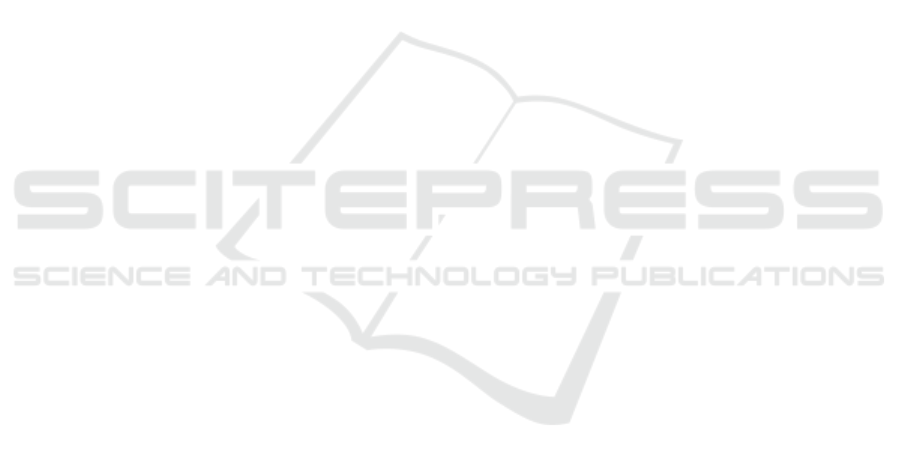
test (t-Test) revealed that the differences between
accuracies of the two considered synthesis methods
are significant.
The results obtained in this study lead to the
conclusion that the proposed model may retain
Lombard effect characteristics.
In the future, we would like to pursue the analysis
of the synthesized phonemes in the context of
checking whether the models created are language-
dependent.
Moreover, future research will expand the
database so that it can be possible to compare the
results obtained with the state-of-the-art algorithms,
such as neural networks (and specifically
convolutional neural networks). The authors have
experience in such an analysis (Korvel et al., 2018),
but even though it will not be possible to directly
compare the results, because, in the case of deep
learning, 2D signal representations will be used
(cepstrogram, spectrogram, etc.).
Additionally, in the case of speech synthesis, an
essential element is the subjective test that allows for
assessing the quality of the synthesized sounds
obtained. This aspect is especially interesting in the
context of language specifics. Preliminary, informal
tests show that quality of the synthesized phonemes
may be directly compared to the original sound.
Therefore, the subjective quality evaluation will be
based on formal listening test sessions in which
normal-hearing subjects will participate. The original
phoneme, as well as the corresponding synthesized
versions, will be used. Subjects will be asked to
answer the following question: “Does the phoneme
sound natural?” and to assign a corresponding score.
Then, the participants will have to distinguish
between the original phoneme and the synthesized
one in the AA-AB comparison test, where A is the
original sound and B the synthesized phoneme. Thus,
this will be thoroughly researched in the future.
ACKNOWLEDGMENTS
This research is funded by the European Social Fund
under the No 09.3.3-LMT-K-712 “Development of
Competences of Scientists, other Researchers and
Students through Practical Research Activities”
measure.
REFERENCES
Al-Ali, A. K. H., Dean, D., Senadji, B., Chandran, V., Naik,
G. R., 2017. Enhanced forensic speaker verification
using a combination of DWT and MFCC feature
warping in the presence of noise and reverberation
conditions, IEEE Access, 5, 15400-15413.
Boril, H., Hansen, J.H.L., 2010. Unsupervised Equalization
of Lombard Effect for Speech Recognition in Noisy
Adverse Environments, IEEE Transactions On Audio,
Speech, And Language Processing, 18(6), 1379-1393.
Boril, H., Pollák, P., 2005. Design and Collection of Czech
Lombard Speech Database, Ninth European
Conference on Speech Communication and
Technology.
Brumm, H., Zollinger, S. A., 2011. The evolution of the
Lombard effect: 100 years of psychoacoustic research.
Behaviour, 148(11-13), 1173-1198. DOI: 148. 1173-
1198. 10.2307/41445240.
Chai, T., Draxler, R. R., 2014. Root mean square error
(RMSE) or mean absolute error (MAE)? Arguments
against avoiding RMSE in the literature, Geoscientific
Model Development, 7, 1247–1250.
Ellis, D. P. W., 2004. Sinewave Speech Analysis/Synthesis
in Matlab, Web resource, available:
http://www.ee.columbia.edu/ln/labrosa/matlab/sws/
accessed February 2019).
Ellis, D. P., 2008. An introduction to signal processing for
speech, The Handbook of Phonetic Sciences, 755-780.
DOI:10.1002/9781444317251.ch20
Folk, L., Schiel, F., 2011. The Lombard Effect in
spontaneous dialog speech, Proceedings of the
Interspeech, 2701-2704.
Garnier, M., Bailly, L., Dohen, M., Welby, P.,
Loevenbruck, H., 2006. An acoustic and articulatory
study of Lombard speech: Global effects on the
utterance, Ninth International Conference on Spoken
Language Processing, INTERSPEECH 2006 – ICSLP,
2246-2249.
Garnier, M., Henrich, N., 2013. Speaking in noise: How
does the Lombard effect improve acoustic contrasts
between speech and ambient noise?, Computer Speech
& Language, 28(2), 580-597.
Godoy, E., Koutsogiannaki, M., Stylianou, Y., 2014,
Approaching speech intelligibility enhancement with
inspiration from Lombard and Clear speaking styles,
Computer Speech & Language 28(2), 629-647.
Godoy, E., Koutsogiannaki, M., Stylianou, Y., 2014.
Approaching speech intelligibility enhancement with
inspiration from Lombard and Clear speaking styles,
Computer Speech and Language, 28(2), 629-647.
Huang, D. Y., Rahardja, S., Ong, E. P., 2010. Lombard
effect mimicking. In Seventh ISCA Workshop on
Speech Synthesis.
Kim, J., Davis, Ch., 2014. Comparing the consistency and
distinctiveness of speech produced in quiet and in noise.
Computer Speech & Language, 28(2), 598-606.
Kleczkowski, P., Żak, A., Król-Nowak, A., 2017. Lombard
Effect in Polish Speech and its Comparison in English
Speech, Archives of Acoustics, 42(4), 561–569,
doi:10.1515/aoa-2017-0060.
Korvel, G., Šimonytė, V., Slivinskas, V., 2016. A phoneme
harmonic generator, Information Technology and
Control, 45 (1), 7-12.
SIGMAP 2019 - 16th International Conference on Signal Processing and Multimedia Applications
288