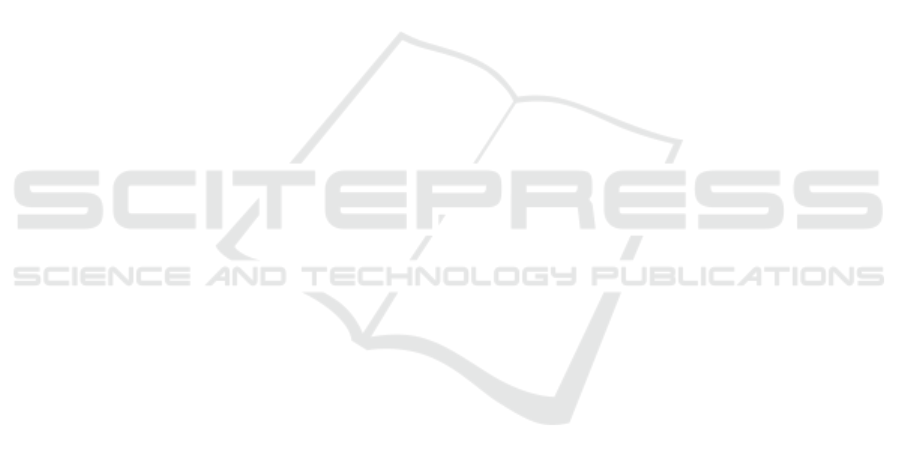
method consists of two main rules. Firstly, an Eu-
clidean distance was proposed to measure the distance
between the bodies of the evidences; then, the credi-
bility degree of the evidences is calculated. Secondly,
a weighted evidence value is given to all the sensors.
By assigning the evidence weight value to a small
number to a sensor deemed less reliable (the sensor
with lowest credibility), highest detection accuracy is
achieved. Modified evidences are fused by applying
the Dempster’s combination rule. A detailed example
for weed detection from an autonomous robot with
conflicting sensor input is presented which showcases
all the steps of the proposed method. A numerical
simulation is used to show that the proposed method
is comparably effective while offering a more compu-
tationally feasible algorithm than other related meth-
ods to handle the conflicting evidence combination
problem under multi-sensor environment
REFERENCES
Basti
`
ere, A. (1998). Methods for multisensor classifica-
tion of airborne targets integrating evidence theory.
Aerospace Science and Technology, 2(6):401–411.
Beynon, M., Curry, B., and Morgan, P. (2000). The
dempster–shafer theory of evidence: an alternative ap-
proach to multicriteria decision modelling. Omega,
28(1):37–50.
Chen, J., Ye, F., and Jiang, T. (2017). Numerical analyses
of modified ds combination methods based on differ-
ent distance functions. In 2017 Progress in Electro-
magnetics Research Symposium-Fall (PIERS-FALL),
pages 2169–2175. IEEE.
Chen, L. and Rao, S. (1998). A modified dempster-shafer
theory for multicriteria optimization. Engineering op-
timization, 30(3-4):177–201.
Chen, S., Deng, Y., and Wu, J. (2013). Fuzzy sensor fusion
based on evidence theory and its application. Applied
Artificial Intelligence, 27(3):235–248.
Dempster, A. P. (2008). Upper and lower probabilities in-
duced by a multivalued mapping. In Classic Works
of the Dempster-Shafer Theory of Belief Functions,
pages 57–72. Springer.
Deng, X. and Jiang, W. (2018). An evidential axiomatic
design approach for decision making using the evalu-
ation of belief structure satisfaction to uncertain target
values. International Journal of Intelligent Systems,
33(1):15–32.
Deng, Y. (2015). Deng entropy: a generalized shannon en-
tropy to measure uncertainty. viXra 1502.0222.
Denoeux, T. (1995). A k-nearest neighbor classification rule
based on dempster-shafer theory. IEEE transactions
on systems, man, and cybernetics, 25(5):804–813.
Denoeux, T. (2000). A neural network classifier based on
dempster-shafer theory. IEEE Transactions on Sys-
tems, Man, and Cybernetics-Part A: Systems and Hu-
mans, 30(2):131–150.
Hafeez, M. (2011). Application of dempster shafer theory
to assess the status of sealed fire in a cole mine.
Inagaki, T. (1991). Interdependence between safety-control
policy and multiple-sensor schemes via dempster-
shafer theory. IEEE Transactions on Reliability,
40(2):182–188.
Jiang, W., Wei, B., Xie, C., and Zhou, D. (2016a).
An evidential sensor fusion method in fault di-
agnosis. Advances in Mechanical Engineering,
8(3):1687814016641820.
Jiang, W., Zhuang, M., Qin, X., and Tang, Y. (2016b). Con-
flicting evidence combination based on uncertainty
measure and distance of evidence. SpringerPlus,
5(1):1217.
Kang, B., Chhipi-Shrestha, G., Deng, Y., Mori, J., Hewage,
K., and Sadiq, R. (2018). Development of a pre-
dictive model for clostridium difficile infection inci-
dence in hospitals using gaussian mixture model and
dempster–shafer theory. Stochastic Environmental Re-
search and Risk Assessment, 32(6):1743–1758.
Li, B., Wang, B., Wei, J., Huang, Y., and Guo, Z. (2001).
Efficient combination rule of evidence theory. In Ob-
ject Detection, Classification, and Tracking Technolo-
gies, volume 4554, pages 237–241. International So-
ciety for Optics and Photonics.
Lin, Y., Wang, C., Ma, C., Dou, Z., and Ma, X. (2016).
A new combination method for multisensor con-
flict information. The Journal of Supercomputing,
72(7):2874–2890.
Liu, T., Deng, Y., and Chan, F. (2018). Evidential supplier
selection based on dematel and game theory. Interna-
tional Journal of Fuzzy Systems, 20(4):1321–1333.
Luo, W. (1993). Imprecise probability and decision in
civil engineering: Dempster-Shafer theory and appli-
cation. PhD thesis, University of British Columbia.
Ma, J., Liu, W., Miller, P., and Zhou, H. (2016). An eviden-
tial fusion approach for gender profiling. Information
Sciences, 333:10–20.
Murphy, R. R. (1998). Dempster-shafer theory for sensor
fusion in autonomous mobile robots. IEEE Transac-
tions on Robotics and Automation, 14(2):197–206.
Sadiq, R. and Rodriguez, M. J. (2005). Interpreting drink-
ing water quality in the distribution system using
dempster–shafer theory of evidence. Chemosphere,
59(2):177–188.
Shafer, G. (1976). A mathematical theory of evidence, vol-
ume 42. Princeton university press.
Smets, P. (2000). Data fusion in the transferable belief
model. In Proceedings of the third international con-
ference on information fusion, volume 1, pages PS21–
PS33. IEEE.
Sun, S., Gao, J., Chen, M., Xu, B., and Ding, Z. (2013).
Fs-ds based multi-sensor data fusion. Journal of Soft-
ware, 8(5):1157–1162.
Xiao, F. (2017). A novel evidence theory and fuzzy pref-
erence approach-based multi-sensor data fusion tech-
nique for fault diagnosis. Sensors, 17(11):2504.
Xiao, F. (2018). An improved method for combining con-
flicting evidences based on the similarity measure
ICINCO 2019 - 16th International Conference on Informatics in Control, Automation and Robotics
670