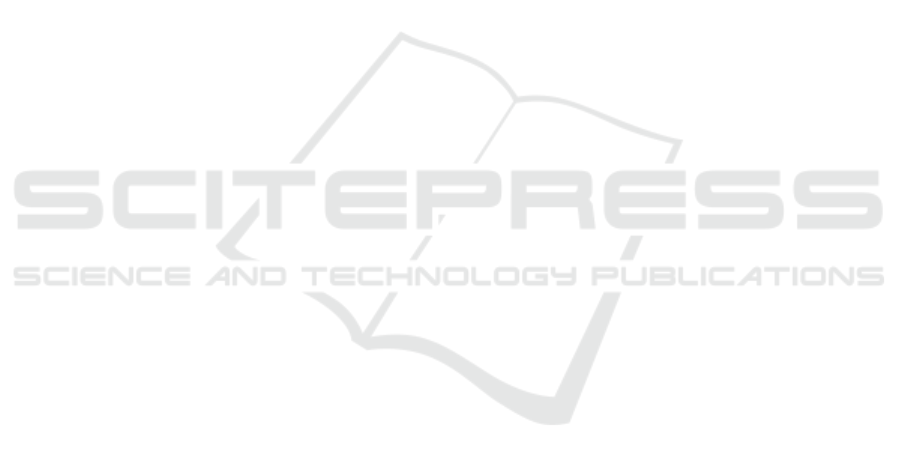
Hermans, A., Beyer, L., and Leibe, B. (2017). In defense
of the triplet loss for person re-identification. CoRR,
abs/1703.07737.
Hoffer, E. and Ailon, N. (2015). Deep metric learning
using triplet network. In International Workshop on
Similarity-Based Pattern Recognition, pages 84–92.
Springer.
Hoque, E. and Stankovic, J. (2012). Aalo: Activity recog-
nition in smart homes using active learning in the
presence of overlapped activities. In 2012 6th Inter-
national Conference on Pervasive Computing Tech-
nologies for Healthcare (PervasiveHealth) and Work-
shops, pages 139–146.
Hu, J., Lu, J., and Tan, Y. (2014). Discriminative deep
metric learning for face verification in the wild. In
2014 IEEE Conference on Computer Vision and Pat-
tern Recognition, pages 1875–1882.
Huang, C., Zhu, S., and Yu, K. (2012). Large scale
strongly supervised ensemble metric learning, with
applications to face verification and retrieval. CoRR,
abs/1212.6094.
Kedem, D., Tyree, S., Sha, F., Lanckriet, G. R., and Wein-
berger, K. Q. (2012). Non-linear metric learning. In
Advances in Neural Information Processing Systems,
pages 2573–2581.
Li, F., Shirahama, K., Nisar, M. A., K
¨
oping, L., and Grze-
gorzek, M. (2018). Comparison of feature learning
methods for human activity recognition using wear-
able sensors. Sensors, 18(2).
Liu, X., Liu, L., Simske, S. J., and Liu, J. (2016). Human
daily activity recognition for healthcare using wear-
able and visual sensing data. In 2016 IEEE Interna-
tional Conference on Healthcare Informatics (ICHI),
pages 24–31.
Mahalanobis, P. C. (1936). On the generalised distance in
statistics. In Proceedings National Institute of Sci-
ence, India, volume 2, pages 49–55.
Margarito, J., Helaoui, R., Bianchi, A. M., Sartor, F.,
and Bonomi, A. G. (2016). User-independent
recognition of sports activities from a single wrist-
worn accelerometer: A template-matching-based ap-
proach. IEEE Transactions on Biomedical Engineer-
ing, 63(4):788–796.
Mehr, H. D., Polat, H., and Cetin, A. (2016). Resident ac-
tivity recognition in smart homes by using artificial
neural networks. In 2016 4th International Istanbul
Smart Grid Congress and Fair (ICSG), pages 1–5.
Paszke, A., Gross, S., Chintala, S., Chanan, G., Yang, E.,
DeVito, Z., Lin, Z., Desmaison, A., Antiga, L., and
Lerer, A. (2017). Automatic differentiation in pytorch.
In NIPS-W.
Reyes-Ortiz, J.-L., Oneto, L., Sam
`
a, A., Parra, X., and
Anguita, D. (2016). Transition-aware human activ-
ity recognition using smartphones. Neurocomput.,
171(C):754–767.
Sakoe, H. and Chiba, S. (1978). Dynamic programming
algorithm optimization for spoken word recognition.
IEEE Transactions on Acoustics, Speech, and Signal
Processing, 26(1):43–49.
Schroff, F., Kalenichenko, D., and Philbin, J. (2015).
Facenet: A unified embedding for face recognition
and clustering. CoRR, abs/1503.03832.
Sempena, S., Maulidevi, N. U., and Aryan, P. R. (2011).
Human action recognition using dynamic time warp-
ing. In Proceedings of the 2011 International Con-
ference on Electrical Engineering and Informatics,
pages 1–5.
Seto, S., Zhang, W., and Zhou, Y. (2015). Multivariate
time series classification using dynamic time warping
template selection for human activity recognition. In
2015 IEEE Symposium Series on Computational In-
telligence, pages 1399–1406.
Singhal, S. and Dubey, R. K. (2015). Automatic speech
recognition for connected words using dtw/hmm for
english/ hindi languages. In 2015 Communication,
Control and Intelligent Systems (CCIS), pages 199–
203.
Stisen, A., Blunck, H., Bhattacharya, S., Prentow, T. S.,
Kjærgaard, M. B., Dey, A., Sonne, T., and Jensen,
M. M. (2015). Smart devices are different: Assess-
ing and mitigatingmobile sensing heterogeneities for
activity recognition. In Proceedings of the 13th ACM
Conference on Embedded Networked Sensor Systems,
SenSys ’15, pages 127–140, New York, NY, USA.
ACM.
Weinberger, K. Q. and Saul, L. K. (2009). Distance met-
ric learning for large margin nearest neighbor clas-
sification. Journal of Machine Learning Research,
10(Feb):207–244.
Xing, E. P., Ng, A. Y., Jordan, M. I., and Russell, S. (2002).
Distance metric learning, with application to clus-
tering with side-information. In Proceedings of the
15th International Conference on Neural Information
Processing Systems, NIPS’02, pages 521–528, Cam-
bridge, MA, USA. MIT Press.
Zeng, M., Nguyen, L. T., Yu, B., Mengshoel, O. J., Zhu,
J., Wu, P., and Zhang, J. (2014). Convolutional neural
networks for human activity recognition using mobile
sensors. In 6th International Conference on Mobile
Computing, Applications and Services, pages 197–
205.
Robust Human Activity Recognition based on Deep Metric Learning
663