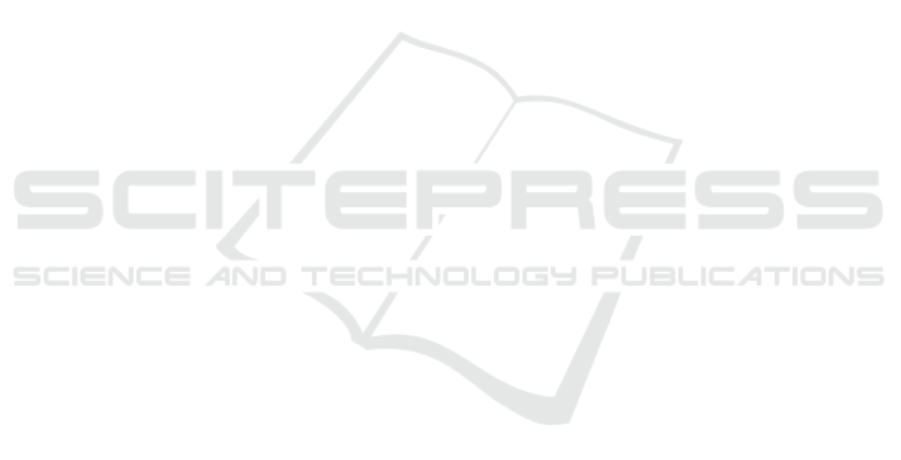
enabled us to calculate correlations between the es-
timations and the in-field measurements of the crop
variables. Since the vegetation indices tend to be
evolve linearly during the crop growth, we achieved
accurate correlations using multivariable regressions;
on average, correlations of 80% for biomass and 78%
for nitrogen were achieved. Upcoming work is ori-
ented towards improving the correlations by including
more sophisticated image classification and clustering
algorithms to consider several feature spaces for the
NIR pixels. By now, our system is not reliable during
the reproductive stage of the crop due to the mixed
plant color in between yellow and green. Also, differ-
ent genotypes of rice varieties are planted in the same
plot area. In this sense, we also expect to improve on
the estimation, since the biomass and nitrogen read-
ings are highly dependent of the plant variety.
ACKNOWLEDGEMENTS
This work was funded in part by the OMICAS pro-
gram: Optimizaci
´
on Multiescala In-silico de Cul-
tivos Agr
´
ıcolas Sostenibles (Infraestructura y vali-
daci
´
on en Arroz y Ca
˜
na de Az
´
ucar), sponsored within
the Colombian Scientific Ecosystem by The WORLD
BANK, COLCIENCIAS, ICETEX, the Colombian
Ministry of Education and the Colombian Ministry
of Industry and Turism under GRANT ID: FP44842-
217-2018. Also, by the research project entitled De-
sarrollo de una herramienta para la agricultura de
precision en los cultivos de arroz: sensado del es-
tado de crecimiento y de nutricion de las plantas us-
ando un drone autonomo, under the COLCIENCIAS
- GRANT ID 120371551916, CT167-2016 (FONDO
NACIONAL DE FINANCIAMIENTO PARA LA
CIENCIA, LA TECNOLOGIA Y LA INNOVACION
-FRANCISCO JOSE DE CALDAS).
REFERENCES
Arroyo, J. A., Gomez-Castaneda, C., Ruiz, E., de Cote,
E. M., Gavi, F., and Sucar, L. E. (2017). Uav technol-
ogy and machine learning techniques applied to the
yield improvement in precision agriculture. In 2017
IEEE Mexican Humanitarian Technology Conference
(MHTC), pages 137–143.
Carrijo, G. L. A., Oliveira, D. E., de Assis, G. A., Carneiro,
M. G., Guizilini, V. C., and Souza, J. R. (2017). Au-
tomatic detection of fruits in coffee crops from aerial
images. In 2017 Latin American Robotics Symposium
(LARS) and 2017 Brazilian Symposium on Robotics
(SBR), pages 1–6.
Devia, C. A., Rojas, J. P., Petro, E., Martinez, C., Mon-
dragon, I. F., Patino, D., Rebolledo, M. C., and Col-
orado, J. (2019). High-throughput biomass estimation
in rice crops using uav multispectral imagery. Journal
of Intelligent & Robotic Systems.
Dongyan Zhang, Xingen Zhou, Jian Zhang, Linsheng
Huang, J. and Zhao (2017). Developing a small uav
platform to detect sheath blight of rice. IGRASS, pages
7–10.
Gago, J., Douthe, C., Coopman, R., Gallego, P., Ribas-
Carbo, M., Flexas, J., Escalona, J., and Medrano, H.
(2015). Uavs challenge to assess water stress for sus-
tainable agriculture. Agricultural Water Management,
153:9 – 19.
Gevaert, C. M., Suomalainen, J., Tang, J., and Kooistra,
L. (2015). Generation of spectral 2013;temporal re-
sponse surfaces by combining multispectral satellite
and hyperspectral uav imagery for precision agricul-
ture applications. IEEE Journal of Selected Topics
in Applied Earth Observations and Remote Sensing,
8(6):3140–3146.
Gnyp, M. L., Miao, Y., Yuan, F., Ustin, S. L., Yu, K.,
Yao, Y., Huang, S., and Bareth, G. (2014). Hyper-
spectral canopy sensing of paddy rice aboveground
biomass at different growth stages. Field Crops Re-
search, 155:42–55.
Guo, T., Kujirai, T., and Watanabe, T. (2012). Map-
ping Crop Status From an Unmanned Aerial Vehicle
for Precision Agriculture Applications. ISPRS - In-
ternational Archives of the Photogrammetry, Remote
Sensing and Spatial Information Sciences, XXXIX-
B1(September):485–490.
Hongli, L., Zhoumiqi, Y., Jinshui, Z., and Shuai, G.
(2017). Highly efficient paddy classification using
uav-based orthorectified image. In 2017 IEEE Inter-
national Geoscience and Remote Sensing Symposium
(IGARSS), pages 3230–3233.
Jose, N., Ivan, M., Diego, P., and Julian, C. (2016). Mul-
tispectral mapping in agriculture: Terrain mosaic us-
ing an autonomous quadcopter UAV. In 2016 Inter-
national Conference on Unmanned Aircraft Systems,
ICUAS 2016, pages 1351–1358.
Khanna, R., Martin, M., Pfeifer, J., Liebisch, F., Walter,
A., and Siegwart, R. (2015). Beyond Point Clouds -
3D Mapping and Field Parameter Measurements us-
ing UAVs. IEEE 20th Conference on Emerging Tech-
nologies & Factory Automation, pages 5–8.
Lu, J., Miao, Y., Huang, Y., Shi, W., Hu, X., Wang, X.,
and Wan, J. (2015). Evaluating an unmanned aerial
vehicle-based remote sensing system for estimation of
rice nitrogen status. 2015 4th International Confer-
ence on Agro-Geoinformatics, Agro-Geoinformatics
2015, pages 198–203.
Naito, H., Ogawa, S., Valencia, M. O., Mohri, H., Urano,
Y., Hosoi, F., Shimizu, Y., Chavez, A. L., Ishitani,
M., Selvaraj, M. G., and Omasa, K. (2017). Esti-
mating rice yield related traits and quantitative trait
loci analysis under different nitrogen treatments using
a simple tower-based field phenotyping system with
modified single-lens reflex cameras. ISPRS Journal
of Photogrammetry and Remote Sensing, 125(Supple-
ment C):50 – 62.
Rojas, J., Carlos, D., Petro, E., Martinez, C., Mondragon,
I. F., Patino, D., Rebolledo, M., and Colorado, J.
ICINCO 2019 - 16th International Conference on Informatics in Control, Automation and Robotics
102