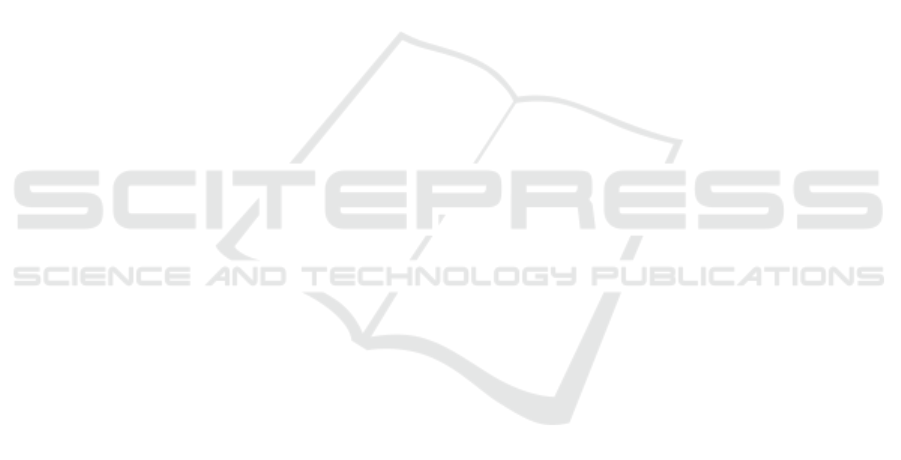
9 CONCLUSIONS
Product positioning is key in targeting the right
consumers. Commercial organisations continuously
monitor their product positioning by gathering data
online and offline. Products mispositioning however,
could jeopardise the marketing strategy of a company
and should be avoided. Validating strategies early in
the product release cycle constitute a vital process for
effective sales performance. Therefore, companies in
addition to other information sources, should also
utilise data from the blogosphere to understand
customers’ opinions in real time and accordingly
respond to their needs (Al-Obeidat, F., Spencer, B.
and Kafeza, E., 2018). Data mining can help
enterprises resolve marketing issues and improve
product positioning through quicker analysis of
online consumers opinions.
This work presented a technique for evaluating
product positioning using eWOM analysis. An
application of eWOM analysis was also presented, for
the marketing strategy of two Huawei smartphones.
Limitations of this work lie in the small sample size
which concentrated on specific geographical regions.
For future work the authors are considering
expanding on the methodology to evaluate the impact
of marketing strategies using more sophisticated
sentiment analysis techniques with less false positives
and false negatives rates and hence require less
manual evaluation.
REFERENCES
Jung, J. J., 2008. Taxonomy alignment for interoperability
between heterogeneous virtual organizations. Expert
Systems with Applications, 34(4), pp.2721-2731.de
Chernatony, L. (2000). Succeeding with brands on the
Internet. Brand Management, 8(3), 186-195.
Forbes, 2018 (https://www.forbes.com/companies/twitter/)
Retrieved 10 April 2018
Statista, 2018 (https://www.statista.com/statistics/282087/
number-of-monthly-active-twitter-users/) Retrieved 13
April 2018
Huawei, 2018 (http://www.huawei.com/en/about-huawei/
corporate-information/milestone) Retrieved 14 April
2018
Sagolla, D. (2009). 140 characters: A style guide for the
short form. Hoboken: Wiley.
Jansen, B. J., Zhang, M., Sobel, K., & Chowdhury, A.
(2009). Twitter power: Tweets as electronic word of
mouth. Journal of the American Society for Information
Science, 60(11), 2169-2188.
Zhang, M., Jansen, B. J. and Chowdhury, A., 2011.
Business engagement on Twitter: a path analysis.
Electronic Markets, 21(3), p.161.
Wauters, R. (2009). Twitter spawned 50,000 apps to date,
will open up firehose for more. Retrieved January 6,
2010, from http://www. techcrunch.com/2009/12/09/
twitter-le-web-2009/.
Keller, E. (2007). Unleashing the power of word of mouth:
Creating brand advocacy to drive growth. Journal of
Advertising Research, 47(4), 448–452.
Chamlertwat, W., Bhattarakosol, P., Rungkasiri, T. and
Haruechaiyasak, C., 2012. Discovering Consumer
Insight from Twitter via Sentiment Analysis. J. UCS,
18(8), pp. 973-992.
Lei, N. and Moon, S. K., 2015. A Decision Support System
for market-driven product positioning and design.
Decision Support Systems, 69, pp.82-91.
Jung, J. J., 2012. Evolutionary approach for semantic-based
query sampling in large-scale information sources.
Information Sciences, 182(1), pp.30-39.
Ferreira, A., 2011. Android OS changes smartphone life
cycle. The Vista, February.
Mostafa, M.M., 2013. More than words: Social networks’
text mining for consumer brand sentiments. Expert
Systems with Applications, 40(10), pp.4241-4251.
Olobatuyi, M. E., 2006. A user’s guide to path analysis.
Lanham: University Press of America, Inc. p.32.
Thelwall, M., Buckley, K. and Paltoglou, G., 2011. Senti-
ment in Twitter events. Journal of the Association for
Information Science and Technology, 62(2), pp.406-418.
Huang, J., Thornton, K. M. and Efthimiadis, E. N., 2010,
June. Conversational tagging in twitter. In Proceedings
of the 21st ACM conference on Hypertext and
hypermedia (pp. 173-178). ACM.
Honey, C. and Herring, S. C., 2009, January. Beyond
microblogging: Conversation and collaboration via
Twitter. In System Sciences, 2009. HICSS'09. 42nd
Hawaii International Conference on (pp. 1-10). IEEE.
Franke, N. and Piller, F. T., 2003. Key research issues in
user interaction with user toolkits in a mass
customisation system. International Journal of
Technology Management, 26(5-6), pp.578-599.
Zhu, F. and Zhang, X., 2010. Impact of online consumer
reviews on sales: The moderating role of product and
consumer characteristics. Journal of marketing, 74(2),
pp.133-148.
Lilien, G. L. and Rangaswamy, A., 2003. New Product and
Brand Management: Marketing Engineering
Applications. Prentice Hall.
Boyd, D., Golder, S. and Lotan, G., 2010, January. Tweet,
tweet, retweet: Conversational aspects of retweeting on
twitter. In System Sciences (HICSS), 2010 43rd Hawaii
International Conference on (pp. 1-10). IEEE.
Petiot, J. F. and Grognet, S., 2006. Product design: a vectors
field-based approach for preference modelling. Journal
of engineering design, 17(03), pp.217-233.
Kwong, C. K., Luo, X. G. and Tang, J. F., 2011. A
methodology for optimal product positioning with
engineering constraints consideration. International
Journal of Production Economics, 132(1), pp.93-100.
Pang, B. and Lee, L., 2008. Opinion mining and sentiment
analysis. Foundations and Trends® in Information
Retrieval, 2(1–2), pp.1-135.
ICEIS 2019 - 21st International Conference on Enterprise Information Systems
272