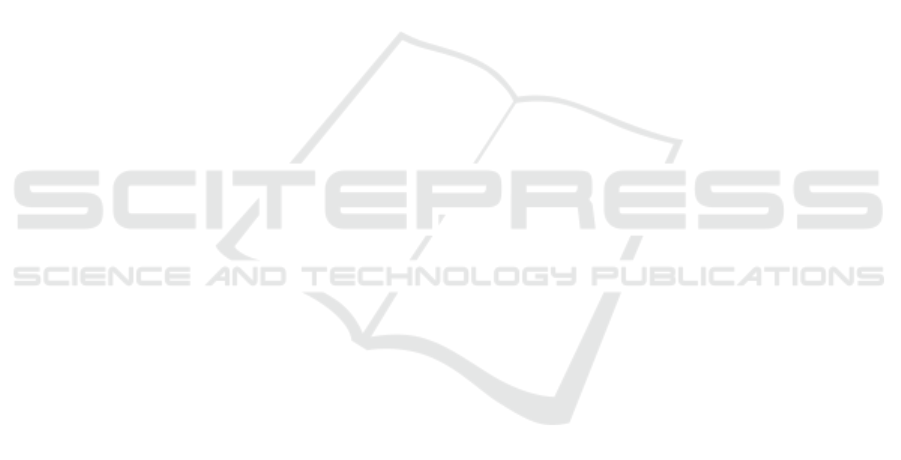
aspects of the problems mentioned in the paper (e.g.,
features, models, metrics, dataset definition, etc.), as
well as scientific works that tackle specific tasks in-
cluding the challenges that have been briefly pre-
sented in the proposed paper (e.g., popularity, relative
attributes, virality, etc.). Furthermore, proper cases of
study aimed to highlight the concepts described in the
paper will be prepared and evaluated, with the aim to
support the key aspects of each addressed issue.
REFERENCES
Alameda-Pineda, X., Pilzer, A., Xu, D., Sebe, N., and Ricci,
E. (2017). Viraliency: Pooling local virality. In 2017
IEEE Conference on Computer Vision and Pattern
Recognition (CVPR), pages 484–492.
Altwaijry, H. and Belongie, S. (2013). Relative ranking of
facial attractiveness. In IEEE Workshop on Applica-
tions of Computer Vision (WACV), pages 117–124.
Battiato, S., Farinella, G. M., Milotta, F. L., Ortis, A., Ad-
desso, L., Casella, A., D’Amico, V., and Torrisi, G.
(2016). The social picture. In Proceedings of the 2016
ACM on International Conference on Multimedia Re-
trieval, pages 397–400. ACM.
Borth, D., Ji, R., Chen, T., Breuel, T., and Chang, S.-F.
(2013). Large-scale visual sentiment ontology and de-
tectors using adjective noun pairs. In Proceedings of
the 21st ACM international conference on Multime-
dia, pages 223–232. ACM.
Bradley, M. M. (1994). Emotional memory: A dimensional
analysis. Emotions: Essays on emotion theory, pages
97–134.
Campos, V., Jou, B., and i Nieto, X. G. (2017). From pix-
els to sentiment: Fine-tuning cnns for visual senti-
ment prediction. Image and Vision Computing, 65:15
– 22. Multimodal Sentiment Analysis and Mining in
the Wild Image and Vision Computing.
Campos, V., Salvador, A., Gir
´
o-i Nieto, X., and Jou, B.
(2015). Diving deep into sentiment: Understanding
fine-tuned cnns for visual sentiment prediction. In
Proceedings of the 1st International Workshop on Af-
fect & Sentiment in Multimedia, ASM ’15, pages
57–62, New York, NY, USA. ACM.
Cappallo, S., Mensink, T., and Snoek, C. G. (2015a). Im-
age2emoji: Zero-shot emoji prediction for visual me-
dia. In Proceedings of the 23rd ACM international
conference on Multimedia, pages 1311–1314. ACM.
Cappallo, S., Mensink, T., and Snoek, C. G. (2015b). Latent
factors of visual popularity prediction. In Proceedings
of the 5th ACM on International Conference on Mul-
timedia Retrieval, pages 195–202. ACM.
Cappallo, S., Svetlichnaya, S., Garrigues, P., Mensink, T.,
and Snoek, C. G. M. (2018). The new modality: Emoji
challenges in prediction, anticipation, and retrieval.
IEEE Transactions on Multimedia. Pending minor re-
vision.
Chen, T., Borth, D., Darrell, T., and Chang, S.-F. (2014).
Deepsentibank: Visual sentiment concept classifica-
tion with deep convolutional neural networks. arXiv
preprint arXiv:1410.8586.
Colombo, C., Del Bimbo, A., and Pala, P. (1999). Seman-
tics in visual information retrieval. IEEE Multimedia,
6(3):38–53.
Dan-Glauser, E. S. and Scherer, K. R. (2011). The geneva
affective picture database (gaped): a new 730-picture
database focusing on valence and normative signifi-
cance. Behavior research methods, 43(2):468–477.
Datta, R., Joshi, D., Li, J., and Wang, J. Z. (2006). Study-
ing aesthetics in photographic images using a compu-
tational approach. In European Conference on Com-
puter Vision, pages 288–301. Springer.
Deza, A. and Parikh, D. (2015). Understanding image vi-
rality. In 2015 IEEE Conference on Computer Vision
and Pattern Recognition (CVPR), pages 1818–1826.
Ekman, P., Friesen, W. V., O’Sullivan, M., Chan, A.,
Diacoyanni-Tarlatzis, I., Heider, K., Krause, R.,
LeCompte, W. A., Pitcairn, T., Ricci-Bitti, P., Scherer,
K., Tomita, M., and Tzavaras, A. (1987). Universals
and cultural differences in the judgments of facial ex-
pressions of emotion. Journal of personality and so-
cial psychology, 53(4):712.
Esuli, A. and Sebastiani, F. (2006). Sentiwordnet: A pub-
licly available lexical resource for opinion mining.
In Proceedings of LREC, volume 6, pages 417–422.
Citeseer.
Fan, Q., Gabbur, P., and Pankanti, S. (2013). Relative at-
tributes for large-scale abandoned object detection. In
Proceedings of the IEEE International Conference on
Computer Vision, pages 2736–2743.
Furnari, A., Battiato, S., and Farinella, G. M. (2018).
Personal-location-based temporal segmentation of
egocentric video for lifelogging applications. Journal
of Visual Communication and Image Representation,
52:1–12.
Hayashi, T. and Hagiwara, M. (1998). Image query by im-
pression words-the iqi system. IEEE Transactions on
Consumer Electronics, 44(2):347–352.
Hogenboom, A., Bal, D., Frasincar, F., Bal, M., de Jong,
F., and Kaymak, U. (2013). Exploiting emoticons in
sentiment analysis. In Proceedings of the 28th Annual
ACM Symposium on Applied Computing, pages 703–
710. ACM.
Huiskes, M. J., Thomee, B., and Lew, M. S. (2010). New
trends and ideas in visual concept detection: the mir
flickr retrieval evaluation initiative. In Proceedings of
the international conference on Multimedia informa-
tion retrieval, pages 527–536. ACM.
Itten, J. (1973). The Art of Color: The Subjective Experi-
ence and Objective Rationale of Color. John Wiley &
Sons Inc.
Jufeng Yang, Dongyu She, M. S. (2017). Joint image emo-
tion classification and distribution learning via deep
convolutional neural network. In Proceedings of the
Twenty-Sixth International Joint Conference on Artifi-
cial Intelligence, IJCAI-17, pages 3266–3272.
SIGMAP 2019 - 16th International Conference on Signal Processing and Multimedia Applications
298