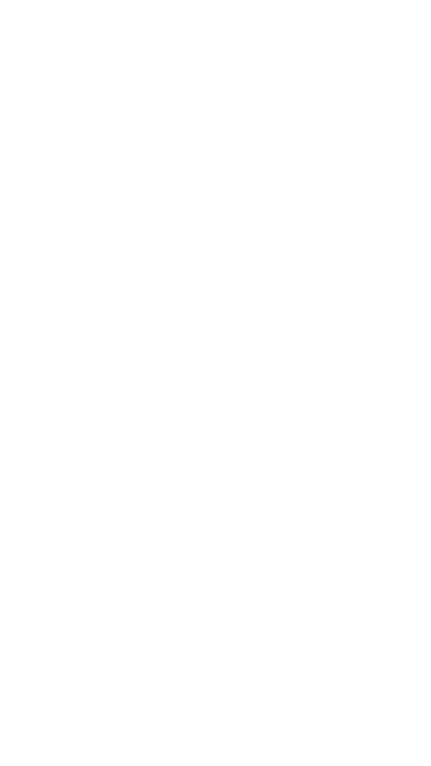
5 CONCLUSIONS
As a result of the analysis, the following
recommendations were formulated for reducing the
number of TA on the roads of Yelabuga:
1. Organization of events to reduce traffic load
and curb traffic violations at the entrances to the city
on Saturday from 17.00 to 18.00 and Sunday from
12.00 to 13.00 by summer drivers and tourist drivers
2. Development and implementation of
propaganda and educational activities to improve the
driving culture of men with driving experience up to
20 years.
3. Development and implementation of activities
for training and consolidating driving skills among
women with driving experience up to 5 years.
4. Organization of events to reduce the number of
drivers who are driving in a state of alcohol and drug
intoxication.
According to the prognostic model built on the
basis of growing trees, an accident class with the
number of injured 2 or more people has been
allocated. The following decision rules were obtained
for him: Type of accident - collisions resulting from
violations of the rules of the vehicle’s location on the
carriageway and non-observance of the travel order;
place of the accident - unregulated intersections of
unequal streets (roads); Month - March, April,
October; The time of the accident - 1.00, 5.00, 21.00.
It is necessary to analyze the impact of the
measures taken for the reconstruction of road
infrastructure, preventive or regulatory measures, and
the modernization of vehicle design on road safety.
At the same time, the evaluation of the effectiveness
of decisions should be made on the basis of feedback.
For this, it is necessary to select among the whole set
of factors those that most strongly influence the
severity of accidents, and then re-calculate
quantitative criteria for assessing the severity of
accidents in the next period. In this case, the adoption
and evaluation of the effectiveness of measures
affecting the selected factors will reduce human
losses.
ACKNOWLEDGEMENTS
Research is partially funded by national grant No.
BR05236644.
REFERENCES
Hu, S., Ivan, J. N., Raishanker, N., Mooradian, J., 2013.
Temporal modeling of highway crash counts for senior
and non-senior drivers. Accid. Anal. Prev. 50, 1003–
1013.
Huang, H., Chin, H. C., Haque, M., 2009. Empirical
evaluation of alternative approaches in identifying
crash hot spots: naive ranking, empirical bayes, and full
bayes methods. Transp. Res. Rec. 2103, 32–41.
Jiang, X., Abdel-Aty, M., Alamili, S., 2014. Application of
Poisson random effect models for highway network
screening. Accid. Anal. Prev. 63, 74–82.
On approval of the Road Safety Strategy, 2019. Retrieved
January 22, 2019, from hxxp://government.ru/docs/
31102/.
Park, P. Y., Sahaji, R., 2013. Safety network screening for
municipalities with incomplete traffic volume data.
Accid. Anal. Prev. 50, 1062–1072.
Road Safety Indicators, 2019. Official website of road
police. Retrieved January 22, 2019, from hxxp://stat.
gibdd.ru.
Serhiyenko, V., Mamun, S. A., Ivan, J. N., Ravishanker, N.,
2016. Fast Bayesian inference for modeling multivariate
crash counts. Anal. Methods Accid. Res. 9, 44–53.
Srinivasan, R., Bauer, K., 2013. Safety Performance
Function Development Guide: Developing Jurisdiction-
Specific SPFs, Final Report. Report No. FSWA-SA-14-
005. Federal Highway Administration September.
Stipancic J., Miranda-Moreno L., SaunierТ.,Labbe А.,
2018. Surrogate safety and network screening:
Modelling crash frequency using GPS travel data and
latent Gaussian Spatial Models. Accid. Anal. Prev. 120,
174–187.
Yannis, G., Dragomanovits, A., Laiou, A., Richter, T.,
Ruhl, S., La Torre, F., Domenichini, L., Graham, D.,
Karathodorou, N., Li, H., 2016. Use of Prediction
model in road safety management – an international
inquiry. Transportation Research Proc. 14, 4257–4266.
Yannis, G., Dragomanovits, A., Laiou, A., La Torre, F.,
Domenichini, L., Richter, T., Ruhl, S., Graham, D.,
Karathodorou, N., 2017. Road traffic accident prediction
modelling: a literature review. In: Proceedings of the
Institution of Civil Engineers – Transport, 170 (5), 245–
254.
Improving Road Safety by Affecting Negative Factors
637