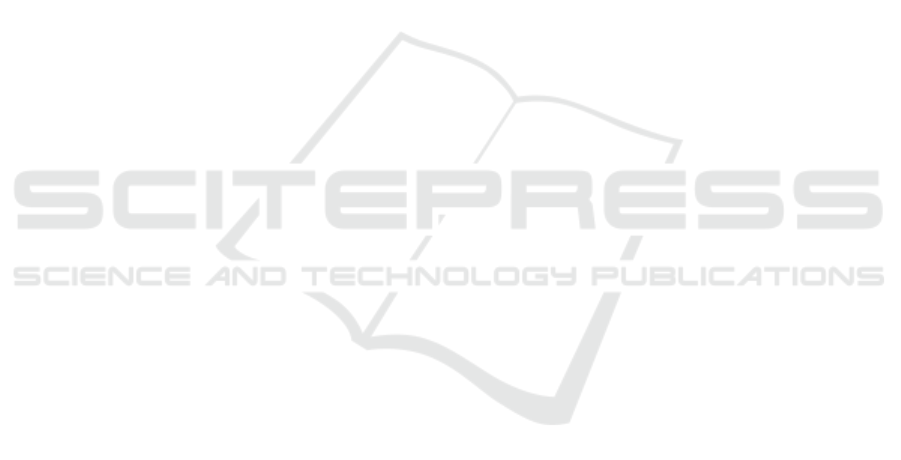
Informatics in Control Automation and Robotics, Lec-
ture Notes in Electrical Engineering. Springer. Under
review.
Karbalayghareh, A., Qian, X., and Dougherty, E. R. (2018).
Optimal Bayesian transfer learning. IEEE Transac-
tions on Signal Processing, 66(14):3724–3739.
K
´
arn
´
y, M., B
¨
ohm, J., Guy, T. V., Jirsa, L., Nagy, I., Ne-
doma, P., and Tesa
ˇ
r, L. (2005). Optimized Bayesian
Dynamic Advising: Theory and Algorithms. Springer,
London.
K
´
arn
´
y, M. and Kroupa, T. (2012). Axiomatisation of
fully probabilistic design. Information Sciences,
186(1):105–113.
Khaleghi, B., Khamis, A., Karray, F. O., and Razavi, S. N.
(2013). Multisensor data fusion: a review of the state-
of-the-art. Information Fusion, 14(1):28 – 44.
Lahat, D., Adali, T., and Jutten, C. (2015). Multimodal
data fusion: an overview of methods, challenges, and
prospects. Proceedings of the IEEE, 103(9):1449–
1477.
Lang, L., Chen, W., Bakshi, B. R., Goel, P. K., and Un-
garala, S. (2007). Bayesian estimation via sequential
Monte Carlo sampling — constrained dynamic sys-
tems. Automatica, 43(9):1615–1622.
Majumder, S. and Pratihar, D. K. (2018). Multi-sensors data
fusion through fuzzy clustering and predictive tools.
Expert Systems with Applications, 107:165 – 172.
Nassreddine, G., Abdallah, F., and Denoux, T. (2010). State
estimation using interval analysis and belief-function
theory: application to dynamic vehicle localization.
IEEE Transactions on Systems, Man, and Cybernet-
ics, Part B (Cybernetics), 40(5):1205–1218.
Pape
ˇ
z, M. and Quinn, A. (2018). Dynamic Bayesian knowl-
edge transfer between a pair of Kalman filters. In 2018
28th International Workshop on Machine Learning for
Signal Processing (MLSP), pages 1–6, Aalborg, Den-
mark. IEEE.
Pavelkov
´
a, L. and Jirsa, L. (2018). Approximate recur-
sive Bayesian estimation of state space model with
uniform noise. In Proceedings of the 15th Interna-
tional Conference on Informatics in Control, Automa-
tion and Robotics (ICINCO), pages 388–394, Porto,
Portugal.
Quinn, A., K
´
arn
´
y, M., and Guy, T. (2016). Fully probabilis-
tic design of hierarchical Bayesian models. Informa-
tion Sciences, 369(1):532–547.
Quinn, A., K
´
arn
´
y, M., and Guy, T. V. (2017). Opti-
mal design of priors constrained by external predic-
tors. International Journal of Approximate Reasoning,
84:150–158.
Shamshirband, S., Petkovic, D., Javidnia, H., and Gani, A.
(2015). Sensor data fusion by support vector regres-
sion methodology-a comparative study. IEEE Sensors
Journal, 15(2):850–854.
Simon, D. and Simon, D. L. (2010). Constrained Kalman
filtering via density function truncation for turbofan
engine health estimation. International Journal of
Systems Science, 41:159–171.
Torrey, L. and Shavlik, J. (2010). Transfer learning. In
Handbook of Research on Machine Learning Appli-
cations and Trends: Algorithms, Methods, and Tech-
niques, pages 242–264. IGI Global.
Vapnik, V. and Izmailov, R. (2017). Knowledge transfer
in SVM and neural networks. Annals of Mathematics
and Artificial Intelligence, 81(1-2):3–19.
Vargas-Melendez, L., Boada, B. L., Boada, M. J. L.,
Gauchia, A., and Diaz, V. (2017). Sensor fusion based
on an integrated neural network and probability den-
sity function (PDF) dual Kalman filter for on-line es-
timation of vehicle parameters and states. Sensors,
17(5).
Vicino, A. and Zappa, G. (1996). Sequential approximation
of feasible parameter sets for identification with set
membership uncertainty. IEEE Transactions on Auto-
matic Control, 41(6):774–785.
Vitola, J., Pozo, F., Tibaduiza, D. A., and Anaya, M. (2017).
A sensor data fusion system based on k-nearest neigh-
bor pattern classification for structural health monitor-
ing applications. Sensors, 17(2).
Willner, D., Chang, C., and Dunn, K. (1976). Kalman filter
algorithms for a multi-sensor system. In 1976 IEEE
Conference on Decision and Control including the
15th Symposium on Adaptive Processes, pages 570–
574.
Xiao, F. (2019). Multi-sensor data fusion based on the be-
lief divergence measure of evidences and the belief en-
tropy. Information Fusion, 46:23 – 32.
Yang, C., Yang, Z., and Deng, Z. (2019). Robust weighted
state fusion Kalman estimators for networked systems
with mixed uncertainties. Information Fusion, 45:246
– 265.
Zou, T., Wang, Y., Wang, M., and Lin, S. (2017).
A real-time smooth weighted data fusion algorithm
for greenhouse sensing based on wireless sensor net-
works. Sensors, 17(11).
ICINCO 2019 - 16th International Conference on Informatics in Control, Automation and Robotics
506