewed as two facets of the same field and they only
depend on the field of application. The pattern recog-
nition term is mainly used in engineering meanwhile
the machine learning term is mainly used in computer
science problems. In any case, it can be defined as
a program or an algorithm that is capable of learn-
ing with minimum or no additional support (Webb
ICINCO 2019 - 16th International Conference on Informatics in Control, Automation and Robotics
182