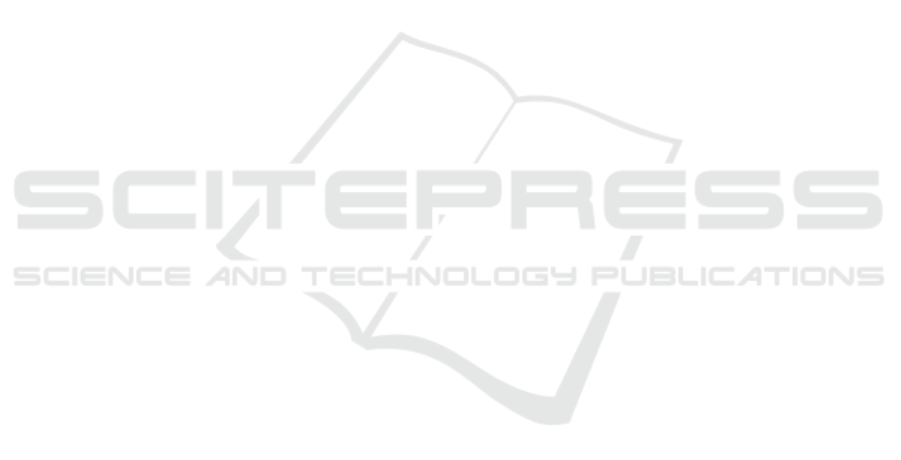
demonstrated event-related desynchronization in both
β and µ frequency bands, which is expected result
considering that the ”nature” of the KI pattern is more
related to the motor action (Neuper et al., 2005; Guil-
lot et al., 2009). On the contrary, the event-related
synchronization was found in the VI subjects.
Then, we enhanced our results using artificial neu-
ral network. First, we classified unoptimized magni-
toencephalographical dataset with 102 channels and
achieved up to 70% accuracy using the low-frequency
filter with cutoff below 15 Hz. The same level of ac-
curacy was achieved with KI by applying the high-
pass filter with cutoff above 30 Hz. Finally, in or-
der to see how data optimization affects the artificial
neural network performance, we selected 14 channels
over the motor-related area and revealed no signifi-
cant changes for KI. On the other hand, the VI group
shown the possibility to enhance artificial neural net-
work performance with particular set of channels and
frequency cutoffs.
Thus, despite the fact that KI is easier to clas-
sify and the KI pattern is more pronounced in time-
frequency structure of the MEG signal, there is a
possibility to achieve comparable results with the
VI. Since the VI mode is more common for un-
trained subjects, we suggest that obtained results can
be useful for implementation of the artificial intelli-
gence systems for MI patterns classification for brain-
computer interfaces, exoskeletons, wheelchairs and
other robotics devices.
ACKNOWLEDGEMENTS
This work has been supported by Russian Science
Foundation (Grant 17-72-30003).
REFERENCES
Arroyo, S., Lesser, R. P., Gordon, B., Uematsu, S., Jackson,
D., and Webber, R. (1993). Functional significance of
the mu rhythm of human cortex: an electrophysiologic
study with subdural electrodes. Electroencephalogra-
phy and clinical neurophysiology, 87(3):76–87.
Callow, N., Jiang, D., Roberts, R., and Edwards, M. G.
(2017). Kinesthetic imagery provides additive benefits
to internal visual imagery on slalom task performance.
Journal of Sport and Exercise Psychology, 39(1):81–
86.
Defays, D. (1977). An efficient algorithm for a complete
link method. The Computer Journal, 20(4):364–366.
Duann, J.-R. and Chiou, J.-C. (2016). A Comparison
of Independent Event-Related Desynchronization Re-
sponses in Motor-Related Brain Areas to Movement
Execution, Movement Imagery, and Movement Ob-
servation. PLOS ONE, 11(9):1–16.
Ferreira, A., Celeste, W. C., Cheein, F. A., Bastos-Filho,
T. F., Sarcinelli-Filho, M., and Carelli, R. (2008).
Human-machine interfaces based on EMG and EEG
applied to robotic systems. Journal of NeuroEngineer-
ing and Rehabilitation, 5(1):10.
Filgueiras, A., Conde, E. F. Q., and Hall, C. R. (2018).
The neural basis of kinesthetic and visual imagery in
sports: an ale meta- analysis. Brain imaging and be-
havior, 12(5):1513–1523.
Fraley, C. and Raftery, A. E. (1998). How many clusters?
which clustering method? answers via model-based
cluster analysis. The computer journal, 41(8):578–
588.
Frolov, A. A., Mokienko, O., Lyukmanov, R., Biryukova,
E., Kotov, S., Turbina, L., Nadareyshvily, G., and
Bushkova, Y. (2017). Post-stroke rehabilitation train-
ing with a motor-imagery-based brain-computer inter-
face (BCI)-controlled hand exoskeleton: a random-
ized controlled multicenter trial. Frontiers in neuro-
science, 11:400.
Graimann, B., Huggins, J. E., Levine, S. P., and
Pfurtscheller, G. (2004). Toward a direct brain
interface based on human subdural recordings and
wavelet-packet analysis. IEEE Transactions on
Biomedical Engineering, 51(6):954–962.
Guillot, A., Collet, C., Nguyen, V. A., Malouin, F.,
Richards, C., and Doyon, J. (2009). Brain activity dur-
ing visual versus kinesthetic imagery: an fmri study.
Human brain mapping, 30(7):2157–2172.
Hanakawa, T. (2016). Organizing motor imageries. Neuro-
science research, 104:56–63.
Jones, S. R., Kerr, C. E., Wan, Q., Pritchett, D. L.,
H
¨
am
¨
al
¨
ainen, M., and Moore, C. I. (2010). Cued spa-
tial attention drives functionally relevant modulation
of the mu rhythm in primary somatosensory cortex.
Journal of Neuroscience, 30(41):13760–13765.
K
¨
ubler, A., Nijboer, F., Mellinger, J., Vaughan, T. M.,
Pawelzik, H., Schalk, G., McFarland, D. J., Bir-
baumer, N., and Wolpaw, J. R. (2005). Patients with
ALS can use sensorimotor rhythms to operate a brain-
computer interface. Neurology, 64(10):1775–1777.
La Touche, R., Grande-Alonso, M., Cuenca-Mart\’\inez,
F., G
´
onz
´
alez-Ferrero, L., Suso-Mart\’\i, L., and
Paris-Alemany, A. (2018). Diminished Kinesthetic
and Visual Motor Imagery Ability in Adults With
Chronic Low Back Pain. PM&R.
Maksimenko, V. A., Pavlov, A., Runnova, A. E., Nedaivo-
zov, V., Grubov, V., Koronovskii, A. A., Pchelintseva,
S. V., Pitsik, E., Pisarchik, A. N., and Hramov, A. E.
(2018). Nonlinear analysis of brain activity, associated
with motor action and motor imaginary in untrained
subjects. Nonlinear Dynamics, 91(4):2803–2817.
McFarland, D. J. and Wolpaw, J. R. (2005). Sensorimotor
rhythm-based brain-computer interface (BCI): feature
selection by regression improves performance. IEEE
Transactions on Neural Systems and Rehabilitation
Engineering, 13(3):372–379.
ICINCO 2019 - 16th International Conference on Informatics in Control, Automation and Robotics
194