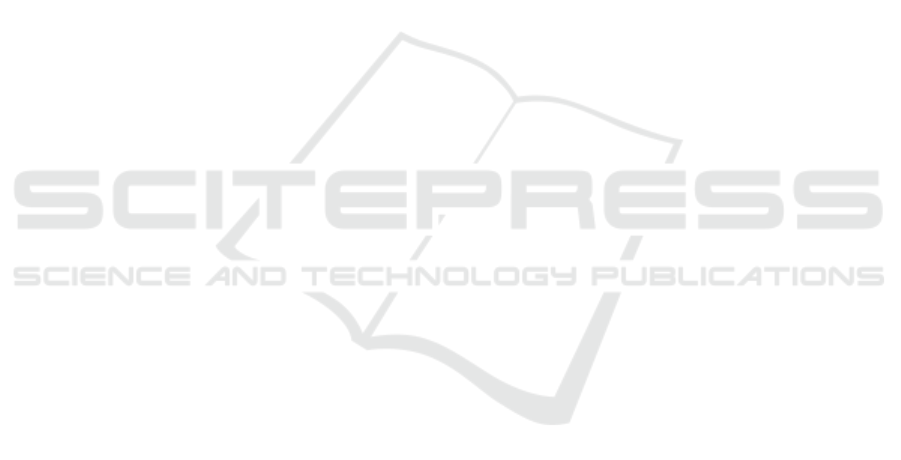
European Commission/EACEA/Eurydice (2015). The Eu-
ropean Higher Education Area in 2015: Bologna Pro-
cess Implementation Report. Technical report, Publi-
cations Office of the European Union, Luxembourg.
Friedman, J. H. (2001). Greedy function approximation: A
gradient boosting machine. The Annals of Statistics,
29(5):1189–1232.
Gaudioso, E., Montero, M., Talavera, L., and Hernandez-
del Olmo, F. (2009). Supporting teachers in collab-
orative student modeling: A framework and an im-
plementation. Expert Systems with Applications, 36(2
PART 1):2260–2265.
Gonz
´
alez-Marcos, A., Alba-El
´
ıas, F., Navaridas-Nalda, F.,
and Ordieres-Mer
´
e, J. (2016). Student evaluation of
a virtual experience for project management learning:
An empirical study for learning improvement. Com-
puters & Education, 102:172–187.
Gonz
´
alez-Marcos, A., Navaridas-Nalda, F., Ordieres-Mer
´
e,
J., and Alba-El
´
ıas, F. (2019). Handbook of Research
on E-Assessment in Higher Education, chapter A
Model for Competence E-Assessment and Feedback
in Higher Education, pages 295–311. IGI Global, Her-
shey PA, USA.
Gonz
´
alez-Marcos, A., Olarte-Valent
´
ın, R., Sainz-Garc
´
ıa,
E., and M
´
ugica-Vidal, R. (2017). A Virtual Learning
Environment to Support Project Management Teach-
ing. In Proceedings of the International Joint Confer-
ence SOCO’17-ICEUTE’17, pages 751–759.
Graf, S., Viola, S. R., and Kinshuk (2007). Automatic
student modelling for detecting learning style prefer-
ences in learning management systems. In Proceed-
ings of the IADIS international conference on cogni-
tion and exploratory learning in digital age (CELDA
2007), pages 172–179.
Hoffait, A. S. and Schyns, M. (2017). Early detection of
university students with potential difficulties. Deci-
sion Support Systems, 101:1–11.
IPMA (2006). ICB: IPMA Competence Baseline, version 3.
International Project Management Association.
Jiang, Y. H., Javaad, S. S., and Golab, L. (2016). Data min-
ing of undergraduate course evaluations. Informatics
in Education, 15(1):85–102.
Laugerman, M., Rover, D. T., Shelley, M. C., and Mick-
elson, S. K. (2015). Determining Graduation Rates
in Engineering for Community College Transfer Stu-
dents Using Data Mining. International Journal of
Engineering Education, 31(6(A)):1448–1457.
Li, N., Matsuda, N., Cohen, W., and Koedinger, K. (2011).
A Machine Learning Approach for Automatic Stu-
dent Model Discovery. In Proceedings of 4th Interna-
tional Conference on Educational Data Mining (EDM
2011), pages 31–40.
Macfadyen, L. P. and Dawson, S. (2010). Mining LMS
data to develop an ”early warning system” for educa-
tors: A proof of concept. Computers and Education,
54(2):588–599.
Marbouti, F., Diefes-Dux, H. A., and Madhavan, K. (2016).
Models for early prediction of at-risk students in a
course using standards-based grading. Computers and
Education, 103:1–15.
M
´
arquez-Vera, C., Cano, A., Romero, C., Noaman, A.
Y. M., Mousa Fardoun, H., and Ventura, S. (2016).
Early dropout prediction using data mining: A case
study with high school students. Expert Systems,
33(1):107–124.
Migu
´
eis, V. L., Freitas, A., Garcia, P. J., and Silva, A.
(2018). Early segmentation of students according to
their academic performance: A predictive modelling
approach. Decision Support Systems, 115:36–51.
Mishra, T., Kumar, D., and Gupta, S. (2014). Mining stu-
dents’ data for prediction performance. International
Conference on Advanced Computing and Commu-
nication Technologies, ACCT, (February 2014):255–
262.
Romero, C., Espejo, P. G., Zafra, A., Romero, J. R., and
Ventura, S. (2010). Web usage mining for predict-
ing final marks of students that use Moodle courses.
Computer Applications in Engineering Education,
21(1):135–146.
Romero, C. and Ventura, S. (2010). Educational data min-
ing: a review of the state of the art. IEEE Transactions
on Systems, Man, and Cybernetics, Part C: Applica-
tions and Reviews, 40(6):601–618.
Romero, C. and Ventura, S. (2013). Data mining in edu-
cation. Wiley Interdisciplinary Reviews: Data Mining
and Knowledge Discovery, 3(1):12–27.
Strecht, P., Cruz, L., Soares, C., Mendes-Moreira, J., and
Abreu, R. (2015). A Comparative Study of Classifi-
cation and Regression Algorithms for Modelling Stu-
dents’ Academic Performance. Proceedings of the 8th
International Conference on Educational Data Min-
ing, pages 392–395.
Vapnik, V. (2000). The Nature of Statistical Learning The-
ory. Springer-Verlag, New York, 2nd edition.
Predicting Students’ Performance in a Virtual Experience for Project Management Learning
673