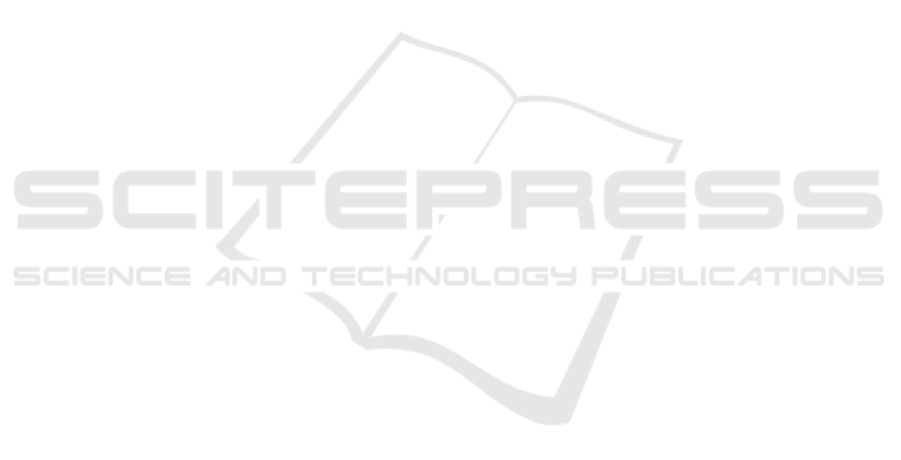
tion (chiller supply and return temperatures and water
temperature). Consequently, the anomaly detection
procedure could be applied to these variables, mak-
ing this process more simple and efficient in terms
of time and computation requirements. Alternatively,
when we are interested on controlling just one vari-
able and this is characterized by a functional nature,
a FDA methodology for control charts has been pro-
posed and applied to the HVAC energy consumption
daily curves. Confidence bands have been estimated
in the calibration stage, allowing us to monitor the
consumption curves of new days and decide if they
correspond to anomalies in the system. This statistical
approach is based on functional data depth calculation
and the application of rank control charts.
ACKNOWLEDGEMENTS
This research/work of Salvador Naya, Javier Tarr
´
ıo-
Saavedra and Rub
´
en Fern
´
andez-Casal have been sup-
ported by MINECO grants MTM2014-52876-R and
MTM2017-82724-R, and by the Xunta de Galicia
(Grupos de Referencia Competitiva ED431C-2016-
015 and Centro Singular de Investigaci
´
on de Galicia
ED431G/01 2016-19), all of them through the ERDF.
The work of Carlos Erias, Ver
´
onica Bol
´
on and Javier
Tarr
´
ıo has been also developed in the framework of
eCOAR project (PC18/03) of CITIC. The research of
Miguel Flores has been partially supported by Grant
PII-DM-002-2016 of Escuela Polit
´
ecnica Nacional of
Ecuador.
REFERENCES
Barbeito, I., Zaragoza, S., Tarr
´
ıo-Saavedra, J., and Naya,
S. (2017). Assessing thermal comfort and energy ef-
ficiency in buildings by statistical quality control for
autocorrelated data. Applied energy, 190:1–17.
Bolon-Canedo, V., Sanchez-Maro no, N., and Alonso-
Betanzos, A. (2017). Artificial Intelligence: Founda-
tions, Theory, and Algorithms Feature Selection for
High-Dimensional Data. Springer.
Bolon-Canedo, V., Sanchez-Marono, N., and Alonso-
Betanzos, A. (2011). Feature selection and classifi-
cation in multiple class datasets: An application to
kdd cup 99 dataset. Expert Systems with Applications,
38(5):5947–5957.
Febrero, M., Galeano, P., and Gonz
´
alez-Manteiga, W.
(2008). Outlier detection in functional data by depth
measures, with application to identify abnormal nox
levels. Environmetrics: The official journal of the In-
ternational Environmetrics Society, 19(4):331–345.
Febrero-Bande, M., de la Fuente, M. O., et al. (2012). Sta-
tistical computing in functional data analysis: The
r package fda. usc. Journal of statistical Software,
51(4):1–28.
Ferraty, F. and Vieu, P. (2006). Nonparametric functional
data analysis: theory and practice. Springer Science
& Business Media.
Flores, M., Tarr
´
ıo-Saavedra, J., Fern
´
andez-Casal, R., and
Naya, S. (2018). Functional extensions of mandel’s
h and k statistics for outlier detection in interlabora-
tory studies. Chemometrics and Intelligent Labora-
tory Systems, 176:134–148.
Francisco-Fern
´
andez, M., Tarr
´
ıo-Saavedra, J., Mallik, A.,
and Naya, S. (2012). A comprehensive classifica-
tion of wood from thermogravimetric curves. Chemo-
metrics and Intelligent Laboratory Systems, 118:159–
172.
Kira, K. and Rendell, L. A. (1992). A practical approach to
feature selection. In Machine Learning Proceedings
1992, pages 249–256. Elsevier.
Lee, J., Kao, H.-A., and Yang, S. (2014). Service innova-
tion and smart analytics for industry 4.0 and big data
environment. Procedia Cirp, 16:3–8.
Liu, R. Y. (1995). Control charts for multivariate pro-
cesses. Journal of the American Statistical Associa-
tion, 90(432):1380–1387.
L
´
opez-Pintado, S. and Romo, J. (2009). On the concept
of depth for functional data. Journal of the American
Statistical Association, 104(486):718–734.
Mallik, A., Tarr
´
ıo-Saavedra, J., Francisco-Fern
´
andez, M.,
and Naya, S. (2011). Classification of wood micro-
graphs by image segmentation. Chemometrics and in-
telligent laboratory systems, 107(2):351–362.
Montgomery, D. C. (2007). Introduction to statistical qual-
ity control. John Wiley & Sons.
Naya, S. (2017). Industry 4.0. an opportunity for the re-
lationship between university and shipbuilding in the
future. In Pan-American Conference of Naval Engi-
neering, pages 169–177. Springer.
Papadimitriou, S., Kitagawa, H., Gibbons, P. B., and Falout-
sos, C. (2003). Loci: Fast outlier detection using the
local correlation integral. In Proceedings 19th Inter-
national Conference on Data Engineering (Cat. No.
03CH37405), pages 315–326. IEEE.
Tukey, J. W. (1977). Exploratory data analysis.
Woodall, W. H. (2007). Current research on profile moni-
toring. Production, 17(3):420–425.
Case Study of Anomaly Detection and Quality Control of Energy Efficiency and Hygrothermal Comfort in Buildings
151