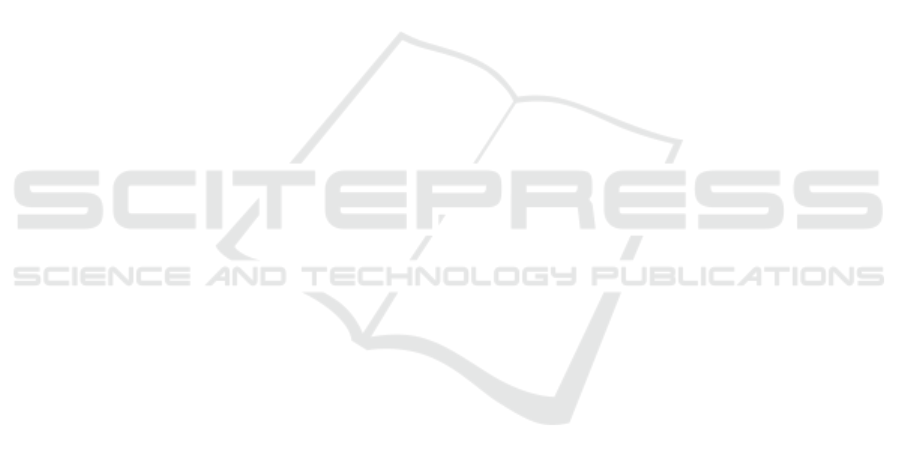
REFERENCES
Y. Sun, B. Leng and W. Guan, 2015. A novel wavelet-SVM
short-time passenger flow prediction in Beijing sub-
way system. Neurocomputing. 166, 109-121
J.D. Ort
´
uzar and L.G. Willumsen, 1996. Modelling Trans-
port, 2nd Edition. Wiley.
Y. Jia, P. He, S. Liu and L. Cao, 2016. A combined fore-
casting model for passenger flow based on GM and
ARMA. International Journal of Hybrid Information
Technology. 9/2, p. 215–226.
Y. Bai, Z. Sun, B. Zeng, J. Deng and C. Li, 2017. A multi-
pattern deep fusion model for short-term bus passen-
ger flow forecasting. Applied Soft Computing. 58, p.
669–680.
S. Zhao, T. Ni, Y. Wang and X. Gao, 2011 A new approach
to the prediction of passenger flow in a transit system.
Computers and Mathematics with Applications. 61, p.
1968–1974.
L. Liu and R. Chen., 2017. A novel passenger flow predic-
tion model using deep learning methods. Transporta-
tion Research Part C. 84, p. 74–91.
J. Roos, G. Gavin and S. Bonnevay, 2016. A dynamic
Bayesian network approach to forecast short-term ur-
ban rail passenger flows with incomplete data. Trans-
portation Research Procedia. 26, p. 53–61.
S. Haar and S. Theissing, 2015. A hybrid-dynamic model
for passenger-flow in transportation systems. IFAC-
PapersOnLine. 48-27, p. 236–241.
L. Sun, Y. Lu, J. Jin, D. Lee and K. Axhausen, 2015. An
integrated Bayesian approach for passenger flow as-
signment in metro networks. Transportation Research
Part C. 52, p. 116–131.
Y. Li, X. Wang, S. Sun, X. Ma and G. Lu, 2017. Forecast-
ing short-term subway passenger flow under special
events scenarios using multiscale radial basis function
networks. Transportation Research Part C. 77, p. 306–
328.
Z. Ma, J. Xing, M. Mesbad and L. Ferreira, 2014. Predict-
ing short-term bus passenger demand using a pattern
hybrid approach. Transportation Research Part C. 39,
p. 148–163.
M. Milenkovi
ˇ
c, L.
ˇ
Svadlenka, V. Melichar, N. Bojovi
ˇ
c
and Z. Avramovi
ˇ
c., 2016. SARIMA modelling ap-
proach for railway passenger flow forecasting. Trans-
port. 2016, p. 1–8.
C. Zhou, P. Dai and R. Li., 2013. The passenger demand
prediction model on bus networks. In: Proceedings of
13th International Conference on Data Mining Work-
shops. p. 1069-1076.
M. Chen and Y. Wei., 2011. Exploring time variants for
short-term passenger flow. Journal of Transport Ge-
ography. 19, p. 488-498.
T. Tsai, C. Lee and C. Wei, 2009. Neural network based
temporal feature models for short-term railway pas-
senger demand forecasting. Expert Systems with Ap-
plications. 36, p. 3728–3736.
X. Jiang, L. Zhang and X. Chen, 2014. Short-term fore-
casting of high-speed rail demand: A hybrid approach
combining ensemble empirical mode decomposition
and gray support vector machine with real-world ap-
plications in China. Transportation Research Part C.
44, p. 110–127.
S. Haar and S. Theissing, 2016. Forecasting passenger loads
in transportation networks. Electronic Notes in Theo-
retical Computer Science. 327, p. 49–69.
V. Peterka. Bayesian system identification, 1981. In: Trends
and Progress in System Identification, P. Eykhoff, Ed.
Oxford: Pergamon Press, p. 239–304.
M. K
´
arn
´
y, J. Kadlec, E.L. Sutanto, 1998. Quasi-Bayes es-
timation applied to normal mixture. Preprints of the
3rd European IEEE Workshop on Computer-Intensive
Methods in Control and Data Processing, Eds: J.
Roj
´
ı
ˇ
cek, M. Vale
ˇ
ckov
´
a, M. K
´
arn
´
y, K. Warwick, p. 77–
82, CMP ’98 /3./, Prague, CZ, 07.09.1998–09.09.
M. K
´
arn
´
y, J. B
¨
ohm, T. V. Guy, L. Jirsa, I. Nagy, P. Nedoma,
and L. Tesa
ˇ
r, 2006. Optimized Bayesian Dynamic Ad-
vising: Theory and Algorithms. Springer, London.
I. Nagy, E. Suzdaleva, 2017. Algorithms and Programs
of Dynamic Mixture Estimation. Unified Approach
to Different Types of Components, SpringerBriefs in
Statistics. Springer International Publishing, 2017.
P. Samaras, A. Fachantidis and G. Tsoumakas, 2015. A pre-
diction model of passenger demand using AVL and
APC Data from a bus fleet. 19th Panhellenic Confer-
ence on Informatics. p. 129–134.
I. Nagy, E. Suzdaleva, M. K
´
arn
´
y and T. Mlyn
´
a
ˇ
rov
´
a, 2011.
Bayesian estimation of dynamic finite mixtures. Int.
Journal of Adaptive Control and Signal Processing.
25(9), p. 765–787.
S. Yang and G. Berdine, 2015. Poisson regression. The
Southwest Respiratory and Critical Care Chronicles.
3(9), 2015, p. 61–64.
I. Nagy, E. Suzdaleva, P. Pecherkov
´
a, 2016. Comparison
of Various Definitions of Proximity in Mixture Esti-
mation. Proceedings of the 13th International Con-
ference on Informatics in Control, Automation and
Robotics (ICINCO). Lisbon, Portugal, July, 29 – 31,
p. 527–534.
Pu
ˇ
sman V., 2013. Optimalizace syst
´
emu organizace ve
ˇ
rejn
´
e
hromadn
´
e dopravy. The dissertation thesis.
ICINCO 2019 - 16th International Conference on Informatics in Control, Automation and Robotics
624