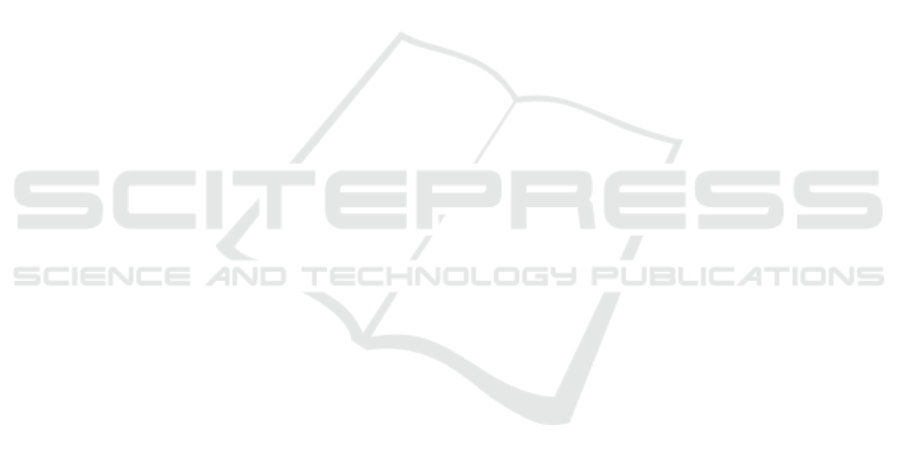
is low, having in some cases a lower T S value in the
cIoC compared to one of the sIoC (i.e., S
7
).
It is important to note that among all the criteria
used in the T S computation, the completeness (i.e.,
C
p
) is the criterion that affects the most the final re-
sult. Whereas, all other criteria are adding individual
values to the T S, the completeness criterion is mul-
tiplying to the overall addition, affecting to a higher
level the T S results of single or composed IoCs.
7 CONCLUSION
This paper presents ET IP, an enriching threat intel-
ligence platform, as an extended import, quality as-
sessment processes and information sharing capabil-
ities in current TIPs. The proposed platform gath-
ers and processes structured information from exter-
nal sources (e.g., OSINT sources) and from the moni-
tored infrastructure. The platform is composed of two
main modules: (i) a Composed IoC Module, in charge
of collecting, normalizing, processing and aggregat-
ing IoCs from OSINT feeds; and (ii) a Context Aware
Intelligence Sharing Module, able to correlate, assess
and share static and real time information with data
obtained from multiple OSINT sources.
The ETIP platform computes a Threat Score (T S)
associated to each IoC before sharing it with both in-
ternal monitoring systems and tools (e.g., SIEMs) and
trusted external parties. Enriched IoCs will contain a
threat score that will enable SOC analysts to priori-
tize the analysis of incidents. The Threat Score eval-
uates heuristics with two types of weights: (i) indi-
vidual weights assigned to every attribute (e.g., rele-
vance, accuracy, variety, etc.); and (ii) global weight
(i.e., completeness criterion) assigned to the heuristic.
The higher the T S value, the more reliable the IoC.
Thus, as the T S value approaches to zero, the IoC can
be considered as poor, incomplete and/or not reliable
with a very low priority level.
Future work will concentrate in developing new
attributes to enrich the threat score analysis, improv-
ing the quality of the refined threat intelligence to be
shared, providing not only the final threat score, but
also detailed information about each single criterion
used in the evaluation of the score itself, which in
turn helps to improve threat detection and incident re-
sponse.
ACKNOWLEDGMENT
The research in this paper has received funding from
the EC through funding of DiSIEM project, ref.
project H2020-700692, NeCS project, ref. project
H2020-675320 and LASIGE Research Unit, ref.
UID/CEC/00408/2019.
REFERENCES
Accenture (2017). Cost of cyber crime study. Online.
CEA (2018). The cost of malicious cyber activity to the u.s.
economy. Online Report.
Dalziel, H. (2014). How to define and build an effective cy-
ber threat intelligence capability. In Syngress, eBook.
ENISA (2015). Actionable information for security incident
response. In Online Technical Paper.
ENISA (2017). Exploring the opportunities and limitations
of current threat intelligence platforms.
Liao, X., Yuan, K., Wang, X., Li, Z., Xing, L., and Beyah.,
R. (2016). Acing the ioc game: Toward automatic
discovery and analysis of open-source cyber threat in-
telligence. In ACM SIGSAC Conference on Computer
and Communications Security, pages 755–766.
Mavroeidis, V. and Bromander, S. (2017). Cyber threat in-
telligence model: An evaluation of taxonomies, shar-
ing standards, and ontologies within cyber threat in-
telligence. In Intelligence and Security Informatics
Conference, pages 91–98. IEEE.
Owen, T. (2015). Threat intelligence & siem. In Masters
Research Project, Lewis University.
Ravana, S. D. and Moffat, A. (2009). Score aggregation
techniques in retrieval experimentation. In Twentieth
Australasian Database Conference.
Sabottke, C., Suciu, O., and Dumitras, T. (2015). Vulnera-
bility disclosure in the age of social media: exploiting
twitter for predicting real-world exploits. In In 24th
USENIX Security Symposium, pages 1041–1056.
Sauerwein, C., Sillaber, C., Mussmann, A., and Breu, R.
(2017). Threat intelligence sharing platforms: An ex-
ploratory study of software vendors and research per-
spectives. In Conference on Wirtschaftsinformatik.
Sillaber, C., Sauerwein, C., Mussmann, A., and Breu, R.
(2016). Data quality challenges and future research
directions in threat intelligence sharing practice. In
ACM on Workshop on Information Sharing and Col-
laborative Security, pages 65–70. ACM.
Skopik, F., Settanni, G., and Fiedler, R. (2016). A prob-
lem shared is a problem halved: A survey on the di-
mensions of collective cyber defense through security
information sharing. computers & security, 60:154–
176.
ThreatConnect (Accessed February 2018). Threat intel-
ligence platforms. everything you’ve ever wanted to
know but didn’t know to ask. In Ebook.
Torra, V. and Narukawa, Y. (2007). Modeling decisions:
Information fusion and aggregation operators. In
Springer-Verlag Berlin Heidelberg.
Tounsi, W. and Rais, H. (2018). A survey on technical threat
intelligence in the age of sophisticated cyber attacks.
In Computers & Security, volume 72, pages 212–233.
Ventures, C. (2017). 2017 cybercrime report. Online.
SECRYPT 2019 - 16th International Conference on Security and Cryptography
48