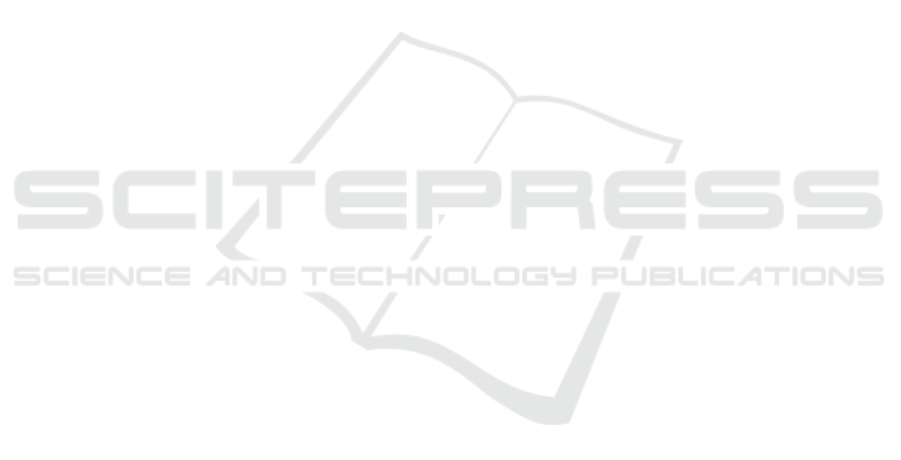
PredM the exact reproduction of patterns from real
industrial factories is not required, as the goal of ML
methods is to find patterns according to the produc-
tion environment at hand. Thus, we are confident
that the developed FT factory model is an appropri-
ate means to perform laboratory research on ML in a
well controlled environment. We also plan to publish
the gained data sets at http://IoT.uni-trier.de so that
they could be used by other researchers as well.
REFERENCES
Abele, E., Metternich, J., Tisch, M., Chryssolouris, G.,
Sihn, W., ElMaraghy, H., Hummel, V., and Ranz, F.
(2015). Learning factories for research, education,
and training. Procedia CiRp, 32:1–6.
Alzantot, M., Chakraborty, S., and Srivastava, M. (2017).
Sensegen: A deep learning architecture for synthetic
sensor data generation. In Pervasive Computing and
Communications Workshops (PerCom Workshops),
2017 IEEE International Conference on, pages 188–
193. IEEE.
Angione, G., Barbosa, J., Gosewehr, F., Leit
˜
ao, P., Massa,
D., Matos, J., Peres, R. S., Rocha, A. D., and Wer-
mann, J. (2017). Integration and deployment of a dis-
tributed and pluggable industrial architecture for the
perform project. Procedia Manufacturing, 11:896–
904.
Babu, G. S., Zhao, P., and Li, X.-L. (2016). Deep convo-
lutional neural network based regression approach for
estimation of remaining useful life. In International
conference on database systems for advanced appli-
cations, pages 214–228. Springer.
Dua, D. and Graff, C. (2019). UCI machine learning repos-
itory.
Eker,
¨
O. F., Camci, F., and Jennions, I. K. (2012). Ma-
jor challenges in prognostics: study on benchmark-
ing prognostic datasets. In Proceedings of the Annual
Conference of the PHM Society. PHM Society.
Esteban, C., Hyland, S. L., and R
¨
atsch, G. (2017). Real-
valued (medical) time series generation with recurrent
conditional gans. arXiv preprint arXiv:1706.02633.
Estrada, R. and Ruiz, I. (2016). Big data smack. Apress,
Berkeley, CA.
Hahsler, M., Bola
˜
nos, M., and Forrest, J. (2017). Intro-
duction to stream: An extensible framework for data
stream clustering research with r. Journal of Statisti-
cal Software, Articles, 76(14):1–50.
Heged
˝
us, C., Varga, P., and Moldov
´
an, I. (2018). The man-
tis architecture for proactive maintenance. In 2018 5th
International Conference on Control, Decision and
Information Technologies (CoDIT), pages 719–724.
IEEE.
Jain, S., Shao, G., and Shin, S.-J. (2017). Manufactur-
ing data analytics using a virtual factory representa-
tion. International Journal of Production Research,
55(18):5450–5464.
Jia, X., Huang, B., Feng, J., Cai, H., and Lee, J. (2018). A
review of phm data competitions from 2008 to 2017.
In Proceedings of the Annual Conference of the PHM
Society.
Khan, S. and Yairi, T. (2018). A review on the application of
deep learning in system health management. Mechan-
ical Systems and Signal Processing, 107:241–265.
Klein, P. and Bergmann, R. (2018). Data Generation with
a Physical Model to Support Machine Learning Re-
search for Predictive Maintenance. In Lernen. Wis-
sen. Daten. Analysen. (LWDA 2018). CEUR Work-
shop Proceedings.
Kreps, J., Narkhede, N., Rao, J., et al. (2011). Kafka: A
distributed messaging system for log processing. In
SIGMOD Workshop on Networking Meets Databases.
Lang, S., Reggelin, T., Jobran, M., and Hofmann, W.
(2018). Towards a modular, decentralized and digital
industry 4.0 learning factory. In 2018 Sixth Interna-
tional Conference on Enterprise Systems (ES), pages
123–128. IEEE.
Lee, J., Kao, H.-A., and Yang, S. (2014). Service innovation
and smart analytics for industry 4.0 and big data envi-
ronment. Procedia CIRP, 16:3 – 8. Product Services
Systems and Value Creation. Proceedings of the 6th
CIRP Conference on Industrial Product-Service Sys-
tems.
Nandi, S., Toliyat, H. A., and Li, X. (2005). Condition
monitoring and fault diagnosis of electrical motors —
a review. IEEE transactions on energy conversion,
20(4):719–729.
Nectoux, P., Gouriveau, R., Medjaher, K., Ramasso, E.,
Chebel-Morello, B., Zerhouni, N., and Varnier, C.
(2012). Pronostia: An experimental platform for bear-
ings accelerated degradation tests. In IEEE Interna-
tional Conference on Prognostics and Health Man-
agement, PHM’12, pages 1–8. IEEE Catalog Number:
CPF12PHM-CDR.
Saxena, A., Goebel, K., Simon, D., and Eklund, N. (2008).
Damage propagation modeling for aircraft engine run-
to-failure simulation. In Prognostics and Health Man-
agement, 2008. PHM 2008. International Conference
on, pages 1–9. IEEE.
Selcuk, S. (2017). Predictive maintenance, its implementa-
tion and latest trends. Proceedings of the Institution of
Mechanical Engineers, Part B: Journal of Engineer-
ing Manufacture, 231(9):1670–1679.
Simons, S., Ab
´
e, P., and Neser, S. (2017). Learning in the
autfab–the fully automated industrie 4.0 learning fac-
tory of the university of applied sciences darmstadt.
Procedia Manufacturing, 9:81–88.
von Birgelen, A., Buratti, D., Mager, J., and Niggemann,
O. (2018). Self-organizing maps for anomaly local-
ization and predictive maintenance in cyber-physical
production systems. Procedia CIRP, 72:480 – 485.
51st CIRP Conference on Manufacturing Systems.
Yuan, M., Wu, Y., and Lin, L. (2016). Fault diagnosis and
remaining useful life estimation of aero engine using
lstm neural network. In Aircraft Utility Systems (AUS),
IEEE International Conference on, pages 135–140.
IEEE.
Zhang, D., Xu, B., and Wood, J. (2016). Predict failures in
production lines: A two-stage approach with cluster-
Generation of Complex Data for AI-based Predictive Maintenance Research with a Physical Factory Model
49