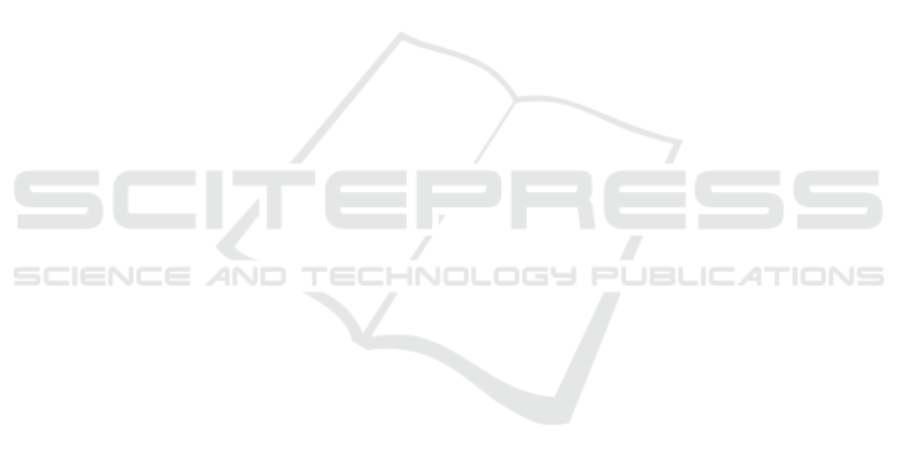
veloped to pick and place, and convey an object in the
domestic environment effectively. Based on the re-
sults, the three strategies have their own advantages at
the different table heights. Therefore, the intelligent
strategy selection system can be applied for domestic
environments that have different table heights.
Actually, the current system could be used to de-
tect, cluster, and extract simple household objects
such as bottles, boxes, etc. However, various objects
that are different in shape exist in the domestic en-
vironment. Therefore, the 3D centroid of an object
would not be able to grasp it. For this reason, we will
develop a grasp pose algorithm for a variety of house-
hold objects with our strategies to save time (Redmon
and Angelova, 2015). In addition, a deep learning-
based approach for extracting grasping point could
be considered to obtain more accurate performance
(Lenz et al., 2015; Levine et al., 2016).
ACKNOWLEDGEMENT
The work described was supported by the Robot-
Era and ACCRA project, respectively founded by
the European Community’s Seventh Framework Pro-
gramme FP7-ICT-2011 under grant agreement No.
288899 and the Horizon 2020 Programme H2020-
SCI-PM14-2016 under grant agreement No. 738251.
REFERENCES
Cavallo, F., Limosani, R., Manzi, A., Bonaccorsi, M., Es-
posito, R., Di Rocco, M., Pecora, F., Teti, G., Saffiotti,
A., and Dario, P. (2014). Development of a socially
believable multi-robot solution from town to home.
Cognitive Computation, 6(4):954–967.
Chitta, S., Sucan, I., and Cousins, S. (2012). Moveit![ros
topics]. IEEE Robotics & Automation Magazine,
19(1):18–19.
Ciocarlie, M., Hsiao, K., Jones, E. G., Chitta, S., Rusu,
R. B., and S¸ucan, I. A. (2014). Towards reliable grasp-
ing and manipulation in household environments. In
Experimental Robotics, pages 241–252. Springer.
Dogar, M. and Srinivasa, S. (2011). A framework for push-
grasping in clutter. Robotics: Science and systems VII,
1.
Fromm, T. and Birk, A. (2016). Physics-based damage-
aware manipulation strategy planning using scene dy-
namics anticipation. In Intelligent Robots and Systems
(IROS), 2016 IEEE/RSJ International Conference on,
pages 915–922. IEEE.
Hershberger, D., Gossow, D., and Faust, J. (2011). Rviz.
Kitaev, N., Mordatch, I., Patil, S., and Abbeel, P. (2015).
Physics-based trajectory optimization for grasping in
cluttered environments. In Robotics and Automa-
tion (ICRA), 2015 IEEE International Conference on,
pages 3102–3109. IEEE.
Leeper, A. E., Hsiao, K., Ciocarlie, M., Takayama, L., and
Gossow, D. (2012). Strategies for human-in-the-loop
robotic grasping. In Proceedings of the seventh an-
nual ACM/IEEE international conference on Human-
Robot Interaction, pages 1–8. ACM.
Lenz, I., Lee, H., and Saxena, A. (2015). Deep learning for
detecting robotic grasps. The International Journal of
Robotics Research, 34(4-5):705–724.
Levine, S., Finn, C., Darrell, T., and Abbeel, P. (2016). End-
to-end training of deep visuomotor policies. Journal
of Machine Learning Research, 17(39):1–40.
Ozg
¨
ur, A. and Akın, H. L. Planning for tabletop clutter
using affordable 5-dof manipulator.
Parasuraman, R., Sheridan, T. B., and Wickens, C. D.
(2000). A model for types and levels of human in-
teraction with automation. IEEE Transactions on sys-
tems, man, and cybernetics-Part A: Systems and Hu-
mans, 30(3):286–297.
Pitzer, B., Styer, M., Bersch, C., DuHadway, C., and
Becker, J. (2011). Towards perceptual shared auton-
omy for robotic mobile manipulation. In Robotics and
Automation (ICRA), 2011 IEEE International Confer-
ence on, pages 6245–6251. IEEE.
Redmon, J. and Angelova, A. (2015). Real-time grasp
detection using convolutional neural networks. In
Robotics and Automation (ICRA), 2015 IEEE Inter-
national Conference on, pages 1316–1322. IEEE.
Rusu, R. B. and Cousins, S. (2011). 3d is here: Point cloud
library (pcl). In Robotics and automation (ICRA),
2011 IEEE International Conference on, pages 1–4.
IEEE.
Sak, H., Senior, A., Rao, K., Beaufays, F., and Schalkwyk,
J. (2015). Google voice search: faster and more accu-
rate. Google Research blog.
Schraft, R., Schaeffer, C., and May, T. (1998). Care-o-
bot/sup tm: the concept of a system for assisting el-
derly or disabled persons in home environments. In
Industrial Electronics Society, 1998. IECON’98. Pro-
ceedings of the 24th Annual Conference of the IEEE,
volume 4, pages 2476–2481. IEEE.
Stilman, M., Schamburek, J.-U., Kuffner, J., and Asfour, T.
(2007). Manipulation planning among movable obsta-
cles. In Robotics and Automation, 2007 IEEE Inter-
national Conference on, pages 3327–3332. IEEE.
Torta, E., Oberzaucher, J., Werner, F., Cuijpers, R. H., and
Juola, J. F. (2012). Attitudes towards socially assis-
tive robots in intelligent homes: results from labora-
tory studies and field trials. Journal of Human-Robot
Interaction, 1(2):76–99.
Trevor, A. J., Gedikli, S., Rusu, R. B., and Christensen,
H. I. (2013). Efficient organized point cloud segmen-
tation with connected components. Semantic Percep-
tion Mapping and Exploration (SPME).
Integration of an Autonomous System with Human-in-the-Loop for Grasping an Unreachable Object in the Domestic Environment
315